Unlocking the Future of Credit: 11 Questions to GiniMachine’s ML Engineer
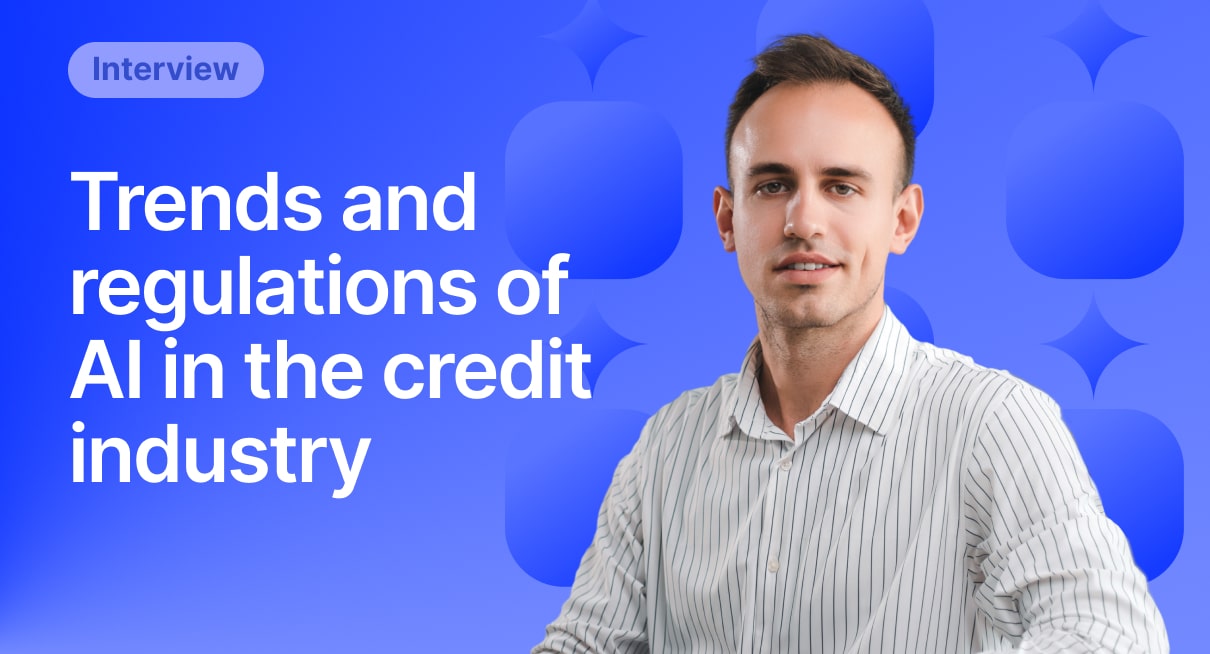
We asked Mark Rudak, ML Product Owner at GiniMachine, 11 questions that will shed light on the transformative role of AI in the credit industry, its limitations, and, of course, how GiniMachine helps financial institutions stay ahead of the curve.
Hi Mark, thank you very much for making the time in your very busy calendar. I know you’re working on some major updates for GiniMachine, so I can imagine it must not have been easy.
Mark: Oh yes, we’re burning the midnight oil and it’s gonna be something! But it’s always a pleasure to share what I’m good at, so let’s get started.
Can’t wait to find out more about it! Ok, let’s get started. My first question is: How does AI currently fit into the credit scoring ecosystem?
Mark: AI is already making a significant impact in the credit scoring ecosystem, and the numbers back it up. According to recent statistics, investments in AI technologies in the financial sector are expected to reach $4.9 billion. That’s a clear indicator of how integral AI has become. Moreover, 77% of financial institutions have either implemented or are planning to implement AI to improve client communication.
But it’s not just about investments and plans; it’s about real-world impact. AI has the potential to increase financial services revenues by 34% and economic growth by 26%. That’s huge! And let’s talk about credit scoring specifically — 80% of executives believe that AI will enhance credit scoring by generating more accurate risk assessments.
