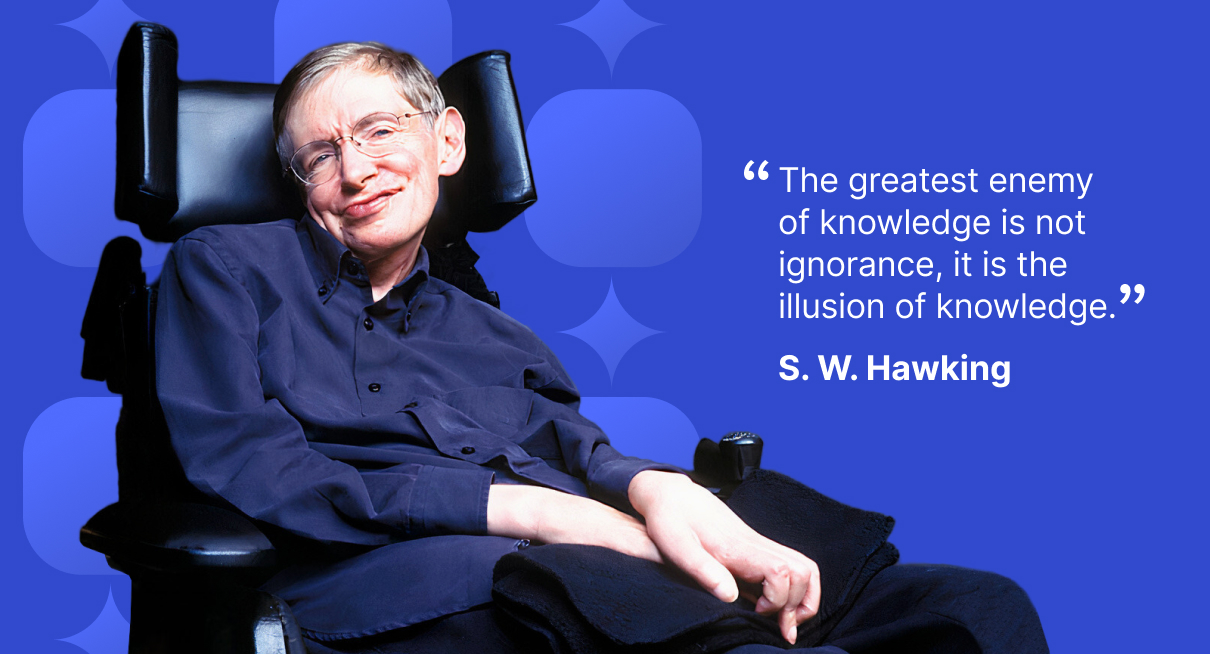
Marketers, data analysis specialists, and other people whose job relates to information about current and potential clients strive to use the data-driven approach when making decisions.
But how much data can we process and analyze?
Can we consider all the multiple factors when making decisions?
And how can we improve our analytical abilities?
Information Paradox
To understand the main problem of the data-driven approach, we need to step back and learn about the information paradox – one of Stephen Hawking’s theories. He suggests that the information is stored in the event horizon – the boundary of the black hole. And when the data returns from there, it is chaotic and useless for practical purposes.
But how can this theory be applied to business?
According to the Kapta survey, around 70% of CEOs they interviewed indicated the lack of relevant data as their main issue. However, other research shows that businesses don’t use up to 80% of the data they already have.
So if we continue with our black hole analogy, the array of data an organization collects – like information on the event horizon – is often so chaotic and unstructured that it is barely possible to use. And therein lies the paradox: we spend thousands of dollars to gather, compile and maintain data, thinking we need more information, but at the same time, we don’t use the majority of it.
