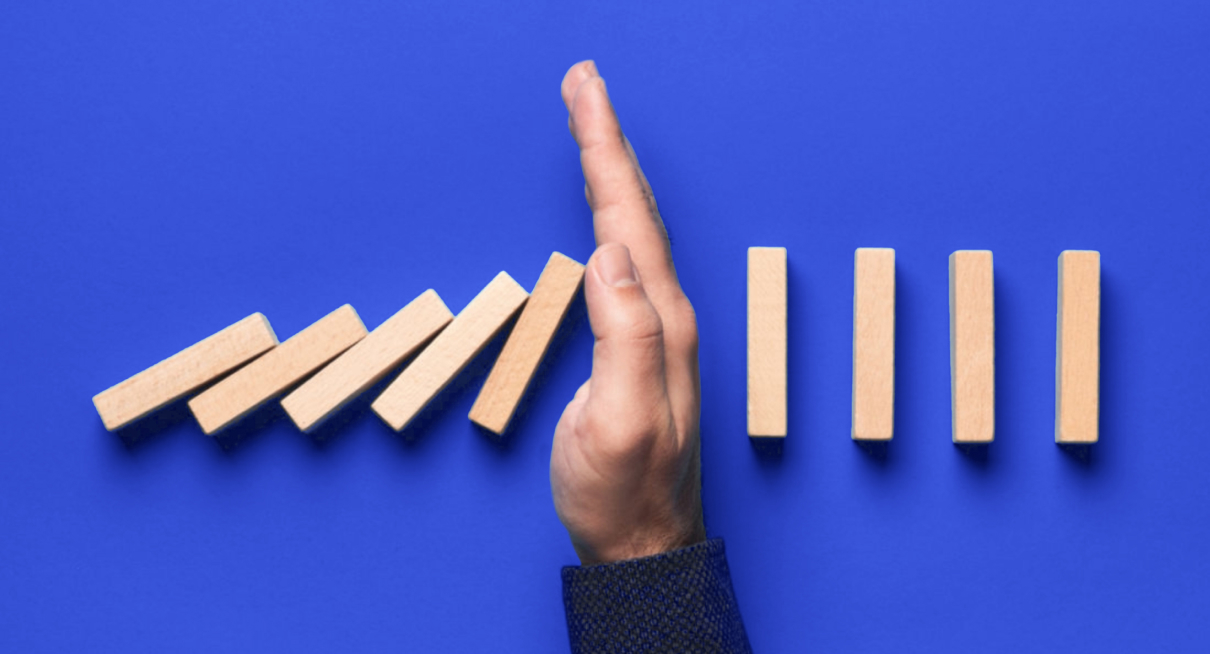
Businesses are accumulating almost unbelievable amounts of data daily. Trying to use this data to their advantage, they explore the possibilities of predictive analytics offered by Artificial Intelligence for decision-making.
AI is helping companies to be one step ahead of competitors by processing tons of data, generating real-time insights, and eventually by making better strategic decisions. AI and ML combined with Data Science have a growing role in the managerial decision-making process.
What is the potential of AI software for decision-making?
The estimates are quite rough. According to the research of McKinsey’s Global Institute, by 2030 AI is potent to increase the world’s economy by $13 trillion. According to 86% of respondents in a PwC research, their businesses have recently increased AI investments and accelerated their AI adoption plans, so they named AI a mainstream technology for their businesses in the early 2020ies.
Gartner’s survey unveils the fact that the existing manual decision-making in business becomes insufficient and untenable, and would benefit from AI assistance. According to the paper, more than a half – 65% of decisions are now more complex than those businesses needed to make several years ago.
AI/ML software is creating an impact in various fields: from smart recommendations in the entertainment industry to healthcare decision support systems, and automated marketing efforts. A lot of companies are already AI-centric benefiting from their AI investments. Some of them are almost there, exploring the prototype or the concept phase of AI software development and hoping to launch it someday. But there are still a lot of businesses not planning or being not able to turn their AI adoption ideas into reality.
What is hindering the AI adoption for decision-making?
Procrastinating the digital transformation in the field of Artificial Intelligence may have various roots. Let’s consider the most popular ones.
Lack of understanding
If the world had more successful and inspiring AI use cases nearby, business people would have a clearer understanding of the AI technology potential. Even though AI appeared around 50 years ago, its practical implementation in business has started in the 2010s. Therefore, a lot of enterprises don’t have enough technical resources with high theoretical knowledge and practical skills in AI/ML.
This is only a part of the problem. Most organizations don’t really have the platforms and the tech stack to do with AI. All that is slowing down the adoption curve.
The solution:
Perhaps, they need time and continuous exploration of the technology to entrust AI with the privilege to run the core business processes. Another way out is to consider external consultants and software vendors with proven expertise in AI and ML to create the necessary infrastructure and implement the business requirements.
Improper work with data
It’s not always clear which data types are useful, and which ones are not. For successful and truly data-driven decision-making businesses need accurate insightful datasets. Сompanies build standard data architectures to gather data in a single place. As a result, they have raw unstructured data, which is very difficult to work with.
The solution:
In most cases, AI algorithms require structured data, so the solution is to introduce data structuring solutions.
At the same time, GiniMachine decision-making software can work with raw unstructured data as well. Its intellectual classification can automatically sort the data fields and measure their importance, work with missing fields and make use of almost any dataset. GiniMachine is a no-code AI solution, which means that businesses require no staffing with machine learning engineers. The operations are carried out via an intuitive interface, with all reports and dashboards at hand.
Excessive expectations
Even understanding that software investment pays off in a while, investors may expect quick returns. Improved decision-making is directly linked to increased revenues, but custom AI development, integration, and adjustment usually take time.
The solution:
For a shorter payback period, businesses may consider readymade AI solutions instead of custom AI development. GiniMachine is a ready-to-use platform that requires no engineering skills. The associated costs are significantly lower when compared to custom AI development, which means a shorter payback period and higher ROI.
To Sum It All Up
When considering the automation of decision-making, it is important to analyze the business need, break it down into pieces and start working on it step-by-step. AI is a great assistant in decision-making, which is potent to meet the business needs related to cost savings, optimizing the workforce, or removing the process bottlenecks.
To become AI-literate, it is important to gradually work on this AI literacy, to understand what AI can and cannot do, and have a mid-term plan in your mind.