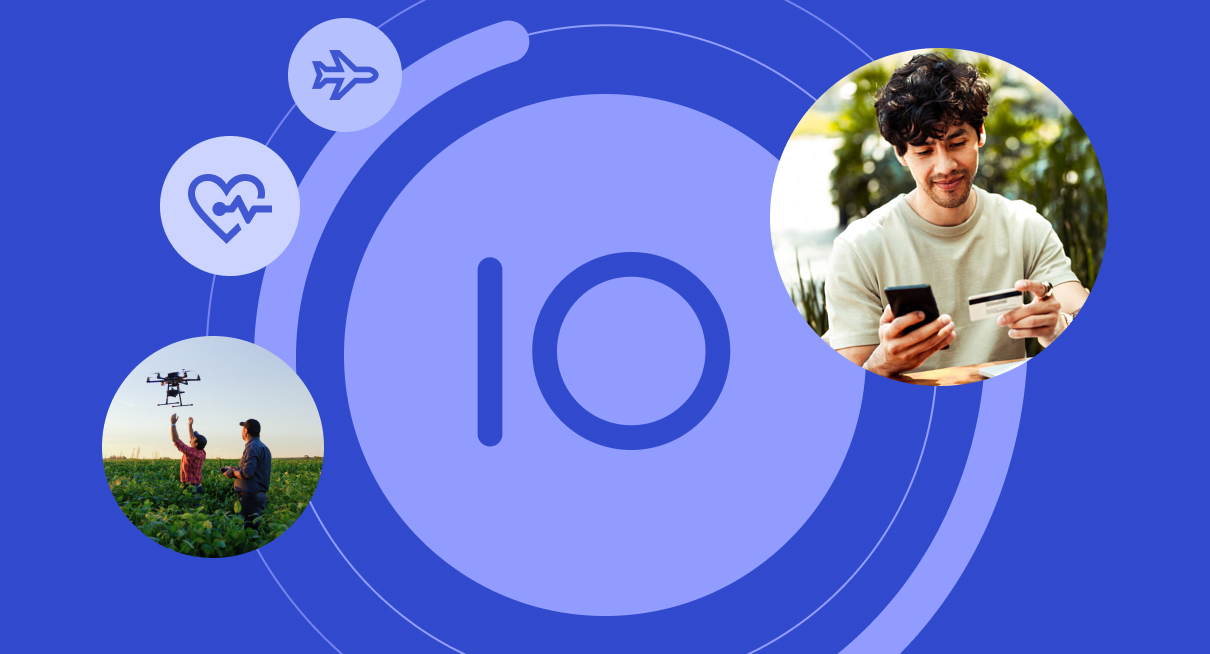
From demand forecasting to online medical diagnosis, artificial intelligence is totally changing the way we do business, live, and travel. In this article, we collected statistics, trends, and facts for those who want to learn more about machine learning and artificial intelligence role in business.
How Is Machine Learning Used in Industry?
According to PwC estimates, AI in different fields is expected to add $15.7 trillion to the global economy, which is more than the current economies of China and India make. Most of the gains will be provided by AI-based product enhancements that boost consumer demand. It becomes possible due to better personalization and affordability of products and services backed up with artificial intelligence. McKinsey’s research says that by 2030 AI will increase the global GDP by about 1.2% annually.
We picked 10 fields that use artificial intelligence where businesses are using AI for unprecedented automation, personalization, and reliable predictions.
No 1. AI in banking and financial services
Known as slow to adopt new technologies, traditional brick-and-mortar banks are now facing the challenge of the competition with tech-driven, super flexible, and digital-only fintechs. However, some of them are building successful digital transformation strategies that provide better online user experience, implement data analytics and automate services to provide more competitive pricing. Previously, we shared how AI in banking building the future and listed necessary steps banks need to take. AI in banking is expanding: it may encompass most of the operations from customer servicing, to risk assessment and marketing of financial services. How is machine learning used in industry of financial services? Several trends are highly likely to dominate AI and ML in the financial industry, here are a couple of those requiring your attention:
- The growing role of data analytics – researchers inform that by 2025 the amount of available data will exceed 180 zettabytes, while the value of this data will be estimated at $100 billion by 2027. Other impactful factors include the emergence of small data and TinyML development in the financial industry – rapid cognitive analysis of the most important data.
