Predictive Lead Scoring or How to Make Accurate Sales & Marketing Decisions
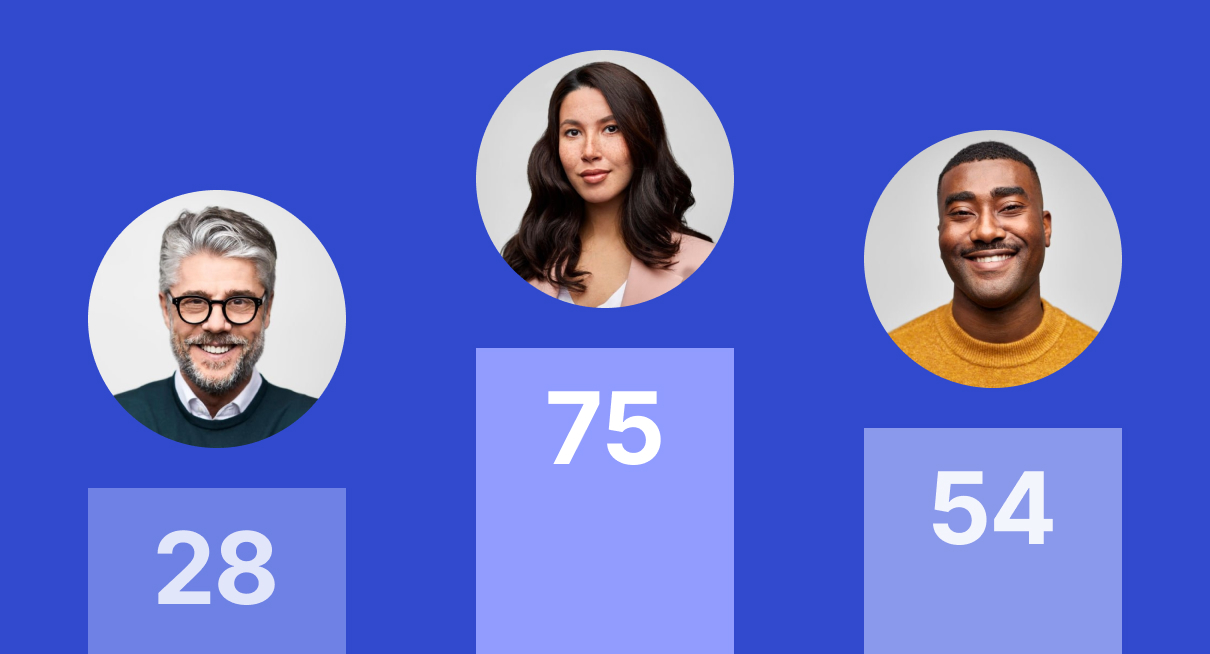
A sales team has to prioritize to find the “best” customers quickly and save the “less likely” ones for last. However, the traditional scoring method is risky since it’s not immune to human errors, data losses, and inaccuracy of counting in general. So, modern companies prefer to adopt AI predictive scoring – an investment in the ability to understand their customers, products, and services by leveraging data-driven models and scoring indexes.
In this article, we’re going to discuss the benefits and limitations of predictive lead scoring, important historical data needed to build a reliable scoring model and review a lead scoring tool called GiniMachine.
What Is Predictive Lead Scoring?
Predictive lead scoring takes the traditional lead scoring to the next level by applying machine learning algorithms, AI, and big data to target the right prospects and improve the efficiency of sales and marketing. Predictive analytics allows evaluating the key behavior patterns of customers and ranking them in order to distinguish those who’re more likely to convert, retain, and buy products or services. But why AI lead scoring is relevant now?
Poor lead prioritization process can lead to a company’s decline when sales targets get missed and teams get demoralized. Also, a poor approach might result in stagnation when sales personnel fail to learn from their previous experience. This can happen when a team works with leads with wildly varying intent. Without a clear strategy of working with every type of customer, a team will see only customers who’re not ready to make a purchase. As a result, a team can lose the motivation to keep selling and developing new sales tactics.
