How to Get Rid of Human Bias in Machine Learning
Contrary to common assumptions, the introduction of technology does not automatically eliminate biases in decision-making. However, AI scoring models have the potential to reduce biases if implemented properly. Let’s see how to do it right.
- Define the AI’s objective: Clearly articulate the specific questions and challenges you want the AI model to address. Translate subjective inquiries into quantitative parameters that ensure fairness in decision-making.
- Consider relevant factors: Identify key factors that contribute to creditworthiness and ensure they align with your organization’s criteria. Examples may include a client’s expendable income, loan quantity, profit margins, and additional requirements.
- Emphasize fairness: While framing algorithms, it is crucial to avoid intentionally or unintentionally creating biased models that may lead to discriminatory practices, such as predatory lending. Ensure that the algorithms operate within ethical boundaries and promote fairness.
- Acquire representative data: Evaluate the sources from which you gather information about lenders and potential credit partners. Ensure that the data is representative of your target audience to avoid inherent biases. Relying too heavily on historical lending decisions can perpetuate past biases, so it’s important to periodically test and review the results.
- Conduct thorough data preparation: Data plays a pivotal role in AI credit scoring models. Properly preparing and cleaning the data is essential for accurate and unbiased outcomes. Validate that the data you have is relevant, reliable, and understood correctly by the algorithm. Address issues like unrepresentative data samples and biases attributed to factors such as gender or geographic location.
How GiniMachine Can Help
Now, let’s delve into real-life examples that demonstrate how GiniMachine’s capabilities empower companies to identify and address biases, leading to improved lending decision-making. These instances highlight the practical application and effectiveness of GiniMachine in creating fairer and more inclusive lending practices.
Challenge 1: Non-performing loans (NPL) are rising without a clear understanding of the underlying reasons
Resolution: Develop a model and upload the most recent credit applications to compare approval rates using your existing method versus the rates achievable with Gini. If your NPL is elevated, it is advisable to reassess your loan approval process. Take note of the factors and their corresponding weights recommended by our algorithm.
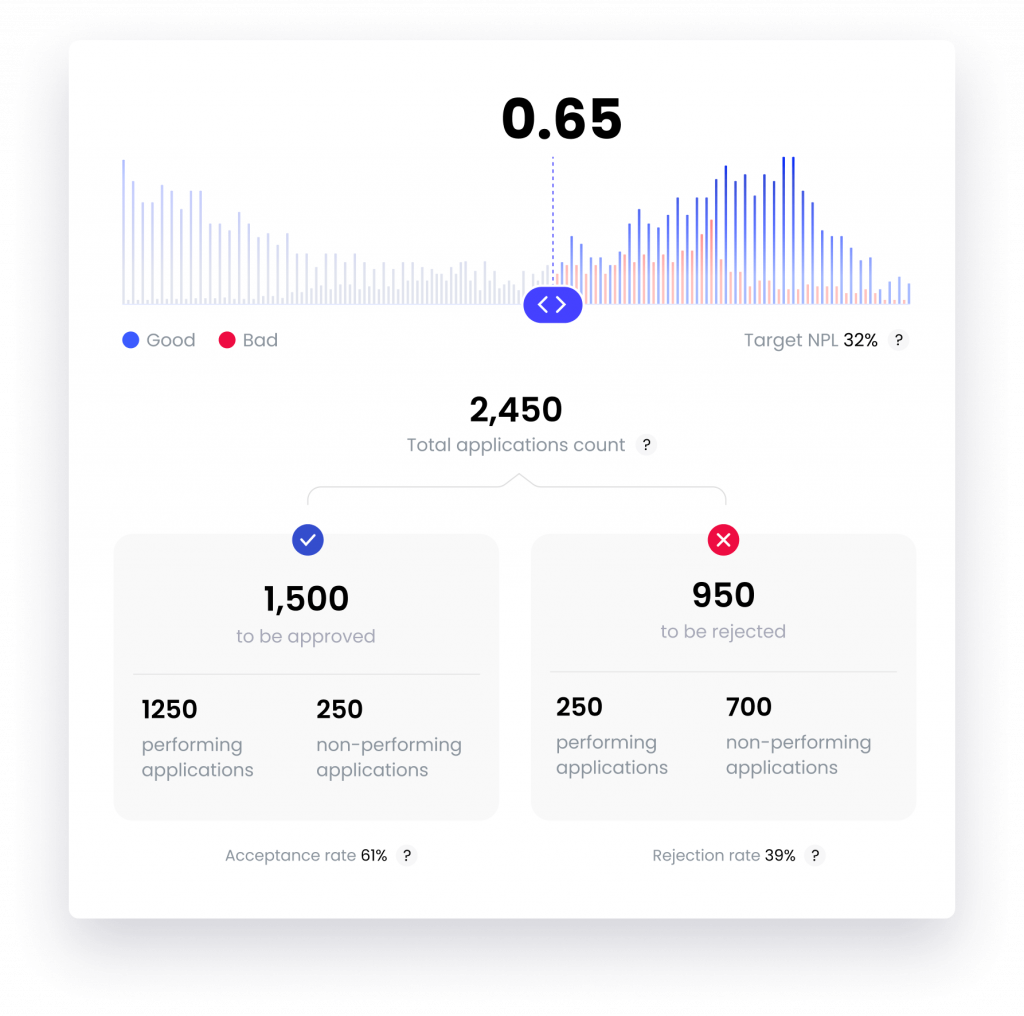
Challenge 2: Bias and identifying growth opportunities
Resolution: Train the model, incorporate supplementary data, and assess the factors influencing decision-making.
One of our clients faced a challenge where loan applications from individuals with criminal records were automatically rejected by a specific department, regardless of their income or the timing of the offense. However, upon closer examination of these applications, it was discovered that many of these applicants were indeed creditworthy. This case was identified through an evaluation of the existing model’s performance for our client. Ultimately, Gini played a pivotal role in revising the credit policy for this particular group, allowing for greater inclusivity and growth opportunities.