ML Model Performance Evaluation: Gini Index, ROC-AUC, Kolmogorov – Smirnov Score
If you’re unsure about the reliability of the data you have at hand, we can provide guidance on how to verify it. In this article, we’ll discuss three commonly used metrics for evaluating ML model quality: AUC/ROC curve, Kolmogorov–Smirnov score, and Gini Index.
What is the AUC/ROC curve?
ROC for Machine Learning Models
The Receiver Operating Characteristic Curve, or ROC curve for short, shows the relationship between True Positive Rate (TPR) and False Positive Rate (FPR) in a plot. TPR is the proportion of correctly predicted positive instances, while FPR is the proportion of incorrectly predicted negative instances.
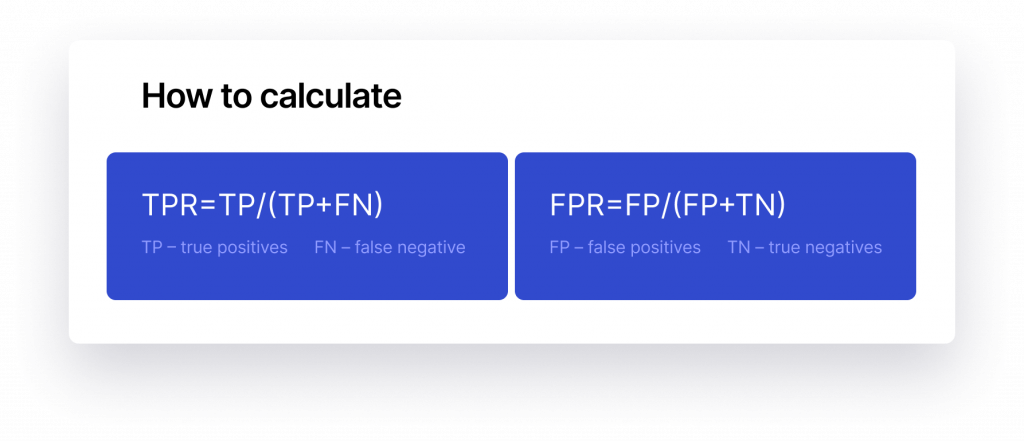
Area Under the Curve (AUC) for ML Model Evaluation
AUC, which stands for Area Under the Curve, measures the overall performance of the ROC curve. It ranges from 0 to 1.
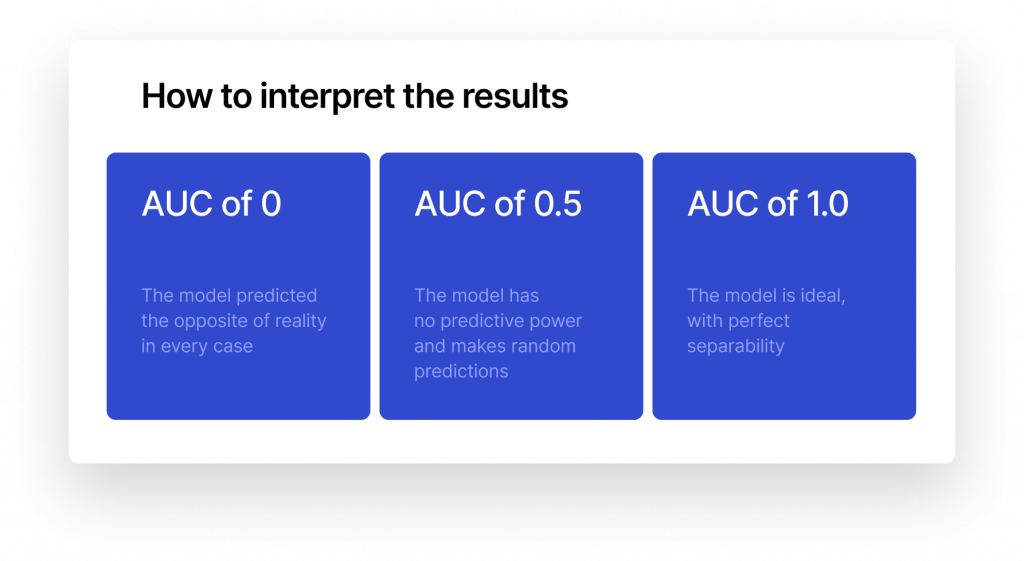
AUC/ROC score was of two tools that are industry-standard math indicators. Now we’ll discuss the Kolmogorov-Smirnov (K-S) test.