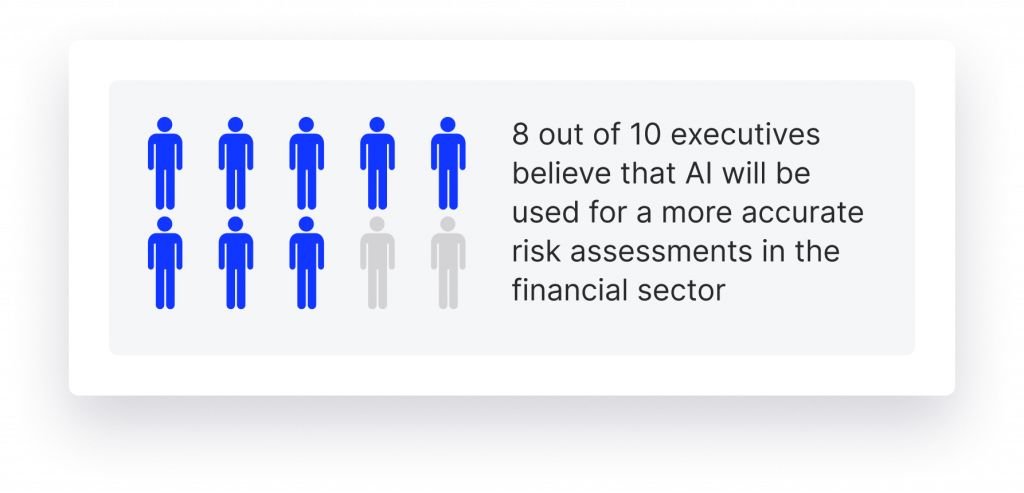
So, as organizations continue to embrace digital transformation, AI is becoming an indispensable tool for making data-driven decisions in credit scoring. It’s not just about automating processes; it’s about making those processes smarter, more efficient, and ultimately, more fair and equitable for everyone involved.
AI versus Traditional Credit Scoring
I see. And what are the limitations of traditional credit scoring methods that AI aims to overcome?
Mark: Traditional credit scoring methods have served us well for a long time, but they do come with their own set of limitations. One of the most glaring issues is the lack of comprehensive human knowledge. Traditional methods often rely on a limited set of variables like income, employment history, and credit history, which can be insufficient for a nuanced understanding of an individual’s creditworthiness.
For example, consider a human agent who is responsible for approving or rejecting loan applications. This agent might have unconscious biases, perhaps favoring applicants from certain backgrounds or with certain types of employment. These biases can lead to unfair or inaccurate credit decisions, which is a significant limitation of the human-centric approach.
Another challenge is the sheer volume of data that needs to be processed, especially as financial markets become more complex and interconnected. Traditional methods often struggle to keep up with the fast-paced changes in both micro and macroeconomic factors, such as fluctuating interest rates or sudden market downturns.
AI offers a compelling solution to these issues. It has the capability for faster estimation compared to human agents, and it can consistently replicate its performance. This means that once an AI model is trained, it can process vast amounts of data in real-time, adapting to new information and providing more accurate and unbiased credit assessments.
So, in essence, AI aims to overcome the limitations of traditional credit scoring by being faster, more adaptable, and free from human bias, thereby offering a more robust and equitable framework for credit assessment.
AI Credit Scoring Trends
Speaking about trends. What are some emerging trends in AI that you think will significantly impact credit scoring?
Mark: Emerging trends in AI are like the new flavors of the month, but some of them are here to stay and really shake things up. One trend I’m particularly excited about is the use of alternative data sources in credit scoring.
You see, traditional credit scoring is like judging a book by its cover. You look at income, existing loans, and credit history, which is fine, but it’s not the whole story. Now, imagine if you could read a few chapters of the book before making a judgment. That’s what alternative data sources allow us to do.
Learn More About Online Loan Solution
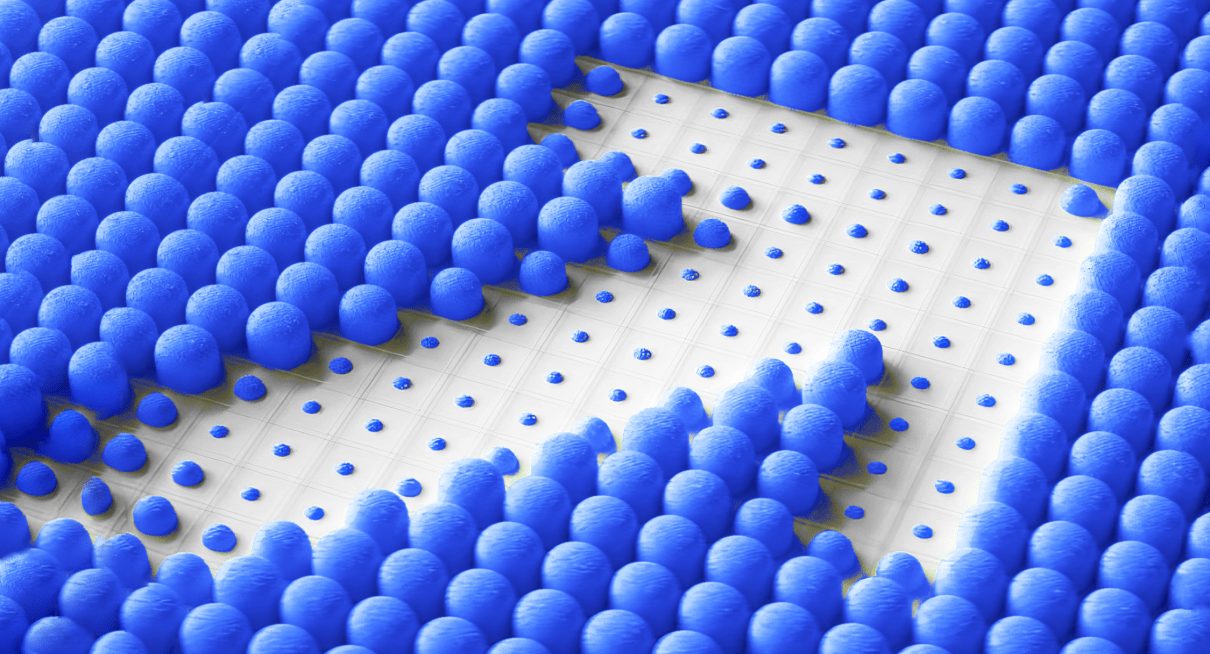
For instance, we’re starting to look at telecom records. Believe it or not, how you manage your phone bill can say a lot about how you’ll manage a loan. Then there’s behavioral patterns, like your online shopping habits or even how often you log into your bank account. It might sound a bit ‘Big Brother,’ but this data can be incredibly insightful.
And let’s not forget transactional data. I’ve been working on models that can analyze your day-to-day spending to predict your financial stability. It’s like having a financial health check-up every time you swipe your card.
