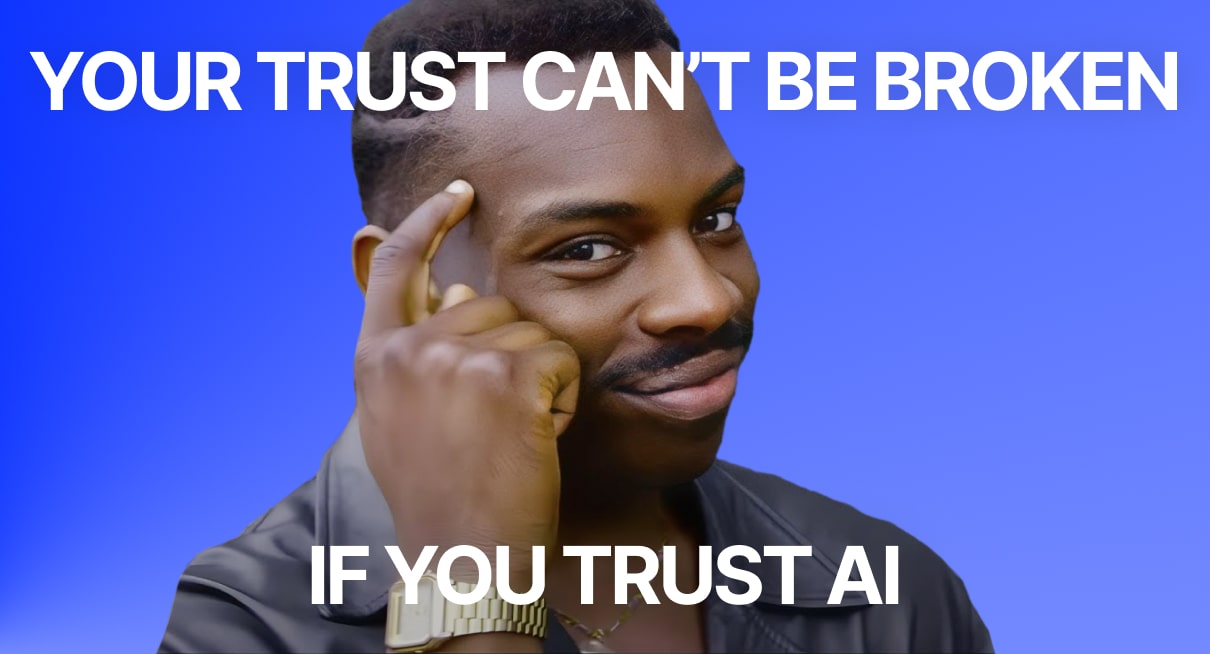
Artificial intelligence (AI) has ceased to be a novel concept and has instead become an integral part of our modern landscape, penetrating various sectors, notably the financial domain.
While its integration promises transformative benefits, the emergence of AI in the finance industry brings forth a mosaic of ethical considerations that require nuanced exploration. This technological marvel carries the potential to revolutionize financial operations, from risk assessment and fraud detection to enhancing customer service.
However, the ethical conundrums it poses regarding fairness, transparency, privacy, accountability, and societal implications demand careful deliberation and proactive mitigation strategies.
In this comprehensive examination, we delve into the intricate ethical issues of AI in finance, highlighting the imperative to strike a harmonious balance between innovation and responsible deployment.
Ethical Challenges in AI Deployment
Ethical considerations shape responsible and sustainable technological advancements. These challenges are not merely theoretical but have profound real-world implications, necessitating a rigorous, thoughtful approach to AI development and implementation.
Bias and Fairness
AI systems heavily rely on the data they ingest for training, ensuring their outputs are as unbiased as the data they consume. Consequently, ensuring fairness in AI algorithms becomes paramount, steering clear of discriminatory outcomes based on race, gender, or socioeconomic status.
To achieve this, meticulous attention is essential during data selection, model development, and ongoing monitoring, aiming to mitigate biases and promote fairness in AI applications.
AI and Privacy Concerns: Data Security and Customer Consent
The significance of AI lies in its dependency on copious amounts of data, which raises significant concerns regarding privacy and data protection. Safeguarding individuals’ personal information and adhering to pertinent data protection regulations are indispensable.
