AI in Demand Forecasting or How to Boost Sales Efficiency
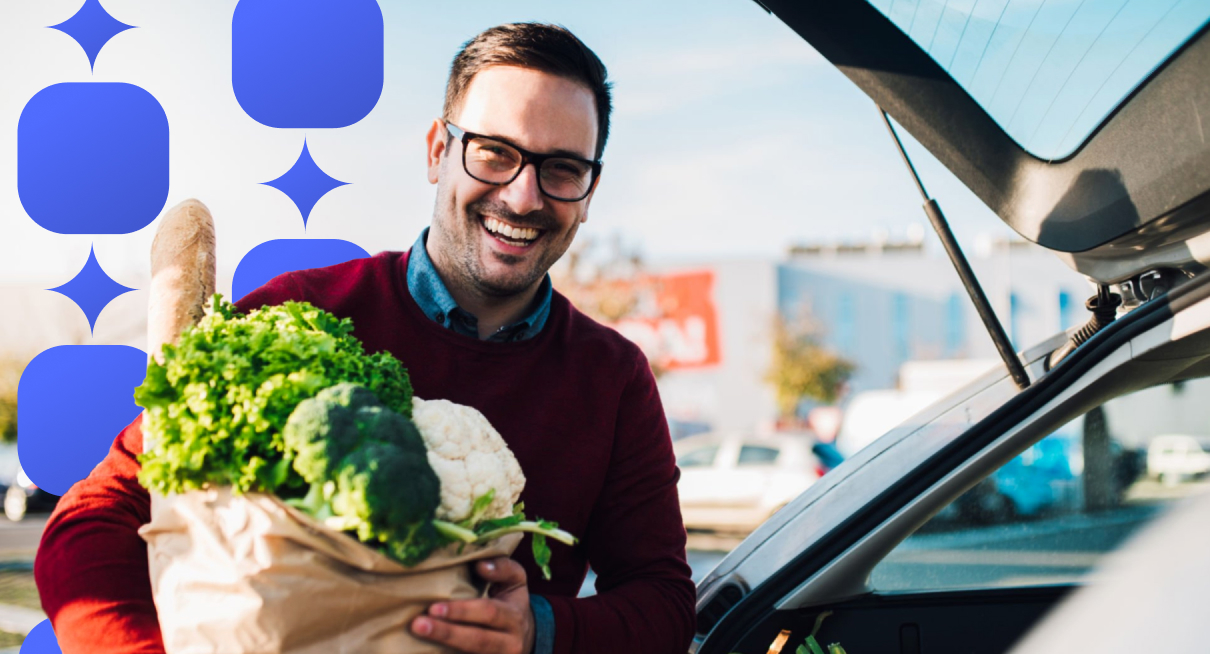
Businesses face various inventory challenges dealing with supply chains. To ensure smooth processes and high margins, mid and large-scale retailers should plan and organize millions of goods flows day after day. If they fail to predict the demand for a specific product, the company is at risk of littering the warehouse with expired goods and losing millions of dollars. Using AI for demand forecasting, retailers can reduce supply chains and improve financial and capacity planning.
According to Gartner‘s CSO Priorities Pulse Survey, 88% of sales offices have already invested in or are going to invest in AI for sales purposes, and demand forecasting is the most widely requested AI demand driven forecasting application today. It’s highlighted that 45% of companies are already using AI demand planning solutions and 43% of them are considering investing in this software by the end of 2022.
Benefits of Artificial Intelligence Forecasting Methods in Sales Planning
All this buzz around technologies in sales is quite as expected since the use of AI and machine learning in demand forecasting can highly predict which goods are needed for each store and supply channel – a great way to maintain minimal stock risks. With a dedicated solution at hand, retailers can ensure the right amount of staff in stores and distribution centers, support capacity management, help manage long-time purchasing, and manage risks.
Here’re the advantages of using AI for demand forecasting:
Improved accuracy of predictions
Despite AI software providing highly accurate predictions, it is designed to always do better. With the power of machine learning algorithms, the forecasting software learns from existing data and makes better predictions over time.
Improved human resources planning
The implementation of AI in demand forecasting can support the Human Resources department to plan their scope of work for the upcoming year as well as make an efficient timetable by combining part of full-time employees. This is a suitable way to optimize the costs and effectiveness of the HR department.
Increased customer satisfaction
Stockouts can reduce customer satisfaction and lead to growing churn rates. Having planned the demand strictly, you can improve brand perception and build a loyal customer base.
Minimized risks of overstocking
Overstocking is the opposite challenge for retailers when products stay unsold longer than expected. This results in higher inventory costs and an increase in risks of, for instance, products becoming overdue or obsolete and ultimately losing their value. The company will have to sell these products with a reduced margin. But AI for demand forecasting can minimize risks by predicting customer behavior and their purchasing needs.
Sustained business development
Accurate demand driven forecasting helps plan business strategies and focus on strategic issues rather than countering stock issues. With a good market forecast, businesses can thrive without fears of unexpected risks and challenges.
