Why You Need Predictive Analytics in Manufacturing for Your Business
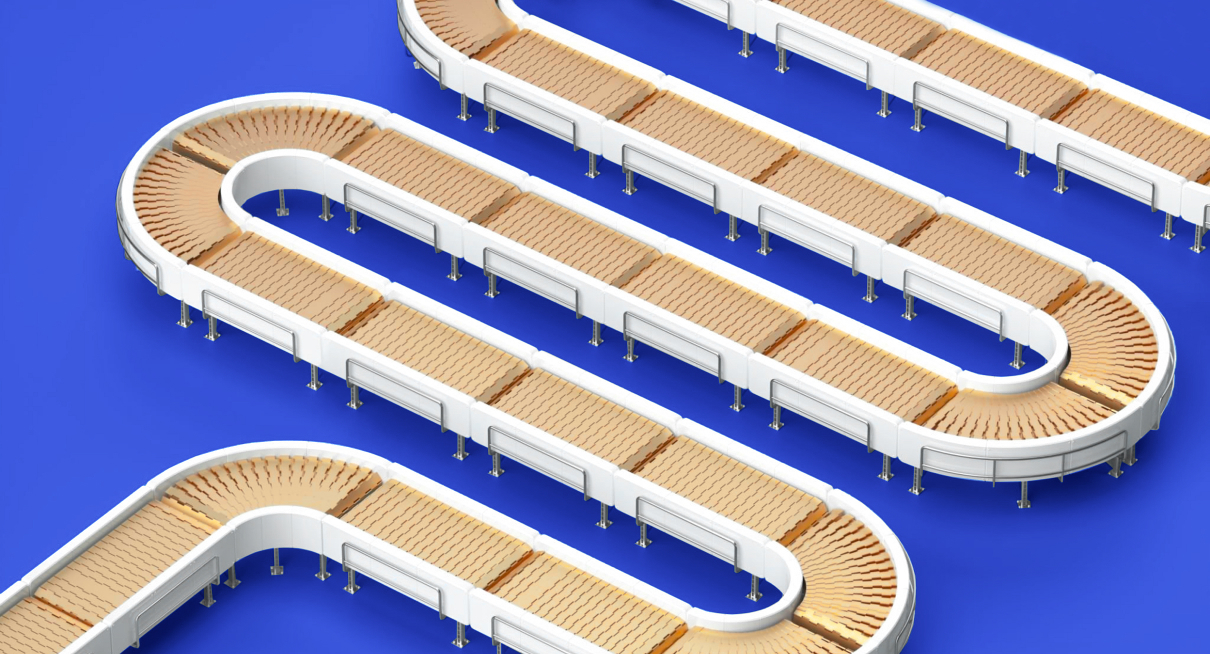
Is it possible to predict the future? Predictive analytics argues that it just might be. A growing trend across many industries, and with a market value of $10.5 billion in 2021, a CAGR of 21.7%, and a growth rate expected to reach $28.1 billion by 2016, predictive analytics is showing no signs of slowing down any time soon.
Driven by an uptick in digital technology usage and as a result of an increasingly digital society in the wake of the coronavirus crisis, more data is available for analysis now than ever before. The manufacturing industry is no exception. Manufacturing data analytics allows companies to plan ahead more effectively, and experts suggest this is one of the keys to success.
Smart analytics delivers the flexibility and agility to ensure the right processes are happening at the correct time, but how can a business engage predictive analytics in manufacturing to get results and why should they invest in this type of solution in the first place? Let’s explore.
What Is Predictive Analytics in Manufacturing?
Before we dive into the use cases and reasons, let’s go back and take a look at just what predictive analytics (manufacturing) is exactly?
Predictive analytics in manufacturing is artificial intelligence technology (AI) and machine learning (ML) tools that allow a business to monitor, analyze and optimize its systems and processes. Manufacturing data analytics:
- Identifies trends in the manufacturing process and market trends
- Detects and predicts future issues that may occur, such as machinery that needs servicing, supply chain issues, and more
- Suggests upgrades for tools and software to optimize the process
- Helps ensure cost-effectiveness, better organizational finances, and reduce losses which can impact the overall company budget
Some data analytics in manufacturing also engages other technologies, such as the Internet of Things, which can monitor the condition of equipment in the manufacturing process as well as supply chain progress.
It can take some time for data to be collected and be valuable to a business. Too little data can affect the results and deliver false analytics, that’s why it’s vital that any company seeking to engage in predictive analytics tools for manufacturing views the upgrade as a process not a purchase.
Manufacturing Analytics Use Cases: Where Can It Be Employed?
