Bankruptcy Prediction Using No-Code AI: Your How-To Guide
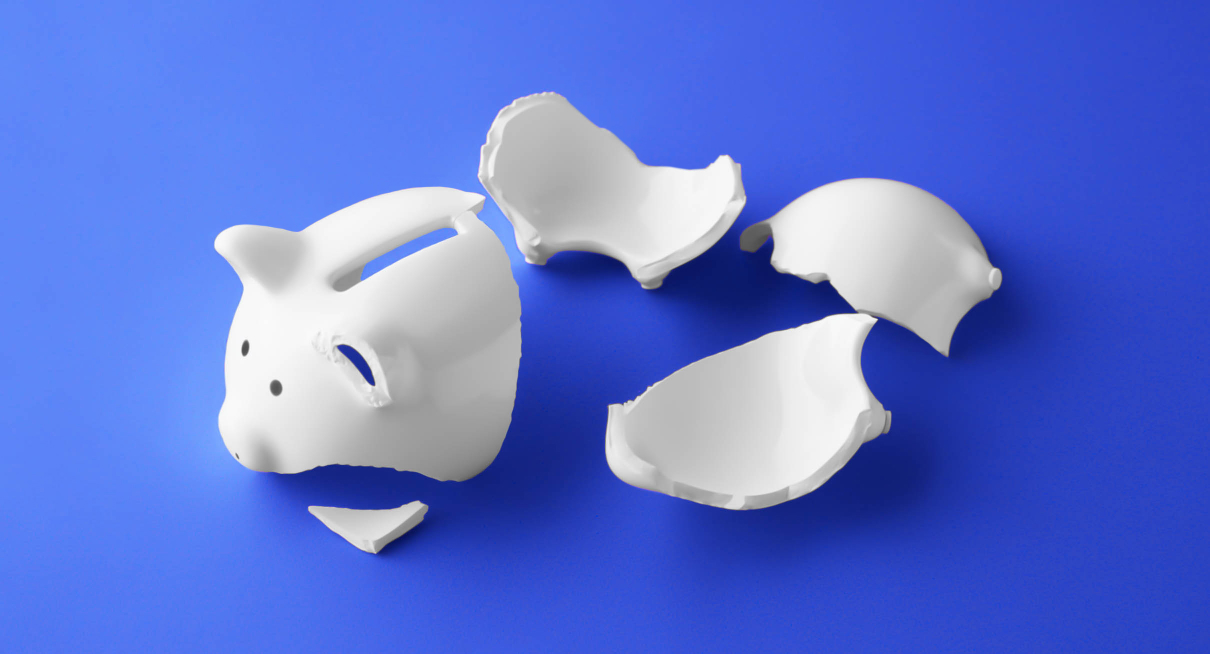
Spotting the early signs of bankruptcy can help avert a financial disaster. Let’s consider the 2008 crisis. The world, prior to the downturn, was experiencing a boom, growth was happing across many sectors and the economy seemed to be going well. That was, until it wasn’t. The crisis seemed to come out of nowhere, but what if there were some missed early warning signs? Bankruptcy prediction software enables companies to detect the early signs of default before they happen and potentially avoid them altogether.
During the 2008 crisis, some very observant market watchers did spot the signs. But they were in the minority. But what if there was a better way to predict bankruptcy? One that was more reliable. With the latest machine learning financial forecasting software, now it’s possible to keep a closer eye on the market, and its trends and identify bankruptcy risks before they occur.
What Is Bankruptcy Prediction Software?
Bankruptcy prediction software utilizes artificial intelligence and machine learning technology to detect the early warning signs of financial distress within a company. When using AI or machine learning for financial forecasting, a company, be it a small or medium-sized enterprise (SME) or large-scale business can more accurately predict their financial trajectory and monitor variables utilizing the latest, smartest AI models.
How Does Bankruptcy Prediction Software Work?
Being able to predict default may seem like a dream, but what’s the reality? Let’s take a look at how bankruptcy prediction works in practice.
There are lots of AI models that can potentially work to predict a financial downturn. Here are some of them:
- Logistic Regression–an AI model that determines the probability of an event occurring, in this case, bankruptcy. It uses the supervised learning technique to predict dependent variables using independent variables.