So, the future of credit scoring is not just about numbers; it’s about understanding behavior.
And what about a more precise time frame? Let’s say, the next 5-10 years?
Mark: So, looking at the next 5-10 years, we’re going to see AI not just dipping its toes but really diving deep into the credit scoring pool. It’s like we’re moving from black-and-white TV to 4K resolution in how we assess creditworthiness.
One trend I’m super excited about is the move towards recommendation systems and behavioral scoring. Imagine a system that knows you so well, it can predict your financial behavior based on how you interact with your money today, last month, or even last year. It’s like Netflix recommending your next binge-watch, but for loans and credit!
For example, I’ve been working on models that can analyze how you manage multiple credit lines. Do you pay off the high-interest ones first? Do you spread your payments evenly? This kind of nuanced behavior can give us incredible insights into how financially savvy you are.
And let’s not forget about the data. We’re talking about petabytes of data here—everything from your spending habits to how often you check your credit score. AI models will be like master chefs, taking all these ingredients and cooking up a credit score that’s far more reflective of your true financial behavior.
So, in the next 5-10 years, I expect we’ll see a lot more personalization and precision in credit scoring, thanks to AI. It’s going to be a transformative decade, to say the least.
Benefits of AI in Credit Scoring
Interesting! It all sounds very promising, yet we see that not many financial institutions have adopted AI. Could you please highlight the key benefits of using AI in credit scoring for credit portfolio owners?
Mark: First off, speed and scale. You can cover so much more ground in less time. We’re talking about processing hundreds, if not thousands, of credit applications in the time it would take a human team to go through a handful. It’s like having an army of analysts working around the clock, but without the coffee breaks!
Next, optimization. AI doesn’t just rubber-stamp applications; it’s like a seasoned chess player, always thinking several moves ahead. For example, I’ve worked on models that can predict the likelihood of a loan going bad in different economic scenarios—like a recession or a housing market crash. This allows you to manage your non-performing loan (NPL) rate more effectively, finding that sweet spot between risk and profitability.
So, to sum it up, adopting AI in credit scoring is like upgrading from a bicycle to a sports car. You go faster, you cover more ground, and you do it all with a level of precision that was previously unimaginable.
Challenges of AI Credit Scoring
What challenges do companies face when implementing AI-based credit scoring models?
Mark: One word – interpretability. Imagine you’ve got this super-smart AI model that’s like a genius mathematician. It can solve complex problems in seconds, but when you ask it to explain how it got the answer, it starts speaking in equations and algorithms that go over most people’s heads.
So, the challenge is making sure we can understand why the AI is making certain decisions. It’s like having a brilliant doctor who can diagnose an illness instantly but struggles to explain it in plain English. We need that explanation, especially when it comes to things like loans and credit, where people’s lives are affected.