What Is the Kolmogorov-Smirnov (K-S) Test
The Kolmogorov-Smirnov, or K-S test, is a statistical method used to assess the similarity between two probability distributions or to compare a sample distribution to a reference distribution. It measures the maximum vertical distance between the cumulative distribution functions (CDFs) of the two distributions.
K-S Score in Machine Learning
In the context of machine learning model performance evaluation, the K-S score can be applied to assess how well a model’s predicted probabilities align with the observed outcomes. It helps determine if the predicted probabilities significantly deviate from the actual data.
To perform the K-S test, the observed data and the model’s predicted probabilities are sorted in ascending order. The CDFs of the observed and predicted distributions are calculated, and the maximum difference (K-S statistic) between the two CDFs is computed. This number also called the K-S score of machine learning, can be anything between 0 and 1.
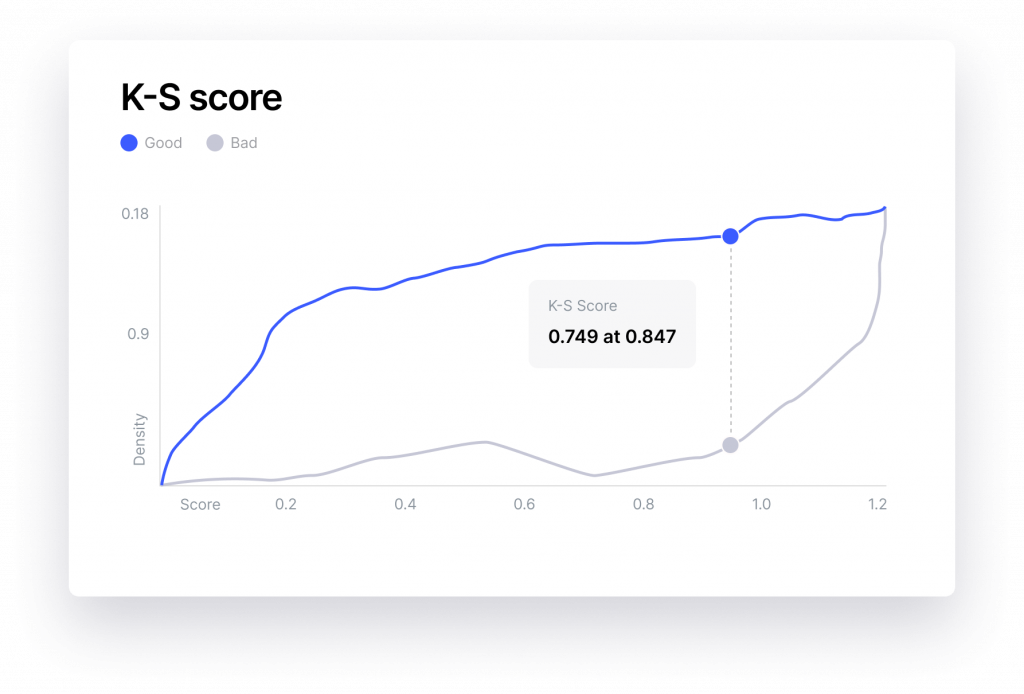
What Is the Difference Between Kolmogorov-Smirnov (K-S) Test and the AUC/ROC Curve?
To put it simply, the K-S test is used to assess how well a model’s predicted probabilities align with the actual observed outcomes. The K-S test provides a single statistical value (the K-S statistic) and a p-value to determine if the model’s predictions significantly deviate from the observed data.
AUC/ROC curve is a graphical representation of the trade-off between the true positive rate and the false positive rate at various classification thresholds. The AUC (Area Under the Curve) quantifies the overall performance of the model across all possible thresholds. A higher AUC value indicates better discriminative ability, with an AUC of 0.5 representing random performance and an AUC of 1.0 representing perfect separation.
