How to Prepare a Perfect Dataset for AI/ML Scoring Model of GiniMachine
Does the term ‘scrubbing’ ring a bell? This is the same term used for ‘data cleaning’, and these two are crucial when it comes to preparing your dataset for future decision-making.
In this guide, we’ll start with the basics and explain the importance of cleaning data in analytics, explain why data cleaning is important, and provide a step-by-step guide on how to prepare data for machine learning. Let’s get started.
Data cleaning, scrubbing, cleansing… Oh my!
All of these terms refer to the process of identifying and correcting issues with data, a critical step in predictive analytics in banking. Unfixed data elements need to be removed from the dataset for several reasons:
- Those elements can introduce inaccuracies and errors into the analysis or modeling process. These elements might contain missing values, outliers, or inconsistent or incorrect information that can significantly impact the reliability and validity of the results.
- Such elements can also disrupt the analysis or modeling algorithms. Including unfixable data elements can lead to biased or misleading results, affecting the overall quality of the analysis or predictions.
- Poor-quality data can cost businesses up to $14.2 million every year.
GiniMachine is an advanced tool backed by powerful AI algorithms, but data validation and cleaning are still necessary to build a reliable predictive model.
In short, when your data is cleaned, the dataset becomes more streamlined and focused, improving the overall quality of the data for decision-making or modeling purposes. It ensures that the remaining data is accurate, consistent, and representative, allowing for more reliable and meaningful insights or predictions to be derived from the dataset.
How to Prepare The Ideal Dataset for GiniMachine: A 9-Step Guide
The AI-powered decision-making process relies on human intervention to prepare the dataset. Despite AI’s intelligence, humans play a vital role in structuring and making the data easily understandable for AI systems. By properly preparing the data, businesses can streamline their processes and foster growth. In this section, we’ll provide a step-by-step guide to help you prepare your data effectively, enabling GiniMachine for informed decision-making and propelling your business forward.
Step #1 Clear Formatting
This step is straightforward. Just ensure that your dataset is free from hidden symbols and unnecessary spaces, as they can cause confusion and lead to inaccurate results.
Step #2 Remove Duplicates
It’s as simple as that. Ensure that there are no duplicate entries in your dataset. If duplicates exist, they can compromise the quality of visual representation and analysis.
Step #3 Remove Irrelevant Data
Consider removing URLs, HTML tags, tracking codes, and unnecessary blank spaces in the text. Also, when analyzing creditworthiness, it’s reasonable to remove personal data like names and email addresses.
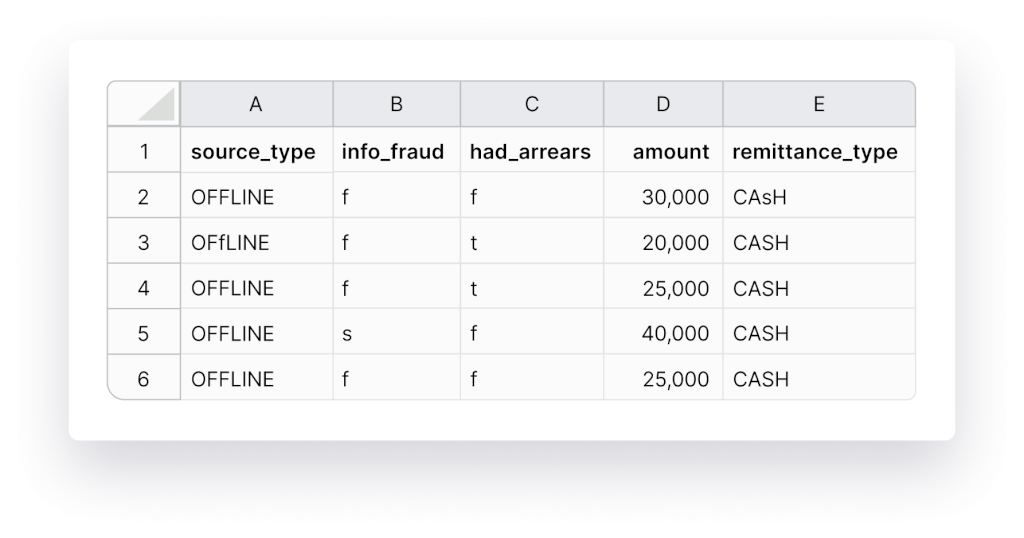
Step #4 Use Numbers For Dates
AI and machine learning thrive on numbers, so let’s simplify their task. Ensure consistency by replacing “June 29th, 1990” with “06/29/1990”. Also, maintain uniformity in currencies and measurements. Convert fields with amounts in US dollars and Euros to the same currency. Similarly, standardize country names, such as consistently using “US” or “United States of America”.