- Conversational AI and better customer engagement – hassle-free communication, clean and simple interfaces, and service quality are the major areas for the implementation of AI and ML technologies in banking and financial services.
- No-code AI – modular solutions that significantly cut down development costs and time-to-market of new financial apps adding more business value to the products have changed the financial industry. GiniMachine credit risk management is a great example of these changes.
- Metaverse impact – experts anticipate the growing impact of Metaverse on the financial services industry due to crypto, NFTs, and digital tokens.
Banks and financial institutions require strategic planning and gradual digital transformation of their legacy software to combat weaknesses and discover additional opportunities in their industry.
No 2. AI in healthcare
When analyzing the role of artificial intelligence in different sectors, the healthcare sector is among the first ones to mention. AI decision-making in healthcare has five most widespread use cases:
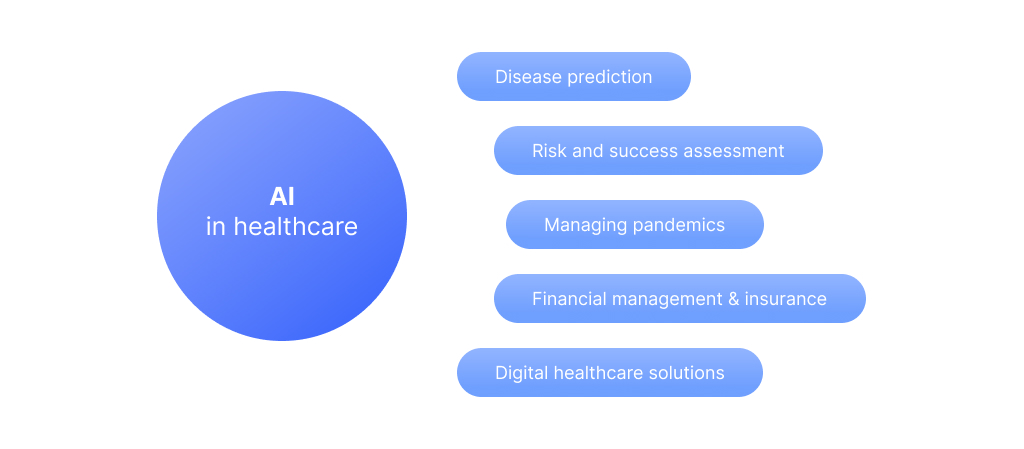
- Disease prediction with the help of statistical data – AI allows diagnosis and treatment of health issues at earlier stages due to the patient’s medical history analysis. The implementation of this type of AI in industry of healthcare allows for timely prevention, decreases therapy costs, and timely delivery of individual recovery plans.
- Risk assessment – AI-based decision-making systems implement the capabilities of neural networks and machine learning to assist medical personnel in the evaluation of risks and success in treatment.
- Managing pandemics and minimizing their impact – AI and ML in pandemic management can predict the behavior of viruses or pathogens. On a more global scale, technologies analyze and predict how a pandemic spreads and moves to identify the area of lockdowns and protect more people.
- Digital healthcare tools – AI in healthcare apps helps with diagnosis chatbots and data monitoring to alert potentially dangerous health conditions. Also, it includes Natural Language Programming that translates written or spoken notes into actionable patient data.
- Insurance and financial management in healthcare – AI helps to reduce the waste of funds in healthcare and increase the efficiency of services. Analysis of staffing, performance of healthcare facilities, and medication waste help avoid fraud and provide more tailored policies. AI-based risk management in insurance with the help of GiniMachine helps to assess realistic scenarios and minimize financial losses.
No 3. E-commerce
One of the industries using AI for quite a long time is ecommerce. Use cases of AI and ML in e-commerce are inspiring and able to change online shopping as we know it. With the help of AI, businesses can provide their customers with more tailored product recommendations, offer smart website searches using images, and discover e-commerce predictive analytics.
Also, artificial intelligence in business examples includes demand forecasting to boost sales efficiency due to improved predictions, proper human resources planning, lower risks of overstocking, and valuable market insights.
