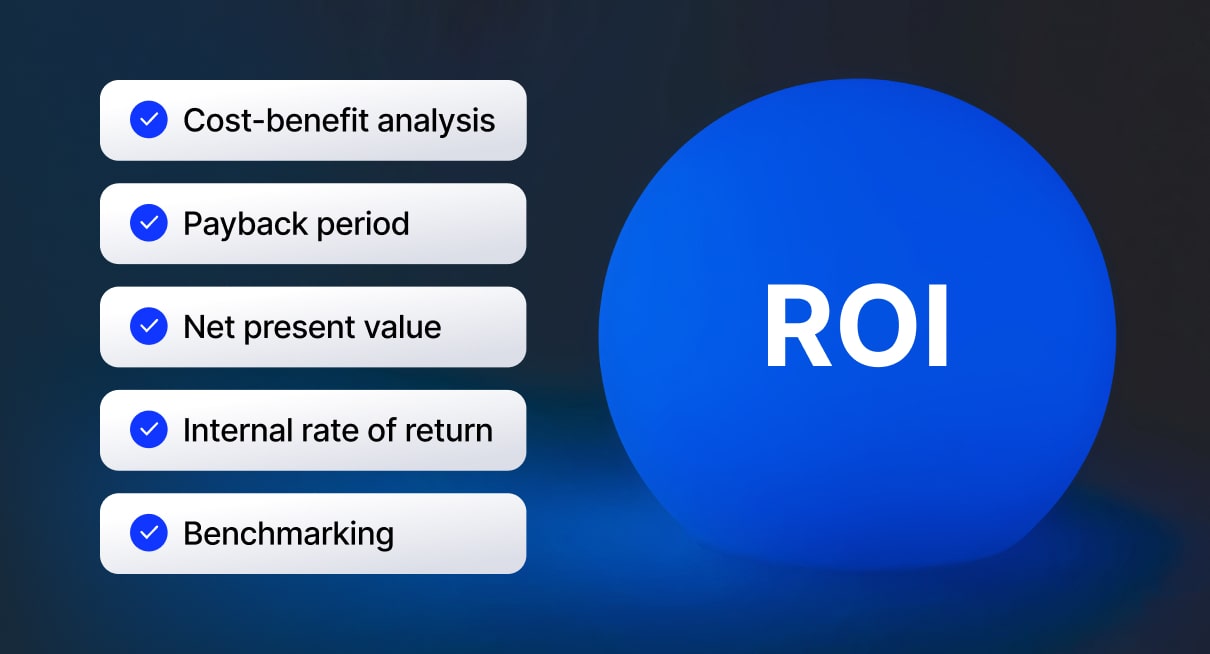
Artificial Intelligence (AI) now became a fundamental component for the future of financial services. According to a report by Autonomous Research, AI technologies could reduce operational costs for financial services companies by up to 22%, amounting to savings of $1 trillion by 2030.
More Stats
- Accenture revealed that 76% of banking executives believe AI is crucial for differentiating themselves in the market.
- A PwC report estimates that AI could contribute up to $15.7 trillion to the global economy by 2030, with financial services being one of the primary beneficiaries.
Whether it’s credit scoring, fraud detection, or personalized customer service, AI technologies are enabling financial organizations to make quicker, more accurate, and more efficient decisions.
While the benefits of AI are evident, the implementation of such technologies comes with its own set of challenges and costs. Financial institutions are often faced with questions like, “What will be the return on this investment?” or “How long will it take to recoup the costs?” Understanding the Return on Investment (ROI) is crucial for justifying the allocation of resources towards AI technologies. It provides a quantifiable measure of the effectiveness of the AI solution in achieving business objectives, be it cost reduction, revenue growth, or customer satisfaction. Therefore, a comprehensive understanding of ROI is not just advisable but essential for any financial institution planning to implement AI solutions.
In this article, we will delve deeper into the intricacies of calculating ROI for AI implementations in financial services.
The Current State of AI in Financial Services
But first, let’s do a quick overview of how AI is used in two critical areas of financial services: credit scoring and fraud detection.
AI in Credit Scoring
Traditional credit scoring methods often rely on a limited set of variables and can be time-consuming. AI, on the other hand, uses machine learning algorithms to analyze a vast array of data points, including social media activity, transaction history, and even behavioral patterns, to assess a borrower’s creditworthiness. This kills two birds with one stone: it speeds up the loan approval process but also makes it more accurate.
We at GiniMachine offer artificial intelligence credit scoring that can even cater to thin-file borrowers who would otherwise be overlooked by traditional systems.
AI in Fraud Detection
Machine learning algorithms can analyze transaction patterns in real-time and flag suspicious activities, significantly reducing the rate of false positives and improving the detection of fraudulent transactions. Capgemini found that 75% of financial institutions with AI implementations saw a 10-20% reduction in fraud cases. These systems learn from each transaction, becoming more effective over time.
Learn More About AI Fraud Detection
Why ROI Matters and How to Calculate It
The concept of Return on Investment (ROI) helps financial institutions make informed decisions about implementing AI solutions by weighing the costs against the benefits. In this section, we will explore why ROI matters, focusing on the cost of implementing Artificial Intelligence and the value of data-driven decision-making.
Implementing AI in financial services is a significant investment that goes beyond the initial purchase of software. Costs can include:
- Software licenses: The cost of the AI software itself, which can vary widely depending on its capabilities.