In the context of alternative credit scoring, AI algorithms analyze a broader range of data points, not just traditional credit histories. This approach necessitates a delicate balance between accessing diverse data sources for AI advancements and protecting individual privacy rights. Such balance is essential for building trust and preventing potential harm, especially when dealing with sensitive financial information.
Respect for human autonomy and facilitating meaningful human-computer interaction are pivotal considerations in AI development, necessitating informed user consent mechanisms, especially in sensitive contexts like data collection and surveillance.
Transparency and Explainability of AI Decisions
The opacity of some AI models poses a challenge to comprehending and explaining their decision-making processes, often referred to as the “black box” problem.
Ensuring transparency in AI systems, particularly in domains like healthcare, finance, and justice, is crucial for accountability and trust.
Endeavors must focus on developing interpretable AI models capable of providing understandable explanations for their outputs, fostering transparency and confidence in their applications.
GiniMachine utilizes the Gini Index, a metric to bolster the transparency and interpretability of AI decisions. It measures the effectiveness of scoring models in lending: the more often a model assigns higher scores to reliable borrowers compared to risky ones, the higher the Gini Index.
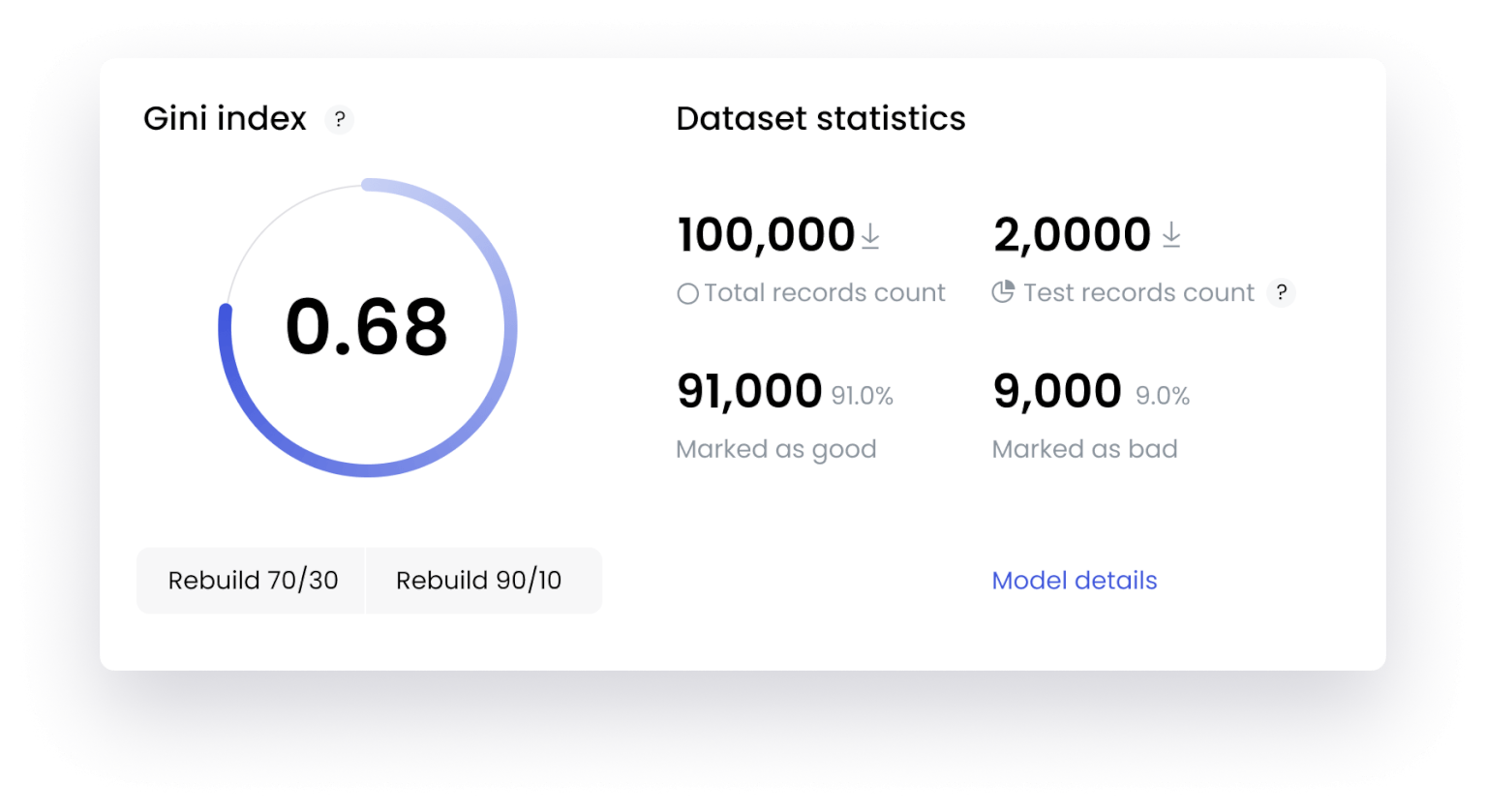
A higher Gini Index indicates more effective differentiation, signifying a robust and reliable model. This metric, derived from the Lorenz curve, which graphically represents the distribution of a variable (like income), provides an intuitive, numerical gauge of a model’s discriminatory power. By translating, the Gini Index translates complex AI decisions into an understandable index, as well as demystifies AI processes and fosters trust in AI applications.