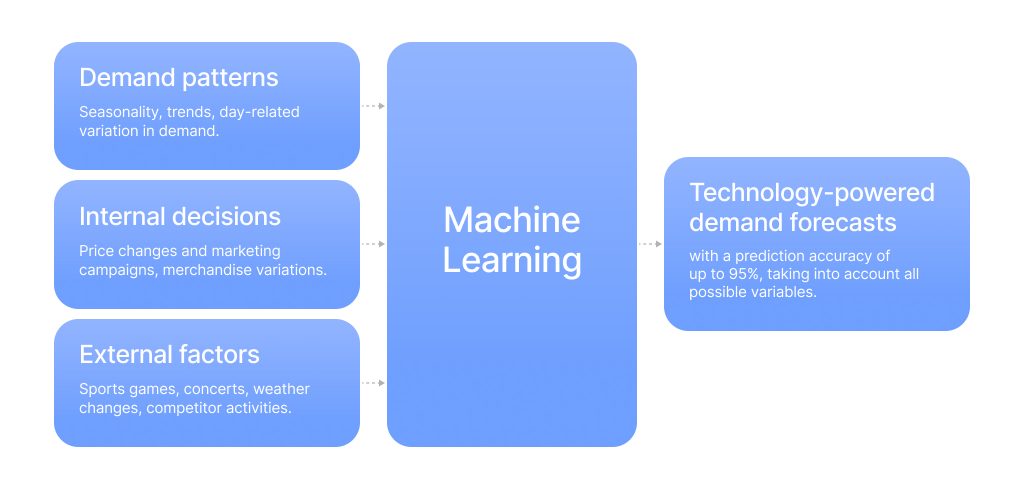
AI in e-commerce allows for improving customer service: according to estimates, 85-95% of online customer interactions are carried out with the help of AI. Chatbots and other communication tools help quickly find answers, reduce frustration rate, and to decrease the bounce rate.
No 4. Sales and marketing
The predictive marketing outreach is growing at a rocket speed: demonstrating over 23% year over year. It encompasses statistical techniques, like artificial intelligence and machine learning, predictive modeling, and data mining. These techniques are used to process data and assess the probability of certain future outcomes.
We notice practical examples of AI in marketing and sales daily. Such artificial intelligence in business examples are automatic up-sell suggestions: if a customer is interested in a bottle of Sauvignon Blanc, predictive analytics is more likely to suggest them premium oysters than a pack of popcorn. Advanced analytics can solve more challenging tasks. Artificial intelligence in marketing allows prioritizing customers by their readiness and likeliness to acquire the products with a higher margin or make returns.
Here are the five most widespread use cases of AI and predictive analytics in marketing and sales:
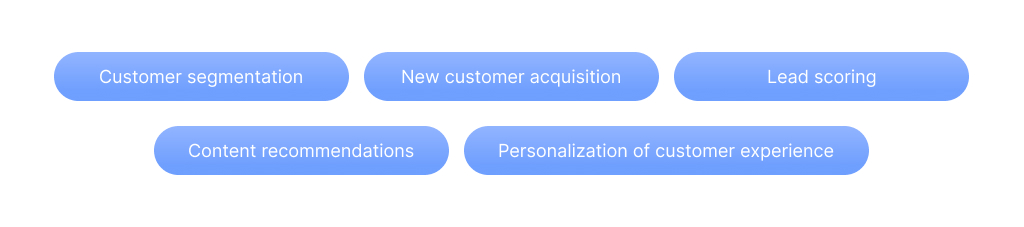
Customer segmentation via cluster modeling helps to get insights into customer behavior, demographics, and interests and back up the importance of these parameters. The technique is used in retail, streaming, sports science, email marketing, and health insurance.
For sales and marketing industries using AI, one more implementation option is churn prediction. It helps forecast customers likely to be lost and timely take measures on their retention, calculate the value of potential lost income, and identify customers ready to upgrade their payment plan.
Identification modeling helps target prospective customers, while AI/ML algorithms for lead scoring rank prospects according to their likelihood to convert. The benefits of predictive scoring include having a single trackable metric to analyze customer value, being able to create marketing campaigns with higher ROI, improving conversion and purchase rates, and obtaining reliable forecasts.
If you need software for predictive analytics lead scoring, GiniMachine provides a number of advantages: it is a 100% no-code AI platform. All you need to start scoring is historical data in a .xls/.csv file format, with user attributes and records. Each record needs to have a binary status: yes/no, good/bad, repaid/overdue, etc. You can build predictive models for scoring leads in a few minutes and get a detailed report with insights and diagrams.