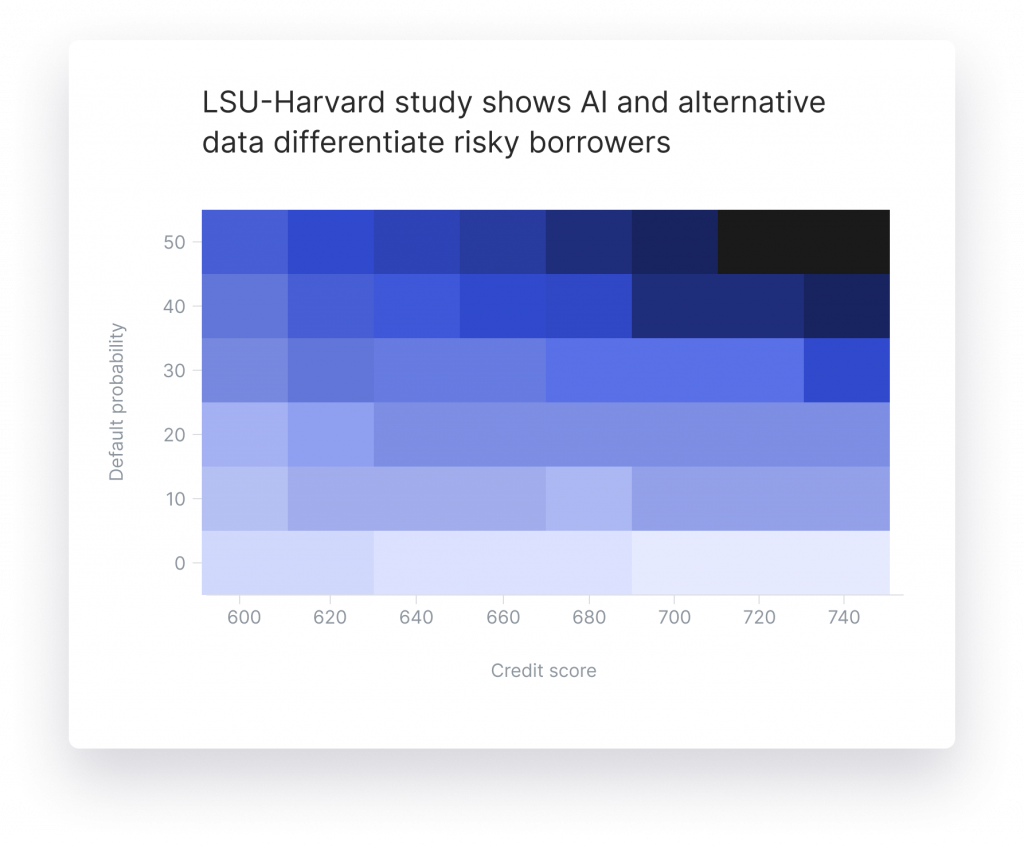
And let’s not forget about the rules and regulations. The financial industry is like a maze of laws and guidelines, right? So, we have to make sure our AI models are not just smart but also law-abiding citizens, so to speak. We need to address concerns about fairness and transparency, making sure we’re not accidentally discriminating against anyone.
AI Scoring and Compliance
How does GiniMachine ensure compliance with regulations like GDPR or the Fair Credit Reporting Act when using AI for credit scoring?
Mark: Compliance is a big deal, especially with laws like GDPR in Europe and the Fair Credit Reporting Act in the U.S. So, how does GiniMachine tackle this? Well, think of it as a really diligent student who not only does the homework but also shows all the work in the margins.
For example, we have this cool feature called ‘attribute importance analysis.’ Imagine you’re trying to figure out why you didn’t get that loan. This feature will break it down for you, saying something like, ‘Hey, your income was a big factor, but your credit history? Not so much.’ It’s like getting a peek behind the curtain to see how the magic trick is done.
We also use pivot tables for attribute statistics. Let’s say regulators want to know how we’re assessing risk. We can show them these tables that lay out how different attributes like age, income, or even zip code play into our decision-making. It’s like showing your math in a calculus exam.
And then there’s scoring result interpretation. This is where we translate the AI’s complex reasoning into plain English. So, instead of saying, ‘Applicant rejected due to Algorithm X-256,’ it’ll say, ‘The applicant has too many active loans to be considered low-risk.’
So, in a nutshell, GiniMachine is all about being transparent and accountable, making sure we’re ticking all the boxes when it comes to regulations.
GiniMachine for Default Managamant
How does AI help in reducing the risk of bad debt?
Mark: That’s an excellent question, and it addresses a critical concern in the lending industry—bad debt. AI acts as an advanced analytical tool, sifting through extensive data to identify risk patterns.
