Turning Data into Dollars: A Comprehensive Guide to Optimizing Your Credit Department with GiniMachine
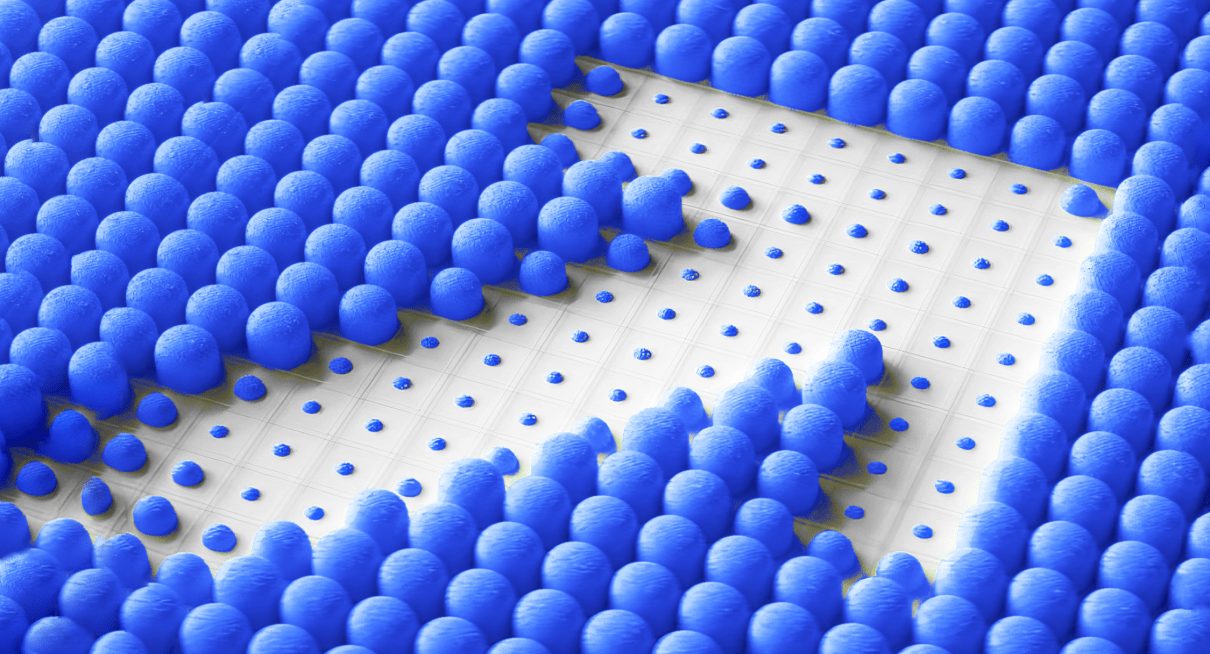
Identifying and correcting errors within your credit department’s operations is key to maintaining a competitive edge. This article provides valuable insights and guidelines that will assist you in enhancing the accuracy and efficiency of your credit decision-making process.
With a credit scoring platform like GiniMachine, you can optimize your credit approval process, improve risk analysis, and ultimately increase your profitability. However, to realize these benefits, a well-prepared and clean dataset is essential.
This article serves as your guide to leveraging GiniMachine for optimizing credit operations. You’ll learn to prepare and upload your dataset into GiniMachine, evaluate predictive models, and understand the significant parameters in the credit approval process. Additionally, GiniMachine’s capability to streamline the analysis of language data not only enhances accuracy but also minimizes NLP-related errors. This, in turn, reduces risks and saves substantial resources, effectively optimizing your credit department’s functioning.
Polishing Your Data to Get the Perfect Data Set
In preparing your dataset for the GiniMachine AI/ML scoring model, meticulous data cleaning is paramount. This involves identifying and correcting issues with your data. The objective is to remove unfixed elements that can introduce inaccuracies, disrupt algorithms, and skew your results. With cleaned data, the dataset becomes streamlined, paving the way for meaningful insights and reliable predictions.
To prepare your dataset, follow these nine steps:
- Clear formatting: Rid your dataset of hidden symbols and unnecessary spaces that could muddle the data interpretation.
- Remove duplicates: Duplicates can distort your analysis and lead to skewed results. Ensure to remove any repetitive entries.
- Remove irrelevant data: Strip out URLs, HTML tags, tracking codes, and superfluous blank spaces. For creditworthiness analysis, it’s also advisable to eliminate personal data.
- Use numbers for dates: AI and machine learning work best with numbers. So, transform date formats into numerical values, e.g., replacing “June 29th, 1990” with “06/29/1990”. Maintain uniformity in currencies, measurements, and country names.
- Standardize capitalization: Consistency in capitalization is important for data interpretation. Adopt a single standard throughout your dataset.