Artificial intelligence role in business is unprecedentedly high now. Predictive analytics in marketing can be used for content recommendations that engage customers and shorten the sales cycle and for building personalized experience due to creative surveys, and incentives to help your target audience feel highly valued, not haunted.
No 5. AI in different fields for their lending solutions
GiniMachine use cases in application scoring are far more versatile than just lending in financial businesses. One of our customers is a telecom company that created an internal system to grant loans to their customers. The company implemented GiniMachine for being able to approve loans instantly and allow their customers to receive financing in no time.
The loan decision is based on a large amount of data, traditional user information (demographics, income, etc.) and alternative data available to the telecommunications provider, for example:
- Contract type
- Use of mobile Internet
- Use of streaming services
- Total day charge
- Monthly charges
- Payment methods
- Total day/night minutes
- Account length
- Voice mail plan
- Area code etc.
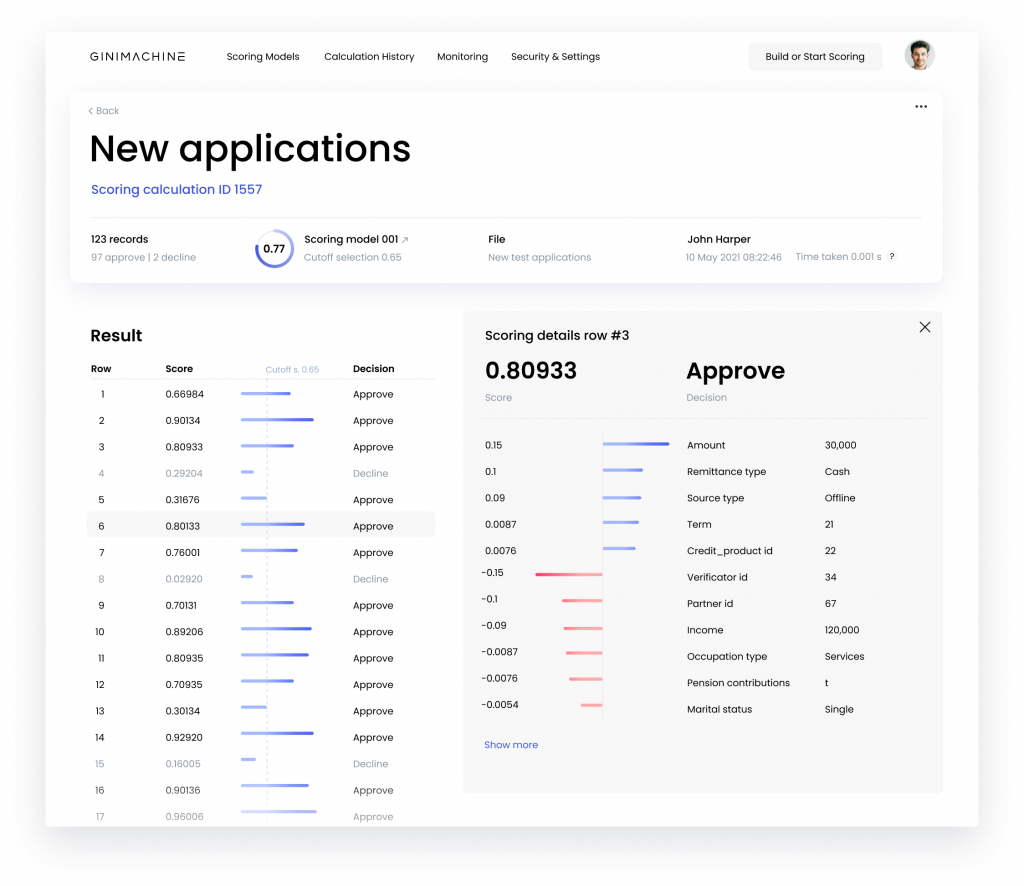
GiniMachine provides a combination of ML techniques to help automate decision-making and validate scoring models in 2-10 minutes depending on the dataset size.
No 6. AI in agriculture
The agriculture industry may implement AI for computer vision technology to monitor soil and crop in order to yield healthier crops, control soil erosion, support necessary conditions for plants and make farming less effort-consuming in general. The global spending on smart agriculture, powered by AI and ML, is expected to reach $15 billion by 2025.
