Customer Scoring Without a Credit History: Best Practices Today
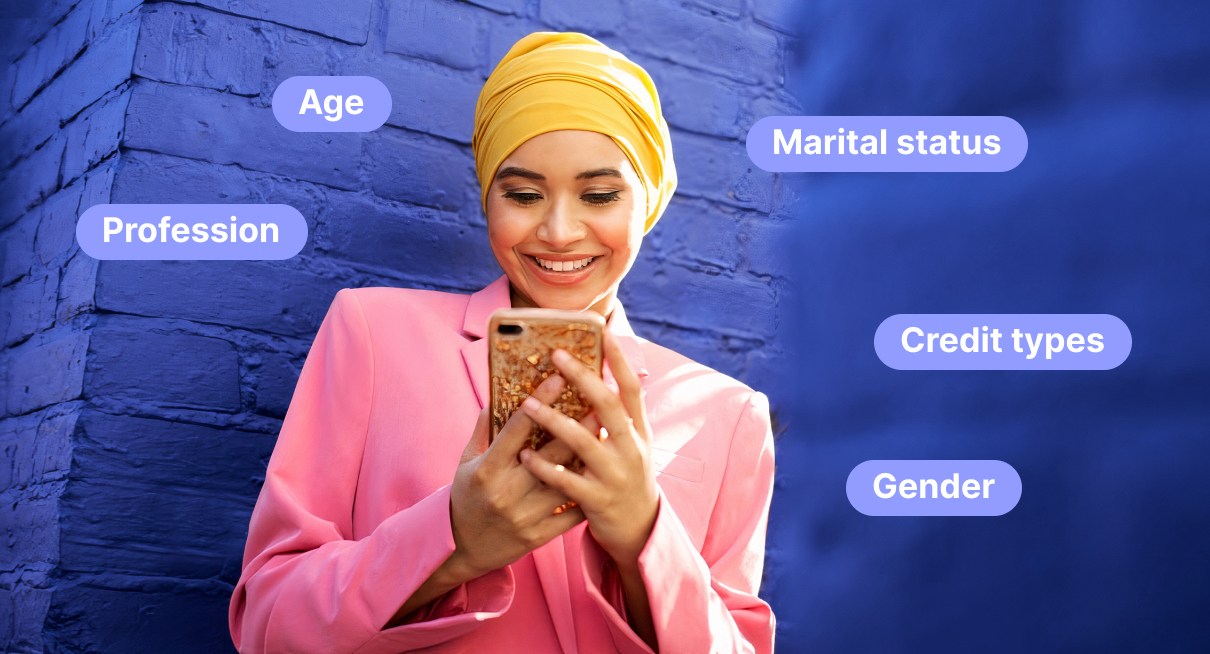
Calculating a client’s credit score is not always a straightforward process. Diversification in financial practices, new approaches to money management, and the wider economic environment mean that traditional methods of customer scoring are a must for lenders. This is especially true when it comes to Millennial and Gen Z clients who may have little to no credit history or have an untraditional relationship with lending. So, what can lending providers do to tailor their services to these new generations of borrowers? Let’s take a look at contemporary customer scoring models and how they can be used in practice.
What Are the Challenges Faced by Millennial and Gen Z Borrowers?
Millennials, a generation born between 1981 and 1996, make up approximately 35% of the US labor market, with that number predicted to rise to 75% by 2025. This gives the generation significant buying power. However, their credit score is lower than that of previous generations, holding at an average of 680 in 2020.
Gen Z is following a similar path. Also known as Post-Millennials, Gen Z is finance-savvy digital natives. Yet, their credit score remains lower than previous generations at an average of around 674 points (2020). While both — Gen Z and Millennials — are classified as ‘good’ credit scores, this isn’t reflective of the entire generation and indicates an area of opportunity in the market as many falls outside this range for a variety of reasons.
Why? This all comes down to how these generations manage their finances. Between 2007 and 2009, the Great Recession hit, impacting Millennials who were just about to enter the labor market, causing financial instability, uncertainty, and a more cautious approach to money. In addition to that, Millennials often found themselves in debt, such as student loans, which had to be paid off as they entered their early careers. This, alongside changing approaches to marriage and family life, with the average Millennial now getting married only after 36. In the 1980s, this number was 23 (women) and 27.8 (men); in 1940, it was 21.5 for women and 24.3 for men, indicating a shift in societal and family values.