- Eliminate errors: Your dataset should be free from typos, misspelled words, or punctuation errors.
- One language at a time: NLP models work best with one language. Stick to one language per dataset.
- Meet the minimum requirements: For a quality model, your dataset should have a minimum of 1000 records. These records should provide a diverse range of data, and GiniMachine supports file formats such as xlsx, csv, and xls.
- Include recommended attributes: Enrich your dataset with diverse attributes such as social and demographic data, geographical data, employment data, credit history data, lender-calculated parameters, and alternative data.
Following these steps will ensure your dataset is ready and optimized for the GiniMachine AI/ML scoring model, enabling accurate and insightful predictions to drive your business growth.
Next, you’ll proceed to upload your dataset. With GiniMachine, it’s absolutely straightforward – you merely need to drag and drop it into the system.
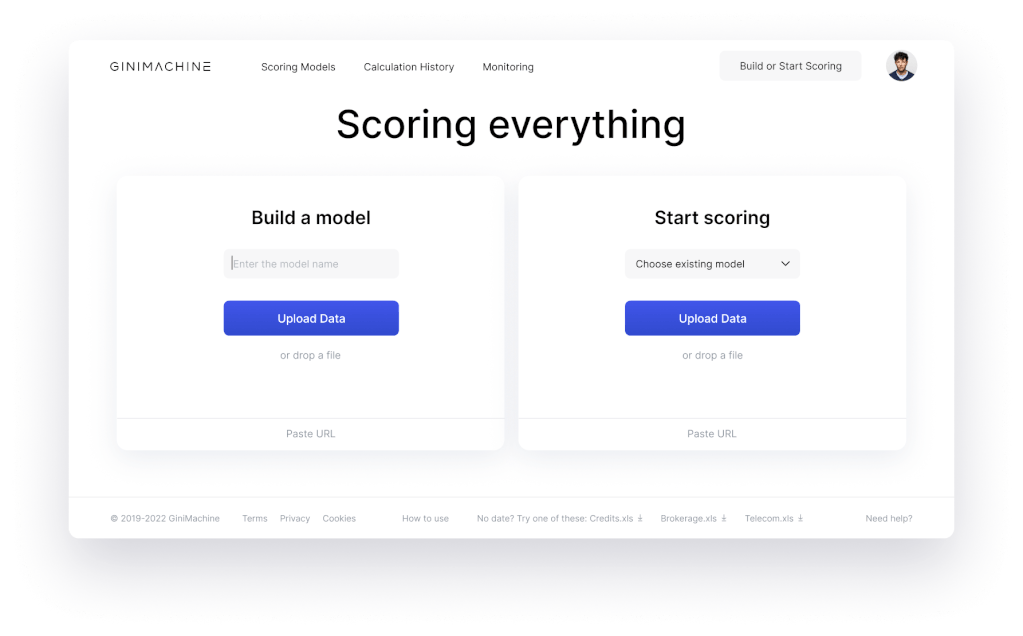
Now, let’s delve into the parameters that can help pinpoint areas for improvement within your credit department’s operations.
Learn More About How to Optimize Your Credit Department
Enhancing Your Credit Department Performance: A Deep Dive into Parameters, Their Weights, and Numerical Values
After preparing and uploading your dataset to GiniMachine, you’re only halfway there. The next significant step is evaluating the performance of the predictive model. This process ensures the system’s effectiveness and helps you find potential areas of optimization. To do so, you need to focus on three primary aspects: the parameters used, the weight of these parameters, and their numerical values.
1. Evaluating Parameters
To start, take a close look at the parameters your credit department has relied upon. Are these parameters representative enough for making sound decisions? Could some be unnecessary, or perhaps are some critical ones missing?
Reviewing your parameters is crucial in this step. You may realize that some parameters, though they seem important, might not be as crucial in the grand scheme of things. For instance, if a parameter like ‘previous credit inquiries’ has a minimal weight in decision-making and its collection process is time-consuming, it might be worth reconsidering its inclusion. Streamlining your parameters can lead to significant time savings and enhance the effectiveness of your predictive model.