Step #5 Standardize Capitalization
Consistency matters not just for AI but also for us humans. “Bill” and “bill” have different meanings, so ensure your data follows a single capitalization standard throughout.
Step #6 Eliminate Errors
The dataset is not the place for typos, misspelled words, or punctuation errors. Accuracy is essential.
Step #7 One Language At a Time
Most NLP models are designed to handle one language at a time, that’s why it’s essential to stick to one language in a particular dataset.
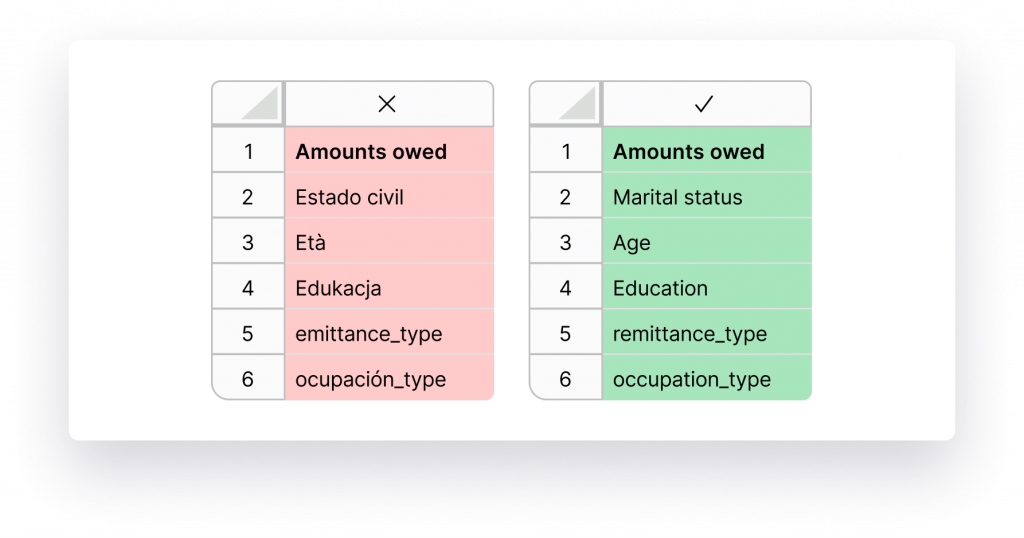
Step #8 Meet The Minimum Requirements
To ensure a quality model, it is recommended to have a minimum of 1000 records in your dataset. These records should include a diverse range of data, such as 870 good loans and 130 bad loans, for example.
GiniMachine supports file formats such as xlsx, csv, and xls. The cells within the files can contain text, numbers, or dates.
Step #9 Include Recommended Attributes
The list of recommended attributes includes but it not limited to:
- Social and demographic data about applicants (gender, age, education, marital status, number of children)
- Geographical data (residence, country, city)
- Employment data (profession, present employment duration, total work experience)
- Data about employers (industry, company size, location: city, region)
- Credit history data (total amount of debt, number of open contracts, total number of delinquencies)
- Parameters calculated by the lender (debt-to-income ratio, payment-to-income ratio , total loan debt)
- Alternative data (behavioral data, payment data from telecom and utility service providers)
Note: it’s recommended to include a maximum of 50 values.
No Time for Data Cleaning? Here’s The List of Tools to Help You Out!
A lack of time is totally understandable but it doesn’t make data cleaning unnecessary. At GiniMachine, we’re constantly using various tools to speed up the process, and these four are our favorites:
- Tableau Prep is best suited for companies that prioritize data visualization and analysis.
- Tibco Clarity is ideal for organizations that require robust data integration and governance solutions. It helps streamline data processes, ensuring data accuracy, consistency, and compliance with data governance policies.
- Informatica Cloud Data Quality is a cloud-based data quality tool that focuses on improving data accuracy, completeness, and consistency.
- Oracle Enterprise Data Quality caters to organizations using Oracle’s ecosystem and offers data profiling, cleansing, and matching capabilities. Oracle Enterprise Data Quality ensures high-quality data for reliable business insights and efficient operations within Oracle environments.
To Sum Up
Data cleaning and the prep work may appear daunting, but it serves as the essential groundwork for making informed and unbiased decisions. In this article, we have outlined 9 fundamental steps of this critical process. While there may be additional steps, GiniMachine is an advanced tool that can handle much of the work for you. Powered by robust AI/ML algorithms, GiniMachine simplifies and automates the data cleaning process, using the cleaned dataset for informed decision-making.