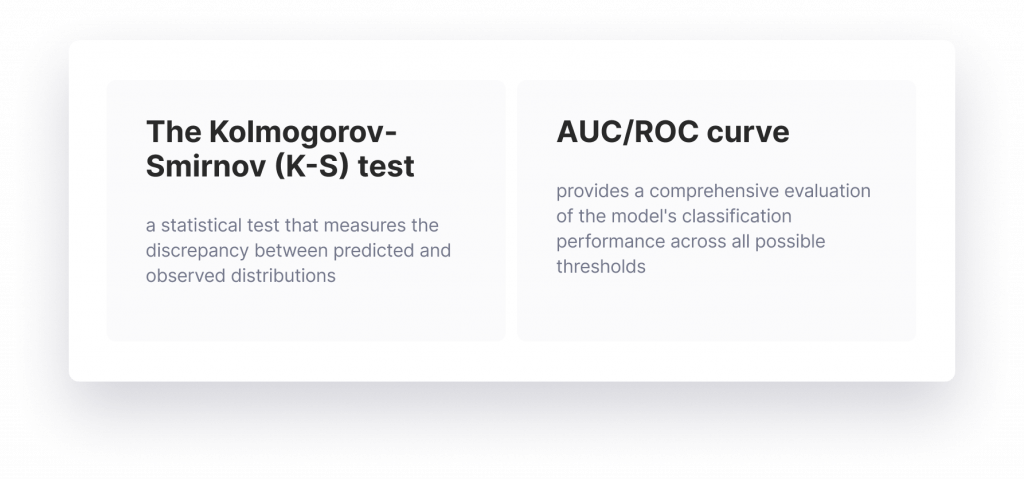
The combination of the Kolmogorov-Smirnov (K-S) test and the AUC/ROC curve provides a more detailed evaluation of model performance, although it involves a higher level of complexity.
Get the Human Involved: Explaining the Gini Index
Originally used in economics, Gini Index turns out to have a strong link to the ROC/AUC score. It also allows for human involvement, making it an excellent choice for companies with data scientist teams.
Gini is unbiased and based solely on historical data and statistics. A human can’t remember all the information from years of work and draw conclusions, but our software can.
In the report, you can observe the impact of each parameter on Gini, including its weight and average value if it represents a numerical indicator.
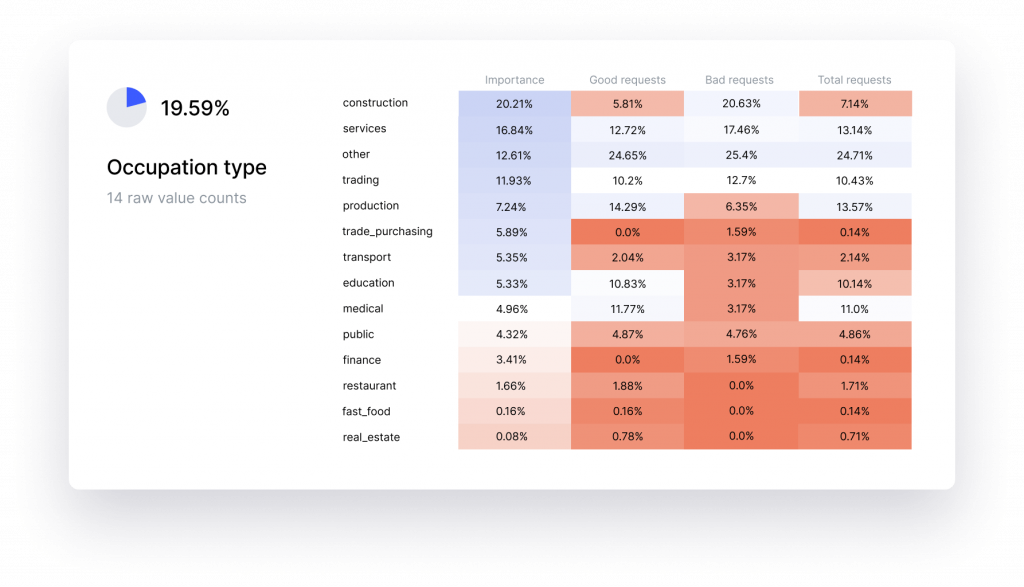
Gini takes into account numerous factors and performs comprehensive analysis that would be humanly impossible to replicate. It considers all parameters and assigns weights to each of them, indicating their respective influence on the decision-making process. This way, GiniMachine ensures a thorough and objective assessment based on the collective impact of all relevant factors.
Gini Index for ML Model
Gini Index can be anything between -1 and 1, and a 0 score equals 0.5 ROC AUC score, which makes it easier to understand.