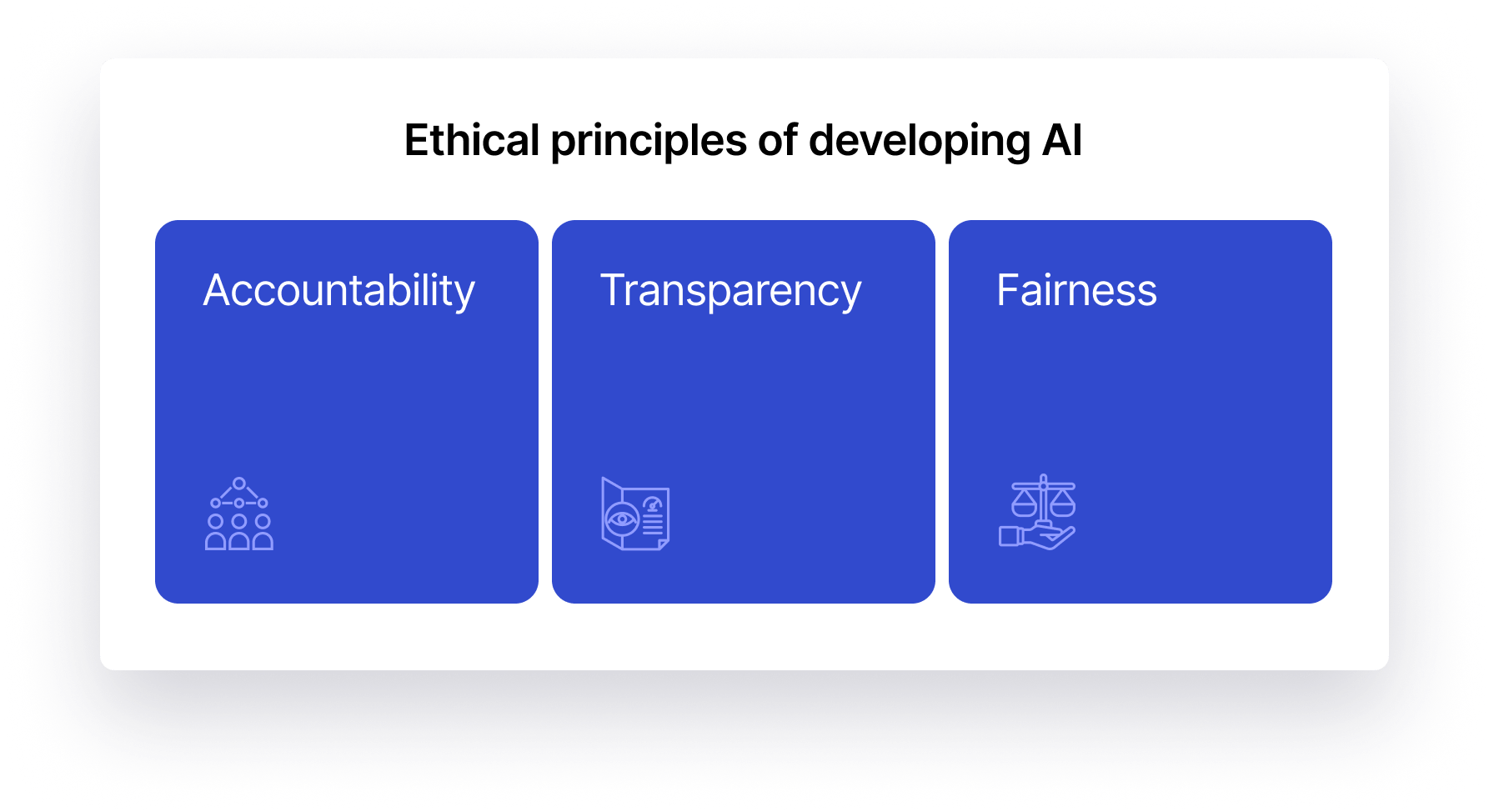
Best Practices for Ethical AI Deployment
Ethical AI deployment is a critical necessity in today’s technology-driven world. Here, we explore the key practices essential for the ethical deployment of AI.
Developing AI with Ethical Principles: Fairness, Accountability, and Transparency
Collaboration among developers, policymakers, and experts is pivotal in crafting embedded AI ethics principles that cater to business needs while addressing ethical concerns adeptly.
AI specialists need to adopt more ethical metrics to gauge their models’ success. It’s crucial to deeply examine the inherent biases within these models and understand their impact. Efforts should focus on finding ways to minimize these biases. Data engineers also play a pivotal role in recognizing and neutralizing biases within the data before it even enters the training phase.
Regulations already exist to manage privacy breaches, and both the government and consumers keep a close eye on this. However, it’s essential for consumers to be vigilant and read through the terms and conditions carefully, even though they’re often transparent but can sometimes seem superficial.
Another key area for AI experts to focus on is developing methods that increase the explainability of decisions without sacrificing the model’s performance. Data presentation is evolving rapidly and should encompass not just showcasing outcomes but also explaining the significance of the model’s internal parameters.
Incorporating Stakeholder Input and Societal Values
Incorporating diverse stakeholders in the design and development process is essential to ensuring ethical AI algorithms. Engaging ethicists, social scientists, and representatives from affected communities helps align AI development with societal values.