- Hardware requirements: Specialized hardware may be needed to run complex AI algorithms efficiently.
- Data preparation: Cleaning and organizing data for AI algorithms can be time-consuming and costly.
- Training and development: Employees may need training to understand how to interact with the new AI systems.
- Maintenance and updates: AI systems require regular updates and maintenance to stay effective.
These costs can add up quickly, making it essential for financial institutions to have a clear understanding of the ROI to justify the investment.
5 Methods for Measuring ROI
Cost-Benefit Analysis
In this method, the first step is to calculate the total cost of implementing the AI system. This should include all the costs mentioned above. Once it’s calculated, it should be compared with the benefits gained. These benefits can be quantified using Key Performance Indicators (KPIs) such as cost savings, time efficiency, revenue growth, and customer satisfaction. The difference between the benefits and costs gives you the net benefit, which can be used to calculate ROI.
Payback Period
The Payback Period is the time it takes for the AI system to “pay for itself.” In other words, it’s the period during which the cumulative benefits equal the initial and ongoing costs of implementation. This is a straightforward method but is most effective for projects where the benefits are expected to be realized in the short term.
Net Present Value (NPV)
Net Present Value (NPV) is a more sophisticated method that takes into account the time value of money. It calculates the present value of all future cash flows—both inflows (benefits) and outflows (costs)—and subtracts the initial investment. A positive NPV indicates that the projected earnings (in present dollars) exceed the anticipated costs (also in present dollars), making the investment profitable.
Internal Rate of Return (IRR)
The Internal Rate of Return (IRR) is the discount rate that makes the NPV of all cash flows from the AI investment equal to zero. In simpler terms, it’s the rate at which an investment breaks even in terms of NPV. A higher IRR indicates a more profitable investment. Financial institutions often use IRR in conjunction with NPV to assess the profitability of an investment over time.
Benchmarking
Benchmarking involves comparing the performance of your AI system against industry standards or competitors who have also implemented AI. This can be done using the same KPIs used in the Cost-Benefit Analysis. Benchmarking provides a relative measure of ROI and can offer insights into how well your AI implementation is performing in the broader industry context.
What Makes GiniMachine a Good Investment
Investing in Artificial Intelligence (AI) for financial services is a significant commitment, both in terms of time and financial resources. However, not all AI solutions are created equal. GiniMachine stands out as a particularly good investment for several reasons, acting as an ROI multiplier for financial institutions.
Tailored Solutions
GiniMachine offers AI solutions that are customized to meet the unique needs of each financial institution. Whether it’s credit scoring, loan approval, or debt collection, GiniMachine’s algorithms are designed to adapt to specific business models and customer demographics.
Speed and Efficiency
Time is money, and GiniMachine understands this well. As seen in the case of Personalized Loans, GiniMachine can dramatically reduce the time required for loan approvals, allowing institutions to process more applications and, consequently, increase revenue.
Risk Mitigation
One of the most significant advantages of GiniMachine is its ability to accurately assess and mitigate risks. For example, the auto lender in South Asia saw a substantial improvement in their credit scoring model’s predictive power, reducing the likelihood of loan defaults and potential financial losses.
Cost Savings
By automating various aspects of financial assessments and decision-making, GiniMachine helps reduce operational costs. Kit Lean Technologies, for instance, saw significant cost reductions by automating their SME assessment process with GiniMachine.
Data-Driven Insights
GiniMachine’s advanced analytics provide actionable insights that can inform strategic decisions, from resource allocation to investment in new market segments. This data-driven approach ensures that every decision you make is backed by reliable data, maximizing ROI.
Success stories of our clients
Don’t take our words for it. Let success stories of our clients speak for themselves.
Kit Lean Technologies
Kit Lean Technologies leveraged GiniMachine to automate their Small and Medium Enterprise (SME) assessment process. Prior to implementing GiniMachine, the company relied on manual methods that were both time-consuming and prone to human error. What they have now is:
- Automated data analysis: The AI algorithms automatically analyzed various data points to assess the creditworthiness of SMEs.
- Significant time savings: The automation reduced the time required for each assessment, allowing the company to process more applications in the same amount of time.
- Cost Reduction: The time savings translated into significant operational cost reductions, contributing to a positive ROI.
Read more about the Kit Lean Technologies case.
Personalized Loans
Using GiniMachine, they revolutionized their loan approval process with remarkable outcomes. The approval time was slashed from 30 minutes down to a mere 5 minutes per application. This heightened efficiency led to a 40% surge in the number of loans issued weekly. Even with this uptick in volume, the company maintained its risk parameters, all credited to GiniMachine’s precise risk evaluation.
Read more about the Personalized Loans case.
Auto Lender in South Asia
An auto lender in South Asia used GiniMachine to improve their credit scoring model. The results were significant:
- Predictive power: The Gini index of their credit scoring model improved from 0.4 to 0.75, indicating a more reliable predictive model.
- Risk mitigation: The improved model allowed for more accurate risk assessment, reducing the likelihood of loan defaults.
- Competitive advantage: The enhanced predictive power gave the lender a competitive edge in the market, contributing to a positive ROI.
Read more about the Auto Lender case.
Final Thoughts
Understanding ROI is essential for the successful implementation of AI in financial services, and GiniMachine offers the tools and expertise to ensure that this ROI is not just positive but maximized for long-term success.
Ready to implement it to your credit business? Contact our team to get started today.