What Are the Benefits of Lead Prioritization Analytics?
If you’ve never ever used predictive analytics for lead scoring, it’s like hiring another department to assist with customer acquisition. The only difference is that you’ll need one specialist to work for the department. Toby Murdock claims that predictive lead scoring is the best option for companies that have lots of data to leverage – more data deliver more impactful scoring models and accurate lead scores.
According to VentureBeat, 90% of users believe that predictive lead scoring provides more value than traditional scoring approaches, and 98% claim that they would purchase a lead scoring platform again. In addition, 88% of respondents prove that they’re receiving value from their predictive analytics software.
Here’re the benefits of predictive scoring:
- Predictive sales intelligence creates a single trackable metric that reflects the perceived value of customers.
- Predictive analytics allows creating more focused marketing campaigns and investments for maximizing ROI from the marketing expenditures.
- It boosts the productivity of sales teams by optimizing their time and effort spent on working with high-quality customers.
- Predictive analytics improves the conversion and purchase rate by aligning sales objectives with the acquisition.
- In addition, as more data is accumulated in the lead scoring tool, forecast quality will increase, further improving sales.
How Lead Scoring Platform Works
The lead scoring process starts with collecting historical data and scoring attributes as well as identifying the target metric. It can be conversion, yield, churn, retention, and more. What is more, you need to train a scoring model based on a variety of key attributes to capture all aspects of your customer’s lifecycle. During the process, you may find out that some attributes are useless and can be excluded from lead scoring. The model will evaluate relations between attributes and predict the possible behavior of customers and score them based on the propensity to convert, for instance.
Here’re the key attributes to include in the predictive score model:
- Customer profile data to measure the core client attributes such as demographics (age, location, job title, and more).
- Account data to consider significant firmographic attributes (industry, company size).
- Customer intent data to measure their attitude towards products and services as well as their willingness to contact your marketing and sales teams for further communication.
- Customer engagement data to capture their web activities such as click-through rate, bounce rate, CTO, contact form fill-out, time spend on a page, product page views and downloads, account creation, and more.
- Purchase data of existing customers such as the amount of money spend on a product, the number of bought goods or services, existing discounts on demanded products, time and frequency of purchase, etc.
- Marketing and sales campaigns to understand their influence on the demand. It’s important to manage acquisition channels such as direct, paid, social networks, referral, and more.
The list of historical data will vary depending on your business type. However, once you’ve collected the key data points for your business, the information will be processed by a predictive sales intelligence system to build a scoring model and evaluate the relationship between the attributes to predict the behavior of your leads.
Predictive Analytics Lead Scoring: Where to Start
If you’re looking for AI and ML-powered software for predictive analytics lead scoring, GiniMachine is a great option. One of the main advantages of this platform is that it doesn’t require any coding experience or Ph.D. – it’s a 100% no-code AI platform.
To start scoring with GiniMachine, you need to collect historical data on your existing customers (1,000 data points would be perfect). You need to prepare the .xls/.csv file format that contains the attributes/parameters and records. Each record must have a status or outcome) where 1stands for repaid, 0 – overdue. Once the file is done, you can upload it to the platform.
It’ll take about two minutes to build a model that saves hundreds of hours of manual work for data analysts. When a model is built, your team can check its accuracy and change analyzed attributes, if needed. The system will deliver a detailed report with all valuable insights and diagrams significant to analyze the model. When the model is perfect, you can start scoring your leads.
Additionally, GiniMachine automatically divides input data into training and test records at a ratio of 70% to 30%. The system will build a model and then check it against the test records. As a result, the quality of every model will grow time after time.
To avoid unfortunate forecasts, we recommend taking into account the following limitations of AI lead scoring software:
- The more historical data you upload, the more accurate the scoring model you get. Thus it’s impossible to build a reliable model if you’re entering the market or launching a business without any previous experience.
- The accuracy of predictions highly depends on the quality of entered data. So, make sure that you consider all possible attributes and parameters.
Looking for a lead scoring platform? Contact us to try GiniMachine in action.