At the same time, Gen Z, following in the footsteps of Millennials, took a more cautious approach to finances and family life. For example, it’s estimated that Gen Z has, on average, $14,700 in debt, compared to Millennials at $27,000. A Bankrate survey concurs with this, indicating that 32% of millennials and 23% of Gen Zers have credit card debt higher than emergency savings. Meanwhile, for Baby Boomers (born between 1946 and 1964), this number is just 15%.
This, coupled with negative sentiment in the current economic status quo, has meant that both generations are reevaluating how they manage their finances in a big way. Credit providers need to move with the times and adjust their practices and credit scoring to the new market, not the other way around.
Changing finances of Millennials and Gen Z
- Waiting longer to get married
- Increased student debt
- New approaches to debt vs. savings
- More cautious in terms of finances
- Likely to use technology
How Does Traditional Credit Scoring Work?
To know what needs to be fixed, it’s important to take a step back and explore what’s broken in the first place. To do that, let’s look at the current customer scoring models used by lending providers. These often take into account five key factors:
- Past credit usage. Making up approximately 30% of an individual’s credit score, past credit interactions the amount of credit a person has used compared to how much is available to them. Low usage is a positive indicator, while high usage may reflect poorly. At the same time, zero usage could also prove a worrying factor for lending providers.
- Payment history. Holding a 35% weight in the overall credit score, payment history shows how good an individual was at making credit payments on time. This reliability factor indicates how likely a client is to repay future debt timely.
- Length of credit history. Based on an age policy, the length of credit history makes up 15% of a person’s credit score. Essentially, the longer a person has had an involvement in credit and showed positive outcomes, the higher the score. This means older generations often rank higher on this factor.
- Credit applications. Accounting for 10% of customer scoring ranking, new credit inquiries show how often credit has been applied for by an individual in the past two years. A high number here could mean a risky borrower for the provider.
- Credit types. Also making up 10% of the credit score, the credit mix shows how effectively an individual has combined various credit types — credit cards, student loans, mortgages, etc.
Currently, credit scores are often calculated using available technology via several established credit reporting agencies that utilize FICO scoring. However, they do not give a full picture of an individual, their credit interactions outside these metrics, or the changing needs of a new generation of borrowers. This has led to the rise of new lending technology and growth in the alternative lending sector, expected to be valued at $407.8 billion by 2027.
What Factors Should Providers Consider When Lending in 2022 and Beyond?
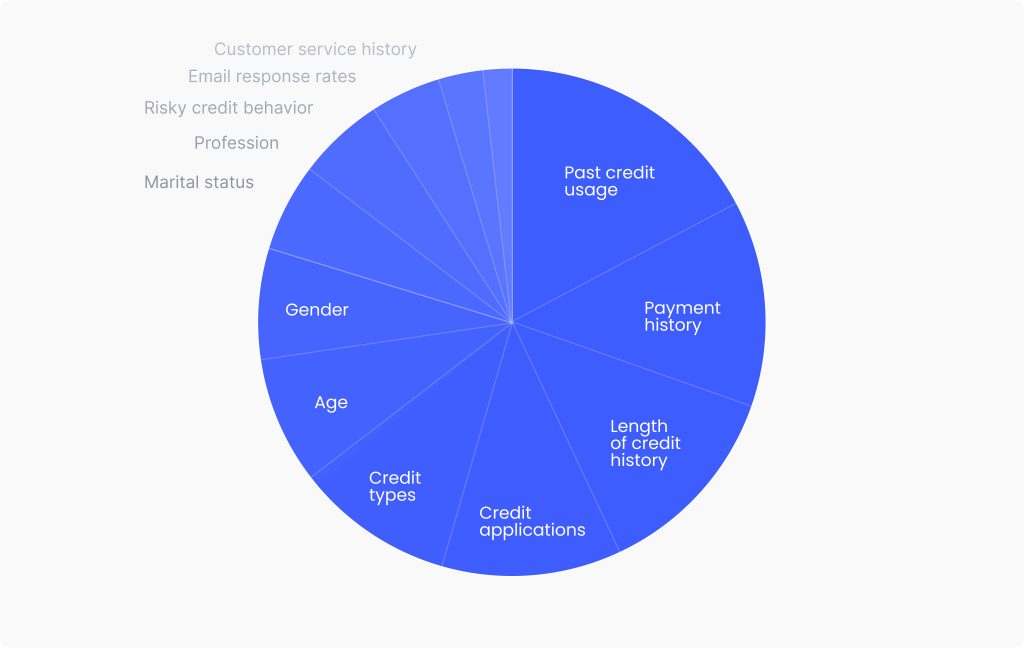
Although current customer scoring models are somewhat effective in predicting how likely an individual is to repay their debt. The ‘tried-and-true’ reasoning doesn’t hold here. With the growth of alternative lending providers and their competitive offering to the market, current credit scoring practices need to change. This can be done by taking into account additional factors, such as:
- Socio-demographic data. It includes the age, gender, marital status, and profession of the individual within the application. Including these details into AI predictive algorithms help to assess the individual more accurately as a whole, not just their current credit score.