- Perceptron–a machine learning model that is trained using data sets. It can arrange input data and classify it effectively for further analysis. When it comes to bankruptcy prediction, the perceptron model is a classifier.
- Deep Neural Network Classifiers (DNN)–this machine learning approach mimics the human brain’s ability to process information and utilizes neural network technology.
- K Nearest Neighbor Classifier (KNN)–an ML algorithm-based approach that solves classification and regression-style problems when it comes to bankruptcy.
- Bagged Decision Trees–like a tree with many branches, bagged decision tree models account for a number of probabilities and use ML to do so.
- Random Forest–used to solve classification and regression challenges, random forest utilizing ‘bagging’ and randomness to develop uncorrelation in its forest of trees, finding the most effective financial model.
- Gradient Boosting–another model that engages regression and classification to make predictions and detect the risk of error. *
*this list is not extensive and other approaches to ML exist which can be effective in detecting financial risks and irregularities.
To establish how effective these models for bankruptcy prediction are, they are usually compared with a variety of factors, such as:
- Error rates–this notes how often the classifier in the model turns out to be incorrect.
- False positives–these metrics focus on how often a not bankrupt result is given when it shouldn’t be.
- Preciseness–denotes the number of times the model indicates a company is ‘bankrupt’ and the percentage of those times it is correct.
- Accuracy–overall accuracy or correctness of the model
- Sensitivity–how often is the model accurate when a company is genuinely bankrupt.
- Prevalence–when bankrupt occurs in the model, how often does this happen?
By combining these factors, a clearer picture can be established of how to build a tailored model that will suit your company. Including as many variables as possible, without overwhelming your bankruptcy prediction technology presents the most effective chance of developing a machine learning financial forecasting model that meets your precise business needs.
Bankruptcy Prediction without a Single Line of Code
For companies that are ready to invest in technology transformation, these complex models may seem confusing, so what does the selection process look like in practice? And what should decision-makers concentrate on aside from all the tech-speak? Here are the steps any decision-maker should take when developing a bankruptcy prediction and machine learning strategy.
1.Understand the challenge you are trying to solve
While it’s easy to say, ‘preventing bankruptcy’, this does not always define the challenge at hand or why you feel it’s necessary to explore this path at this particular time. Knowing what you hope to achieve is the best start when engaging in new technology.
2.Start to build your strategy
In-house vs. outsource vs. a combination. There are tons of options out there for collaborative and bespoke tools for bankruptcy prediction. Your strategy should outline your approach, your team, your desired results, and how you want to achieve them. At this stage, it’s a good idea to seek professional assistance from a team, such as GiniMachine, to better understand how such software can effectively and realistically be used in your business.
3. Work with the data
Before you’ll be able to engage meaningfully with any machine learning tool for bankruptcy prediction, you’ll need the right data to do so. At this stage, it’s good practice to compile, clean, and process the data you need so it is in a usable format for your machine learning prediction software.
4.Work with your team to build models
Now that you have the data you need, the only thing that’s missing is the effective software that can transform it from just some numbers to effective insights that drive your business and ensure it doesn’t fall into financial hardship. Utilize the skills and experience of your team to develop effective models tailored to your needs. Modern technology even allows your company to engage 100% no-code models that can be seamlessly integrated into your current tech stack and can get to work instantly.
5.Test your models to manage the effectiveness
No AI or machine learning model for financial forecasting or otherwise is 100% effective on the first try. At this stage, it’s important to test, test, and test again your model to ensure it works as expected and to iron out any kinks along the way.
6. Deploy and monitor your solution
Now that your solution is ready, it’s time to test it in action. Rest assured and deployed your solution. However, don’t expect AI predictions to be a one-and-done tool. Instead, this software may require some tailoring later down the line to ensure its continued effectiveness. Don’t be afraid to stop and recalibrate the process if you aren’t getting the expected results. Sometimes tweaking the algorithm can correct errors and allow for evolution in human behavior.
Next Steps in Combining Bankruptcy Prediction and Machine Learning Software
When it comes to designing or onboarding a bankruptcy prediction machine learning model that works directly for your business, it can always be difficult to know where to start. Usually, the best place is to get in touch with professionals, such as the GiniMachine team, who are experienced with the nuances of building effective AI models that get results and work from there.
Interested in GiniMachine? Get in touch with our team to book a demo.