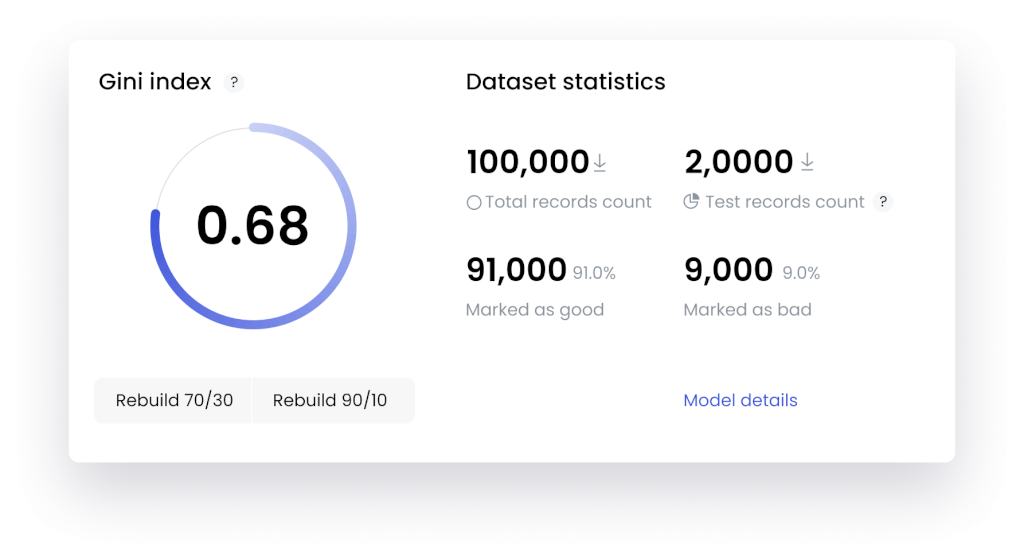
The logic behind GiniMachine predictive modeling is the reason why due to the ease of interpretation, the Gini Index is currently the main parameter for determining the model quality.
ML Model Evaluation Done Right
When you invest resources in evaluating the quality of a built model, you can understand how well it aligns with the desired outcomes and whether it provides valuable insights for your lending business. It also allows for identifying any potential issues or shortcomings early on, enabling improvements and refinements to be made. On top of that, it helps validate the model’s effectiveness and facilitates informed decision-making based on reliable and trustworthy outputs.
We hope that the three commonly used methods covered in this article will prove valuable to you. Below is your benchmark that can be used to ascertain the suitability of the model for commercial purposes.
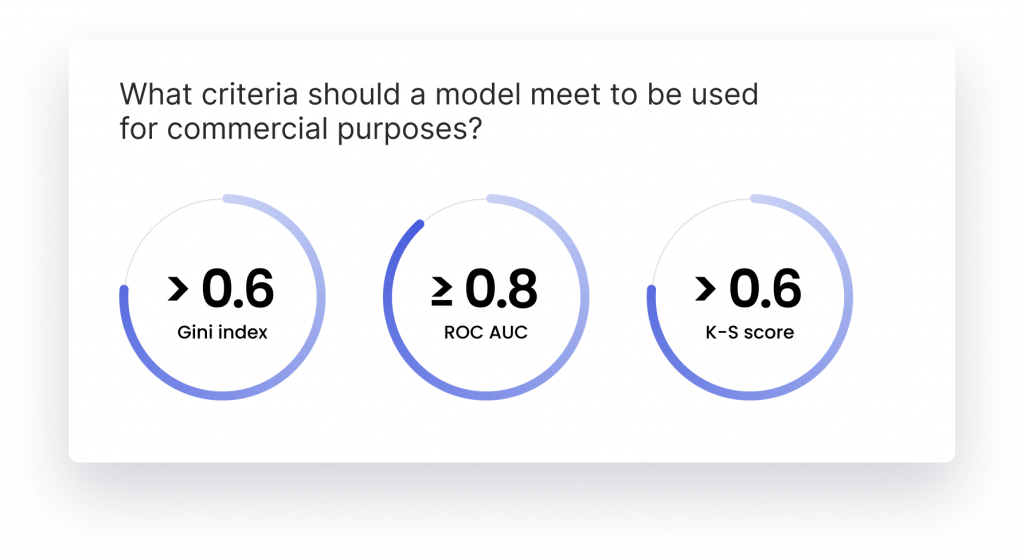