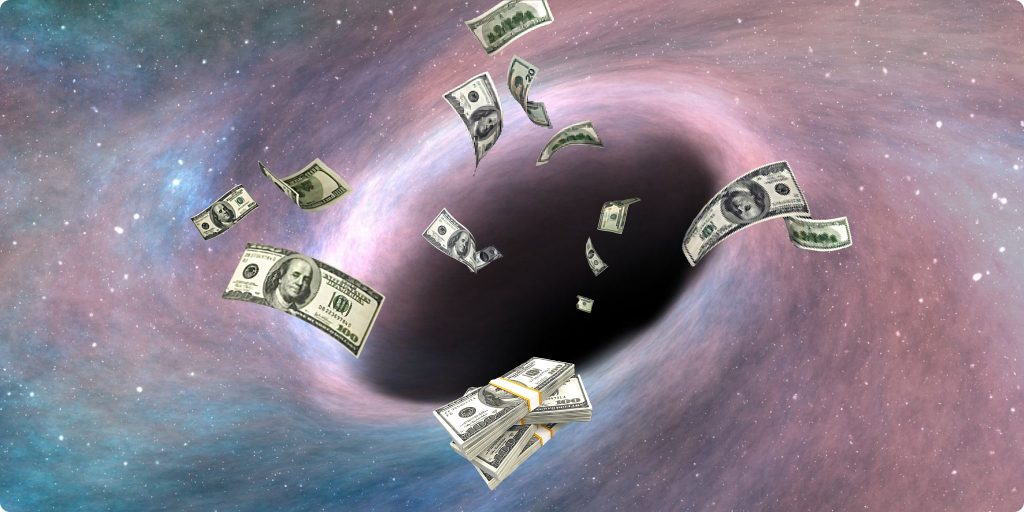
As a result, the information paradox leads to a significant loss of resources. Here are a few examples from the IDC report “The High Cost of Not Finding Information”:
- On average, 36% of work time is spent gathering and merging information from different sources.
- The inability to find knowledge resources, also known as knowledge deficit, costs organizations an average of $5,850 per worker annually.
- Companies lose over $16MM per year on average for recreating existing knowledge.
What causes the information paradox?
Big data is not always good data. The way you structure and analyze it is as important as the data itself. One of the causes of the information paradox is outdated technologies. And when you don’t have the right tools to work with data, you won’t be able to get the most results.
To solve this issue, you need to implement new technologies like AI-powered analytics into your business process.
How AI Analytics Works
First off, we need to understand that data-driven decision-making and AI data analytics are similar yet different concepts with different processes.
The traditional decision-making process can be split into four steps:
- The data analysis process starts with gathering big data and passing this information to machines
- Then machines reduce and summarize data the way humans can understand it
- After that, these summaries are pressed to humans that look through and analyze them
- Finally, based on the data analyses, specialists suggest business decisions.
As you can see, in this process, human judgment is a central processor. And depending on managers’ qualifications, experience, and personal qualities, the results may differ. Moreover, humans can process a limited amount of information compared to machines.
But implementing AI analysis can help you to overcome these factors. AI like GiniMachine can process thousands of lines of information and normally doesn’t have any human biases.
However, AI and predictive analytics work independently if the final decision can be made using structure data only. If you need to consider, for example, cultural aspects of the situation or other non-digital data, you still need humans to be involved.
So the process that combines the powers of AI and human expertise can work like this:
- The machine takes care of the first three steps of decision-making. First, it gathers and prepares data.
- Next, the AI works with the available data using machine learning analytics.
- And as a result, AI offers possible solutions.
- Then the manager analyzes these solutions using the other non-digital information and makes corrections if needed.
- Finally, specialists choose the best business decisions based on the analysis.
During this process, humans don’t interact with the data itself, just with the possible solutions offered by AI. This allows you to avoid the downsides of human judgment but still get a rational result by involving managers in the final steps of decision-making.
How to Implement AI-Driven Decision-Making into Your Business
Say you have decided to switch to the AI-Driven decision-making process. What should be your first steps?
Step #1. Centralize all data
To let AI analyze the data and make decisions based on it, you need to gather all the information from different sources in one place. And the more different sources of information you have, the more difficult this task will be. However, if you already use some ERP or CRM, you can simply look for the integrations that will allow you to connect all the other sources with the main system. Otherwise, you can use ETL tools that automatically synchronize and upload information to a data warehouse.
Step #2.Set up a cloud-based storage system
After the centralized data warehouse is configured, you need to prepare for the constantly increasing amount of data you gather. To reduce maintenance costs, you can choose a cloud-based storage solution. They are easily scalable, which will make the work of the IT team easier. After this, you can query and analyze the data.
Step #3. Build a foundation for AI analysis
Finally, you need to think through how to integrate AI into your current business process. Analyze what data is used for each use case and decide whether you need to develop a custom solution and maintain it using an in-house team or choose a pre-build option like GiniMachine from a third-party provider and outsource this task.
As you can see, the AI-driven approach to the decision-making process is essential for today’s business. The amount of data accumulated by companies keeps growing, and this trend will become even stronger in the future with companies forced to switch to zero-party data. As a result, you need to implement new tools to keep your decisions smart because humans are already not capable of processing all this information.
Ready to build an AI-based decision-making process? GiniMachine can help. Sign in and start a free trial to see AI in action.