Prioritizing the establishment of ethical guidelines and standards guides the development and deployment of AI systems, fostering responsible practices.
Continuous Monitoring and Evaluation of AI Systems
Maintaining AI systems necessitates ongoing monitoring to detect and address unintended consequences. Responsible embedded AI demands a robust and adaptable security system to align with evolving needs.
An effective API governance framework is crucial not only for the seamless integration of AI capabilities but also for enhancing security measures and staying vigilant against potential risks posed by threat actors.
This blend of oversight and API governance fosters growth, adaptation, and, most importantly, confidence in AI systems.
Case Studies: AI Ethics Principles in Finance
HSBC and AI for Fraud Detection
In a bid to fortify its global operations against financial crime, HSBC joined forces with Element AI to create an AI-driven anti-money laundering (AML) system. This innovative system meticulously sifts through colossal data volumes, aiming to pinpoint dubious transactions and activities. What sets it apart is its knack for explaining decisions and offering comprehensive audit trails, which are crucial for compliance.
The impact? HSBC has seen a remarkable drop in false alarms and an upswing in operational precision, saving both time and expenses. This AI-powered solution underscores how AI can substantially bolster regulatory adherence and the integrity of operations.
Equally vital is its interpretability aspect, which plays a pivotal role in upholding HSBC’s transparency and accountability in combating money laundering.
Mastercard’s AI for Financial Inclusion
Enter Mastercard’s foray into leveraging machine learning to support open banking solutions, spanning credit scoring, financial insights, account initiation, and payments.