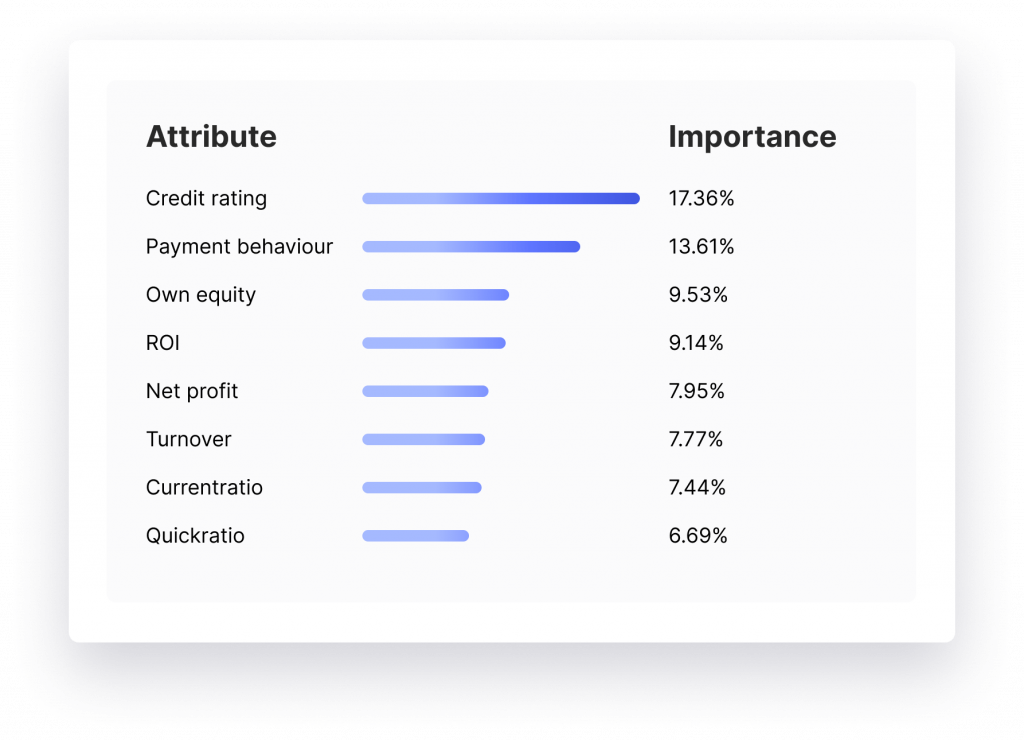
2. Parameter Weight
Understanding the weight or the significance of each parameter in the overall decision-making process is crucial. One example is a client who heavily relied on credit rating when assessing the creditworthiness of a commercial loan. In doing so, they might have missed out on promising young companies that lacked an established credit rating but had stable income and profit indicators. It’s crucial to strike a balance in how much weight is given to each parameter to avoid such scenarios.
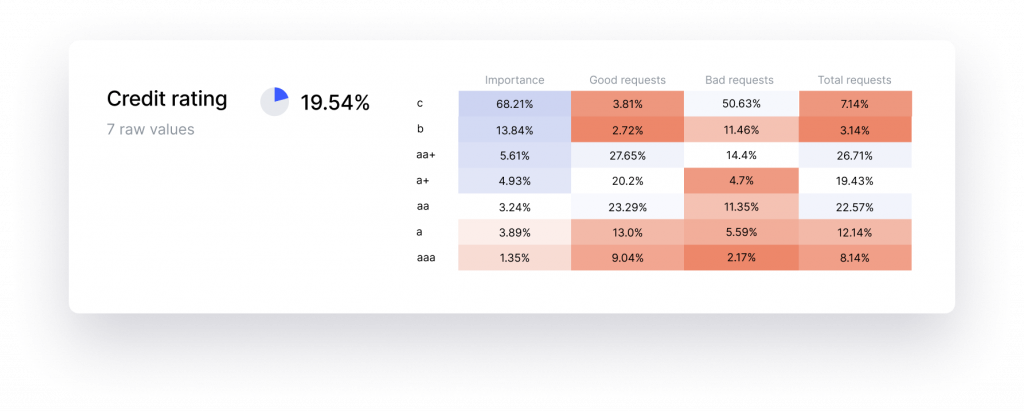
3. Numerical Values of the Parameters
Lastly, pay close attention to the numerical values assigned to the parameters. For instance, if the turnover of a company is a primary factor in your decision-making process, it might inadvertently exclude potentially profitable borrowers. Remember, a stringent filter can limit the growth of your loan portfolio and negatively impact your bottom line.
By taking the time to study these parameters and their assigned numerical values, you can improve the performance of your department significantly. The goal here is to identify systematic errors and biases that might occur due to human prejudice or error.
Wrapping Up
Evaluating the performance of your predictive model is not a one-time process but a continuous one. You should regularly revisit these steps and adjust them based on the evolving market conditions and your organization’s needs. Doing so will ensure that you are always optimizing your credit scoring process, reducing risk, and increasing profitability.
Are you ready to take the first step towards optimizing and empowering your credit department with AI and ML? Let GiniMachine help guide your credit department towards more informed decision-making, lower NLP and higher profitability. With our advanced AI/ML scoring model, your business can significantly improve its risk analysis and credit approval process.
Don’t hesitate to reach out to us for a consultation. We’re here to make the process easier, simpler, and more accurate. Contact GiniMachine today and see the difference that a data-driven approach can make in your credit operations.