Predictive analytics in manufacturing has a variety of use cases. By onboarding the right software, your business can unlock a wealth of data that can empower your company to new heights. These are some of the most beneficial applications of predictive analytics in action:
Improving workplace metrics
From making hiring decisions to ensuring you have enough staff to adequately operate performance analytics and KPIs to productivity and safety management, predictive analytics can help. For example, by monitoring staff performance, you can establish where teams may be lagging and react quickly to create smart solutions. Alternatively, you may notice incident report trends that indicate a safety issue. Predictive analytics can help you tackle this problem before it becomes a major issue.
Optimizing the manufacturing process
Experiencing bottlenecks but don’t know quite where they’re coming from? Struggling to scale when needed? By onboarding predictive analytics, you can evaluate your current manufacturing process data to solve future issues. For example, say you notice that you’re experiencing downtime at certain points throughout the month. By utilizing analytics, you can establish why this is happening, when it is likely to happen again and plan for this eventuality to cut downtime and reduce economic loss.
Predicting demand and optimizing supply
Past behavior may not predict future behavior but having an eye (or some handy AI tech) on market trends can help you stay ahead of the game. By monitoring market supply and demand analytics, you can evaluate potential future needs and adjust your business accordingly. For example, during the coronavirus crisis, many alcoholic drinks suppliers had to quickly adjust their business plans to produce high-demand hand-sanitizers. Predictive analytics in manufacturing puts you ahead of the game.
Analyzing financial and credit risk
Businesses may find themselves taking on credit in order to optimize processes or upgrade their technology. But how to assess if the risk is worth it? By analyzing past statistics and market trends, predictive analytics can help establish whether a potential manufacturing investment is a solid investment or a risky one. Smart AI modules can dive into available data and reveal information that may not be visible through traditional methods.
How Does Data Analytics in Manufacturing Work?
Now we know some of the use cases where data analytics in manufacturing are effective, let’s take a look at how this process works in practice.
Step 1: Data Collection
Before you can start analyzing your data, the first step is data collection. At this stage you need to identify which specific data you will need. For example, you need to identify:
- Which data is most valuable to your manufacturing process?
- How will knowing this information help improve processes?
- What can predictive analytics analyze so that you can get results?
Initially, it may appear that every piece of information is valuable and essential to your process, and indeed it may be. However, in order to get the most from your predictive analytics tools for manufacturing, you’ll need to choose carefully which processes you’d like to improve first. This way you’ll be better placed to monitor the results and decide on future steps.
Next, it’s time to collect and collate all the data relevant and move on to step two.
Step 2: Start preparing your data for analysis
Once you’ve completed step one and gathered your data, next comes the hard part—preparing your data for analysis. Often raw data is messy and can be misleading. At this stage you should format and organize your data in a way that is understandable for AI technology.
At this stage you may encounter such challenges, such as inconsistencies in data formats (kilograms versus pounds, or centimeters versus meters), or even delays in data researching your server from machines if they are not connected to the internet.
At this stage, it’s essential to account for these risks and manage them effectively where possible.
Step 3: Build and test your data analytics in manufacturing models
Before you can unleash your data analytics solution, you need to validate it to ensure that it works. But how?
Generally, this step takes place in the cloud environment due to its potential for data processing. At this stage, you may decide to use custom-built software and tools to test your models and see if they work.
As models are trained, over time they will improve. This stage is often iterative, meaning that the first round of tests is unlikely to yield the best results right off the bat. However, after validating for some time, you will achieve a higher level of accuracy.
As an alternative, you may also consider using ready-to-go solutions like Gini Machine, that are specifically designed to verify and test data analytics models.
Step 4: Deploy and analyze the results
Once you have a data analytics in manufacturing strategy that works for your needs, next comes the best part—putting it into action. Deploy your analytics solutions and get insights about your manufacturing process in real-time.
At this stage, you might also consider engaging in predictive analytics quality management to ensure that your solution continues to function as it should, as if one element changes this can impact your future results.
Moving forward, predictive analytics for manufacturing can be utilized to improve various areas of your business from upgrading individual systems to overall business processes.
Next Steps for Businesses Interested in Onboarding Predictive Analytics in Manufacturing Technologies
Any digital transformation in your manufacturing business is a huge step forward and will require significant investment to realize efficiently to ensure the results you need. To get it right the first time around, and avoid wasting valuable time and resources, it’s worthwhile connecting with technology-first teams that know predictive analytics like the back of their hands. At GiniMachine, our team focuses on predictive AI analytics software for finances, which can boost your business and deliver greater capabilities when it comes to prediction.