Artificial Intelligence and Machine Learning in Financial Services: Fear of AI & ML for Business
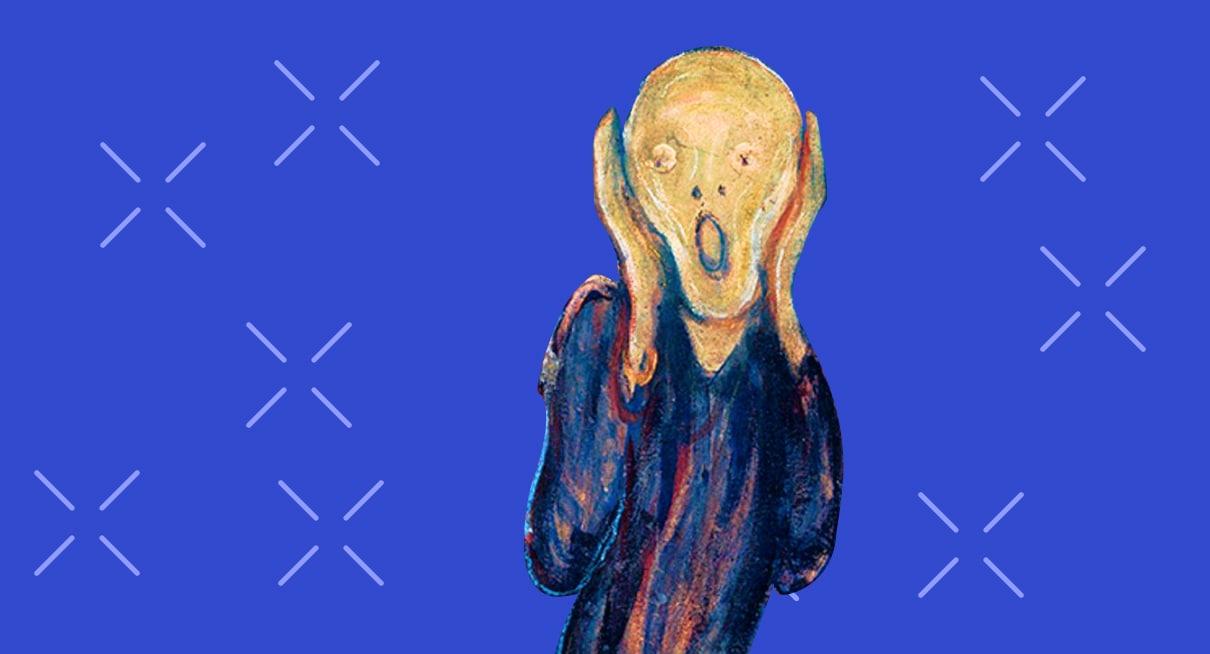
Recently great scientists have been expressing negative concerns and even fear of AI and ML technologies. Physicist Stephen Hawking told BBC that “the development of full artificial intelligence could spell the end of the human race [1]”. Even though fear of artificial intelligence in defense software development is completely justified, its implementation in the financial domain is far less dangerous. To date, “scary” artificial intelligence and machine learning are some of the most fundamental technologies humanity has ever seen and AI capabilities today in the financial sector are unbelievable.
In this article, we want to break down several myths and AI worries to prove that the technology considered as an “enemy of humanity” can be a great business partner. The goal is to explain the bindings between artificial intelligence and machine learning in financial services. We’ll show really-existing examples of how companies use the benefits of artificial intelligence in finance and turn its would-be disadvantages into a game-changing customer approach.
What’re the Advantages of Artificial Intelligence and Machine Learning in Financial Services?
Artificial intelligence (AI) is a technology that allows a machine (program) to replicate and reproduce human behavior, while machine learning is a subset of AI which allows a machine to automatically learn from past data without programming explicitly. To put it simply, a developer implements AI in a computer to solve complex problems as humans do, and uses ML algorithms that allow the machine to learn from previous data to give accurate output.
The most interesting AI and ML-based applications for business are platforms for cyber attacks predictions, Amazon’s Alexa for exploring target marketing base, online recruiting automation apps, credit scoring and decision-making solutions for lenders, etc.
With the growing implementation of AI and ML among banks and fintechs, there is no surprise that the market size of the technology is dramatically increasing. According to Fortune Business Insights, the market size of artificial intelligence in 2019 was valued at $27.23 billion. And this figure is projected to reach $266.92 billion by 2027 – almost tenfold growth in eight years with a CARG of 33.2 percent.
Widespread concern is for the reason, AI and ML opportunities for business allow reshaping workflow processes and win over competitors. Artificial intelligence and machine learning in banking provide the following opportunties:
- Automation leads to both higher production and more efficient use of resources and employees. It reduces workflow time and allows time redistribution per task.
- Data analysis powered with AI and ML can be used to process data more efficiently. It can help to build predictive models to process data within seconds and forecast potential outcomes with high chances.
- Repetitive task management is the benefit that can save your time and automate routine tasks without the need for human intervention.
- Risks reduction with AI tools allows cutting off the chances of manual errors. This can be especially beneficial for businesses that calculate each risk before making a financial decision.
How Developers Address Fears of AI and ML in Finance
Why are people so afraid of technology? Having an impressive amount of benefits for business, AI remains scary technology for users. This can be easily explained that the technology is quite new. The first AI-powered program was developed in 1955. However, only in the 1990s and 2000s were achieved the landmark goals of artificial intelligence, including the first defense of AI over a world chess champion Gary Kasparov.