- Psychological data. Although still in its early stages, exploring a wider range of data and human interactions can help predict how a person is likely to interact with credit now and in the future. For example, expensive interests could lead to risky credit behavior.
- Behavior data. Emerging analytics tools, such as predictive analytics software, allow lending providers to dive deeper into behavioral data. For example, new tools can take account of past purchase history, frequency, email response rates, customer service history, etc., to show how reliable an individual is and how likely they are to repay any debt.
Combining a wider variety of information and utilizing AI technology, such as GiniMachine’s credit scoring model, can help reduce the challenges felt by Gen Z and Millennial borrowers when accessing credit. At the same time, businesses can remain confident that they are lending to reliable borrowers who are likely to repay their debt in a timely way. Taking into account a wider range of factors democratizes the industry and makes it fairer for borrowers with a less traditional financial history while widening the market for providers.
How Can a Company Onboard New Technologies for Customer Scoring?
Although many lending providers now recognize the need to upgrade their software tools and introduce updated customer scoring models, the majority still find themselves struggling with the best way to do so. Numerous factors hinder decision-making when it comes to onboarding these tools, for example:
- Lack of understanding
- Poor data science experience
- Expectations that are too high
So, what should they do instead? Having worked with clients in the lending industry for numerous years. Here are some of the most effective ways that lenders can get tangible results when onboarding new software:
1. Do your research
Avoid unrealistic expectations and know the tools that are out there at the start of your digital journey. By diving deeper into current industry solutions, you can better understand which technologies are workable and which are just sales pitches. Explore case studies of similar companies and look to alternative lenders to see what they are adopting right now.
2. Work on strategy
Although knowing about the latest technology is helpful. One of the challenges we often observe is businesses wanting to adopt a solution because it is popular, not because it meets their business model — this is often a waste of time and budget. Instead, tailor your technology wish list to fit your business needs. For example, you may consider adjusting your credit scoring model to include customer propensity analysis tools and new AI credit scoring without reworking the entire system or trying to reinvent the wheel.
3. Seek out reliable partners
There are numerous credit scoring technology providers on the market, and while many are reliable, you need to find one that meets your specific business needs. The perfect provider for your business understands your business processes and strategy and has the technology stack and experience to put your plans into action. New AI technology for credit scoring is one smart solution that allows almost out-of-the-box technology to be onboarded with minimum disruption and maximum effect.
Next Steps for Reinventing the Customer Scoring Wheel
Client demographics are changing in big ways for lending businesses. Unlike in the past, credit scoring models are becoming less effective due to diversity in the way new generations manage finances, their financial practices, and competition from alternative lending providers.
By onboarding new technologies, you place your business in the best position to succeed in the changing market and prime it for the future. Taking into account additional factors and utilizing AI technology gives you the best tools on the market for ensuring effective service with the lowest possible business risk.
Ready to start implementing alternate AI-based credit scoring using GiniMachine? Get in touch with the team to know more.