With GiniMachine farmers can upload historical data and build predictive models to assess price and demand fluctuations. As for the artificial intelligence in different sectors of agriculture, there are platforms for agricultural lending and insurance, Gini algorithms are implemented in WizeRise along with OneSoil and HES FinTech solutions. The solution combines predictive analytics and precision agriculture to convert field data into actionable reporting. WizeRise helps to find valuable patterns in the loan application to keep risk officers better informed without leaving their offices.
No 7. Industrial AI
Predictive analytics in manufacturing is AI and ML tool that helps companies monitor, analyze and optimize their business processes. The role of artificial intelligence in business and manufacturing is crucial: it helps identify trends, detect future issues with machinery downtime or supply chain issues, helps predict financial troubles, and avoid them with proper planning. Industrial artificial intelligence may also engage the Internet of Things to monitor the condition of equipment and alert about unexpected situations.
AI in manufacturing improves workplace productivity, optimizes resource planning, predicts demand, and analyzes credit risks.
No 8. AI in insurance
The entire insurance industry is valued at over $5.5 trillion now. Forecasts say it can grow to over $6.3 trillion in the next 4 years, so most insurance businesses are searching for the tools to automate their work and optimize their risk profile. AI decision-making and predictive analytics in insurance are highly valuable due to their potential to filter tons of user data and make important decisions data-driven.
Back-office AI is not the only area of AI implemented by insurance companies. Client-facing decision-making in insurance can be improved with AI: optimize form filling and onboarding, build data models for faster lending decisions, etc.
Additionally, insurance can benefit from installing connected devices tracking the quality of a person’s driving in order to reduce fees for those who drive safer. Similar practices can be used in health insurance as well. Cognitive technologies are a point of interest for insurance businesses because they allow businesses to tailor their offerings based on customers’ behavior, optimize processes, and automate routine procedures.
No 9. Business decision-making
Using machine learning, businesses can create algorithms to learn from data and extract key insights for understanding business patterns and connections. Perhaps, the key role of artificial intelligence in business is that such tools provide intelligence on decision-making trends as more and more data is collected and processed.
For example, with a data-driven approach, ML can educate stakeholders on the decision taken in the past and provide analytics on correct and incorrect suggestions. This way leaders can eliminate errors and get a realistic picture of the consequences.
For sure, automated AI decision-making possesses certain risks. ML-based decision-making cannot tell you in 100$ cases that the collected data is high quality, but there is a lot done to avoid bias. For example, in GiniMachine we include a stage of predictive model validation to make sure it works as intended. Also, GiniMachine can process missing fields, and work with unstructured or raw data. Ensuring proper data evaluation, hiring experts for independent analysis, and using the proper underlying infrastructure with necessary security measures will help to avoid bad data infusions and flawed decisions in business.
No 10. Travel and hospitality
Travel and hospitality were among the top industries using AI before the pandemic. What’s with the AI in industry now?
In addition to face recognition technologies in airports, there are more AI-based services appearing for private companies to make traveling more affordable and pleasant. For example, AI in travel booking and flight price prediction, customer service AI in airports and hotels that use robo-assistants, and more.
More specific AI-based apps include AI for travel disruption management that suggest alternative solutions in case of bad weather or other delays. Just like many other industries, travel and hospitality may benefit from customer churn prediction with the help of AI. GiniMachine predictive analytics uses historical data to identify hidden patterns and inform companies about the risk of canceling their reservations or flights. AI data analytics in hospitality is widely used in luxury hotels and resorts. Analyzing visitors’ sentiments, the system may improve guest experience, timely reveal problems, and send notifications about the necessity of human intervention.
AI in travel and hospitality has multiple options in the future. It may include more voice assistants and robots helping tourists, smart baggage handline, augmented reality, end-to-end travel planning, etc.
To Sum It All Up
AI solutions are applicable to almost any industry we know: from sales and marketing in retail businesses to manufacturing and healthcare. From credit scoring and risk management to travel agency chatbots and robot assistants.
We have no idea what’s going to happen in the future, but the only thing we know for sure is that artificial intelligence and machine learning have great potential to improve the life quality of people by taking care of their well-being, fostering great business decisions, offering inclusive financial services, and much more.
Book a free 15-min demo to explore how GiniMachine can benefit your business.