AI worries are reinforced by movies and science fiction books like Transcendence or I, Robot where machines become too smart and may harm humanity. We feel general anxiety about AI and its potential capabilities, and that’s OK. However, life differs from movies. Actually, an AI-powered machine cannot become a dangerous thing to humanity in a couple of days. Every program always has a certain set of functions, i.e. possibilities, beyond which the machine can not move in general. So if your developer has not programmed the robot to destroy humanity or ruin your business, you have nothing to worry about.
Other fears of AI in business stem from a few common causes: fear of unemployment, concerns about machines making wrong decisions, unethical issues, and dehumanization of tasks. Let’s discuss all of them in more detail on the example of AI and machine learning use cases in banking and finance.
#1. AI technology causes mass unemployment
One of the major fears of AI is based on the idea of mass unemployment when machines replace workers. The idea is reinforced by the historical mass unemployment after the Industrial Revolution in the 18th and 19th centuries. However, there’s a difference between the current situation and the past. The revolution was targeted at reducing manufacturing-oriented jobs, while AI is aimed at changing the work of white collars.
Systems based on artificial intelligence aren’t supposed to replace many human jobs. AI in business provides lots of capabilities, automates, and reduces possible manual errors, but it can’t operate in a fully automated mode. The technology is supposed to support humans at routine tasks, so the demand for economists and accountants will continue to grow. The machine just replaces old ways of making reports, for example.
According to Adobe, the impact of artificial intelligence in business is projected to increase labor productivity by 40% and enable people to use working time more efficiently. So, AI doesn’t kill jobs, it kills outdated ways of doing jobs.
For example, Vic.ai develops software for efficient accounting. Their software can select invoices meeting the set confidence threshold and automates them sending to approvers. As a result, an accountant doesn’t need to spend hours looking for the “right” invoices and sending everyone to a receiver.
#2. AI violates ethics issue
No matter how and where we want to implement AI – application scoring, stock market, risk management, chatbots – you need to collect as much data as possible to gain a reliable result. And this is not always considered ethical.
Let’s review the situation with a broader amount of analyzed data in credit scoring. To calculate credit scores more correctly, AI solutions need more historical and personal data. Not everyone is eager to present it in detail. According to the surveys, 36% claim that they’ll share more data only in case of tighter legislation aimed to protect borrowers’ rights when they apply for funding and to restrict the information included in the assessment. While 20% believe that the use of non-traditional data oversteps the ethical line and should be controlled.
To deal with the problem, explain to your customers why do you need such information. Prove that your security terms are highly regulated and you guarantee to use the data only for business purposes. The problem is rooted in a bad understanding of AI in business, and deep guideline is solvation here.
#3. AI and ML make wrong decisions for business
When business owners want to buy software for predictions, they’re afraid to choose a solution that makes wrong decisions. Sometimes we find it difficult to believe that a machine can make accurate predictions, just because we don’t understand how it works. In ML and AI solutions, the word “prediction” refers to the output of an algorithm after its training on historical data and applying it to fresh data when we need to make a forecast. So, the system, in general, cannot make worse decisions than you used to do, simply because it analyzes your experience to avoid such mistakes.
To secure users from possible bad decisions, developers create AI solutions able to check their work. The user can monitor the efficiency of taken decisions and rest assured that the risk-management processes work correctly.
Among good examples of AI and machine learning in banking is GiniMachine – no-code software for accurate scoring. The system is helpful for banks, credit unions, lenders, and others who need to predict risks and take decisions according to their cut-off value. GiniMachine also runs blind tests, taking 30% of analyzed data for self-checking. This way, users are always sure that they can rely on the system in credit risk management.
#4. AI approach dehumanizes customer experience
Sometimes customers might feel anxious due to the absence of a human response from a service provider. In cases when users need a real human touch, AI can have some bad calls, especially when a customer needs certain information that isn’t set in its program.
There’s always a need for human intervention, considering B2C communication, especially when it comes to solving customer issues connected with rational thinking and morality. As a solution, the majority of chatbot AI developers allow their solution to contact the real support team if a bot can’t help a user. Here a chatbot is mainly used to reduce the burden on the support team.
However, the statistics show that about 70% of consumers use chatbot support and are satisfied with the outcome.
Among interesting examples of AI for business communication, we can note Flow XO. The chatbot welcomes website visitors, answers simple questions, and accepts payment by identifying a service or product customers would like to purchase. Besides, Flow XO allows you to hand over a discussion with a real support team representative on live chat when needed.
AI and Machine Learning in Financial Services: Yes or No?
Being scared of AI technology doesn’t always mean that it’s dangerous for mankind. Sometimes we just don’t understand how it works or how to turn its cons into pros. Considering AI and ML implementation in banking, the technology doesn’t cause mass unemployment, doesn’t dehumanize the whole communication process or makes bad decisions every time leading you to bankruptcy. Being scared of technology here means that you should discover the AI software, understand it, and carefully use it in business.
AI and ML drive lots of business benefits. With a quality solution onboard, businesses can automate processes that usually take weeks or even months. It’s the best way to allocate resources, reduce operational costs, and find breaches in task performance.
Should we worry about AI? Get in touch with GiniMachine to meet AI and machine learning in financial services closer.