Through this technology, lenders gain the ability to analyze consumer data—like bills and banking transactions—to gauge repayment capacity and assess credit eligibility.
The resultant advancement in credit modeling has broadened access to credit for marginalized demographics. This initiative significantly bolsters financial inclusivity, offering opportunities to individuals traditionally underserved by the financial sector.
To navigate the swiftly evolving digital landscape responsibly, Mastercard invests in a cadre of data scientists, AI technologists, and governance experts. Their joint efforts ensure ethical AI use, aligning with Mastercard’s public commitment, the Data Responsibility Imperative.
This pledge emphasizes individuals’ rights regarding data ownership and usage, reinforcing ethical data practices in AI deployment.
American Express and Ethical Credit Scoring
Leveraging AI to refine credit scoring models, American Express aims to make credit decisions more inclusive and fair. The AI-driven system assesses a wide range of data points, including non-traditional ones, to evaluate creditworthiness, enabling a more comprehensive and fair assessment.
This approach reduces the risk of biases that can arise from conventional credit scoring methods. In line with ethical AI practices, American Express is careful to ensure transparency and fairness in its AI models, taking strides to avoid inadvertently discriminating against any group.
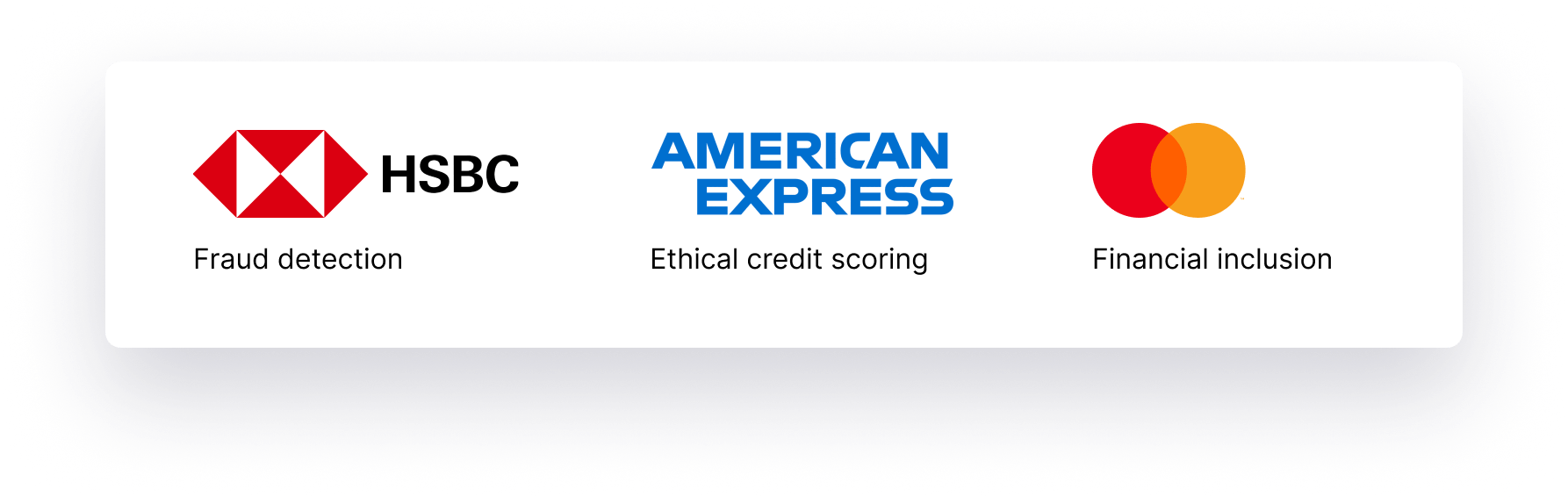
Conclusion
Ethical considerations are fundamental in guiding AI towards responsible development and deployment, ensuring it serves the greater good of humanity. This requires a unified effort from technologists, policymakers, and society. By embedding ethical frameworks in AI, especially in the fintech sector, we achieve more than just technological advancement; we uphold ethical responsibility, paving the way for a more harmonious and inclusive future. For those interested in seeing these principles in action, particularly in fintech, contacting GiniMachine for a demonstration would be a valuable next step.