AI and Machine Learning in Demand Forecasting
According to McKinsey, AI and ML forecasting can reduce errors by up to 50% in supply chain networks, leading to around a 65% cut in lost sales and up to 40% decrease in warehousing costs.
A human is unlikely to analyze a full range of potential variables and estimate an accurate prediction for every product type. But machine learning algorithms can consider the impact of every variable for decision making in detail. This is extremely important since ML not only can handle thousands of different factors but also distinguish those that have the greatest impact on demand planning and exclude unnecessary variables. Imagine that only weather data can consist of different important factors that potentially impact demand. What is more, no-code ML platforms learn from your prediction models and automatically improve their future forecasting models. The primary advantage of this process is that AI and ML solutions like GiniMachine do not require any coding experience or Ph.D., they’re easy-to-use and one-click. So, it’s not surprising that many retailers today are choosing machine learning in demand forecasting over traditional processes.
Traditional forecasting models are getting outdated since they allow analyzing a limited amount of data. With the implementation of machine learning into demand forecasting, companies can improve their accuracy of predictions by taking into account thousands of data and variables, such as demand patterns, business decisions, external factors, and others.
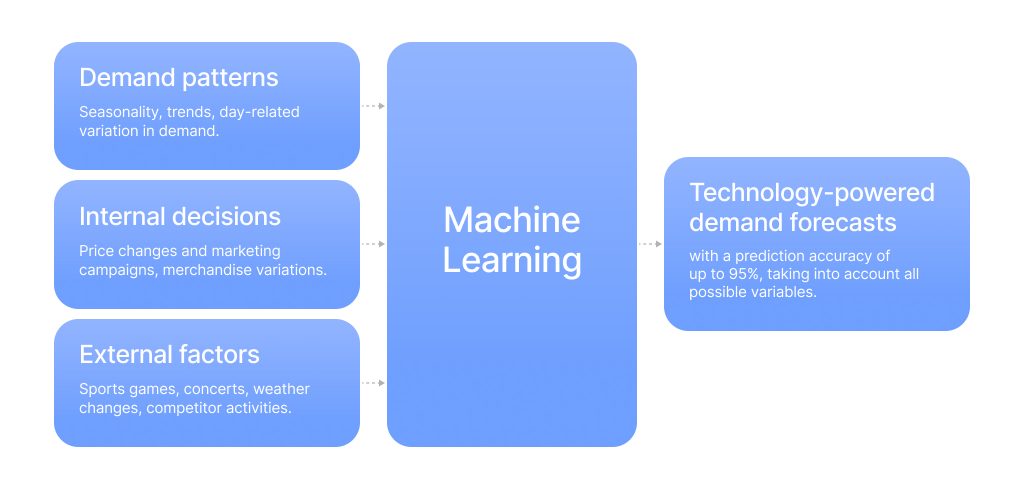
Demand factors affecting sales planning
Planning sales, retailers need to consider recurring factors such as weekdays, seasonality, holidays, and others. Finding the dependencies between decrease and increase in demand with specific days and seasonal changes is a true approach to managing warehouses and sales. Based on these data, retailers can set up their marketing campaigns and change prices to make a profit.
Artificial intelligence forecasting methods in combination with machine learning can capture the interaction of multiple factors, like a day of the week, marketing campaign, and weather. To give an example, during a snowfall retailers can launch a campaign promoting their snow tubings and note a much bigger demand for the product.
Internal business decisions impacting demand
Any business decision taken by a retailer is a significant source of demand variation, from ad campaigns to pricing adjustments and merchandise changes. And demand driven forecasting with AI allows us to accurately predict how any change might affect the product’s demand. This capability is highly valuable for promotion forecasting and understanding the behavior of customers. In addition, ML can help see the effect of a promotion campaign of one product on the demand for the other.
External factors influencing sales and demand
The use of concert and sports dates, competitor prices, weather data, and others is an example of external factors also affecting the demand. Specialists indeed can check weather forecasts manually and take an ordering decision, like purchasing umbrellas before a rainfall season. However, it’s hard for a human to adjust forecasts slightly downwards in demand during several sunny days of this season. While a demand forecasting solution with AI and ML power can take it into account.
Based on surveys, considering all possible weather changes in the demand forecasting process can reduce errors by up to 15%.
AI Demand Planning: Where to Start?
Working with a demand forecasting solution, any business should note that AI tools have preventable pitfalls. To avoid unfortunate and inaccurate forecasts, we recommend exploring the following:
- Demand forecasting is based on historical data collected by a business. But new products don’t have sufficient historical data. Thus organizations should not expect highly accurate predictions of demand for newly released products.
- The accuracy of predictions highly depends on the input data. Companies need to make sure that all important challenges and market changes are reflected in their data sources.
- Excluding the marketing data from the demand prediction may increase the error margin since they highly impact future sales.
Want to integrate predictive analytics into your demand planning process? Don’t hesitate to contact us and get a free demo tour.