Let’s say we had a borrower who was 35 years old, had an income of $1000, 5 kids, and 10 active loans, and guess what? He had a poor payment history. Now, when a new application comes in with the same or similar characteristics, the AI model flags it, saying, ‘Hey, watch out! This one’s likely to be a poor payer too.’
This is a complex pattern that the model has learned from past credit history, and it applies this knowledge to new applications. And this is just one of potentially thousands, or even more, intricate patterns that the model identifies.
So, by doing this, we can make more informed decisions, which helps us avoid bad debt.
Sounds like a game-changer. For credit portfolio owners who have been in the industry for decades, what would be the most compelling reason to transition to AI-based credit scoring now?
Mark: It is indeed a game-changer. Now, for those who’ve been in the credit industry for ages, you might wonder, ‘Why fix what isn’t broken?’ But here’s the thing: AI isn’t about fixing; it’s about enhancing.
Imagine you’ve been using the same old map for years, and it’s worked fine. But now, someone offers you a GPS that not only shows you the route but also tells you about traffic jams, roadwork, and even finds the best parking spots. That’s what AI does for credit scoring.
For example, let’s say you’ve been rejecting applications based on a set of criteria you’ve trusted for years. AI can go back and analyze those rejected applica
tions to see if some of them might have actually been good bets. Maybe you rejected someone five years ago who’s now a CEO of a successful startup. AI can catch that and help you refine your criteria.
It can also predict future scenarios. Like, ‘Hey, if you keep using your current model, you might miss out on $2 million in profits next year.’ It’s like having a financial crystal ball!
So, for seasoned credit portfolio owners, the most compelling reason to switch to AI is that it gives you this 360-degree view of your operations. It helps you identify growth areas, estimate what you might be leaving on the table, and provides actionable insights to really drive your business forward.
Learn More About GiniMachine
AI Credit Scoring KPIs
What are the key metrics that GiniMachine uses to evaluate the performance of its AI-based credit scoring models?
Mark: The bread and butter of any data scientist. At GiniMachine, we’ve got a whole smorgasbord of metrics to make sure our AI-based credit scoring models are top-notch. But it’s not just about the techy stuff; we also focus on business-oriented metrics that speak the language of credit portfolio owners.
Firstly, let’s talk about the Gini Index. It’s a metric that ranges between -1 and 1, and it’s super useful for understanding model quality. The cool thing is, it’s not just a number; it actually tells you the collective impact of all the factors we consider, like income, credit history, and so on. It’s like having a dashboard that shows you how each parameter influences the final decision.
Then we have ROC AUC, which stands for Receiver Operating Characteristic Area Under the Curve. It’s a mouthful, I know, but it’s a fantastic way to measure the overall performance of our models. It ranges from 0 to 1, and the closer to 1, the better the model is at distinguishing between good and bad credit risks.
And don’t forget the K-S Score, short for Kolmogorov-Smirnov. This metric helps us see how well our model’s predicted probabilities align with real-world outcomes. It’s like a reality check for our AI, making sure it’s not living in a fantasy world.
We also look at other metrics like Recall, which focuses on how many actual positive cases we caught; Precision, which is about how many of the caught cases were actually positive; and False Omission, which helps us understand the cases we might have missed.
So, in a nutshell, GiniMachine serves as a credit scoring software that offers a 360-degree view of model performance, making sure we’re aligned with both the geeky technical stuff and the real-world business needs.
Handling Extreme Events
How does your AI model account for “black swan” events, like economic downturns or pandemics, in its risk assessment?
Mark: Yeah, this question is especially relevant nowadays. ‘Black swan’ events are rare but highly impactful occurrences. Our AI models are specifically designed to be resilient and adaptive in the face of such unpredictable scenarios.
The models are trained on extensive historical data sets that capture a wide range of economic conditions, including recessions, market crashes, and even global pandemics. This allows the AI to identify hidden trends and patterns that may not be immediately apparent but can be indicative of larger systemic risks.
For instance, during the training phase, the models may encounter data from periods of economic instability and learn how various factors interacted during those times. This learning equips the model to make more nuanced risk assessments, even in the face of unprecedented events. It’s akin to a stress test for the model, ensuring that it can adapt its risk assessment algorithms to account for extreme conditions.
I see. We’ve covered a lot of ground today, from the transformative role of AI in credit scoring to its limitations and the future trends that are set to revolutionize the industry. For our readers who are interested in diving deeper into the topics we’ve discussed, I highly recommend checking out the articles and videos we’ve linked throughout this interview.
Mark: Thank you very much! It’s always a pleasure.
Don’t hesitate to reach out to us to make credit scoring smarter, faster, and more equitable. Contact GiniMachine today and take the first step in revolutionizing your credit portfolio.