How to Improve Credit Risk Management: AI for Banking
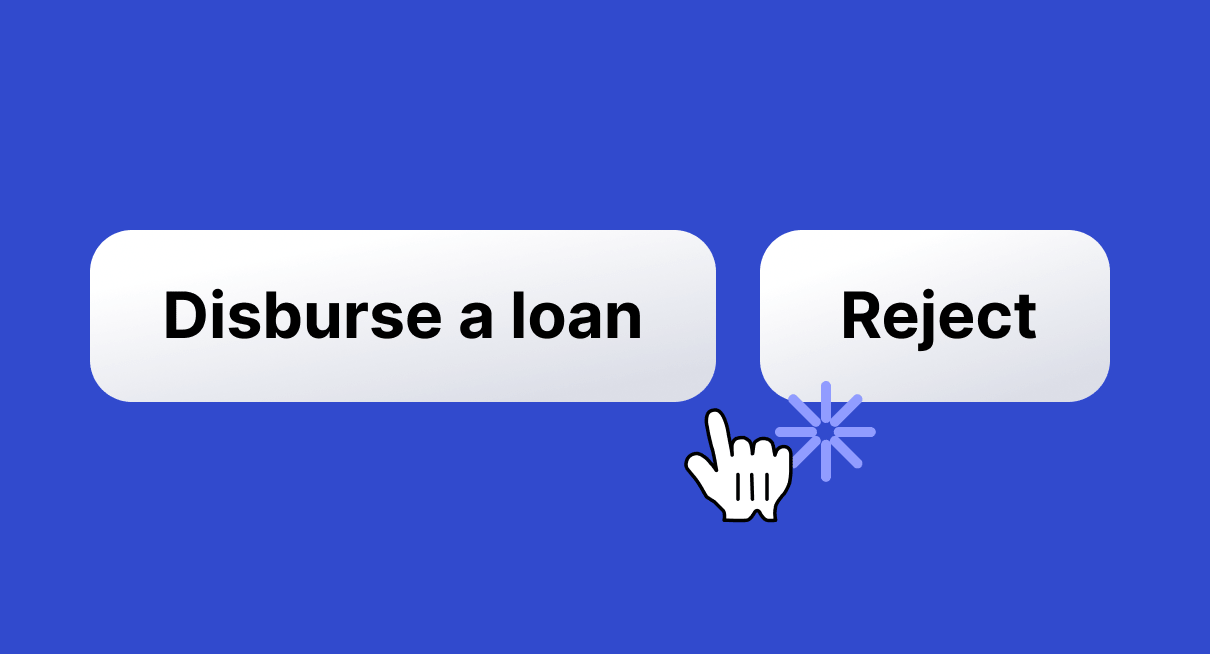
Loan granting reminds Russian roulette — you never know when it shoots. However, a bank can calculate credit risks and reduce the number of possible bad loans, while a player can’t.
In this article, we decided to compare traditional credit risk management processes with the modern artificial intelligence (AI) approach. Technologies never stand still; companies that implement top-notch solutions and partner with fintech organizations have a huge market advantage. Let’s discover the pros and cons of a traditional credit risk management process and realize how artificial intelligence tackles the disadvantages of the old system.
What is Credit Risk Management: Pros & Cons
If you’re looking for ways to empower your credit risk management, you 100% know what credit risk means. But, just in case, let’s explain a little; credit risk is a simple term that refers to risks that a bank takes lending out money. The majority of banks and financial organizations can’t fully assess whether a borrower returns the money or not. Moreover, sometimes an economic shift plays a bad game with borrowers who have always been paying on time. As a result, a bank loses money. So, how do banks reduce the number of bad loans? They manage their credit risks. And the majority of businesses start implementing AI solutions.
Today’s competition in the banking market is strong — the number of only FDIC-insured commercial banks in the U.S. exceeds 4,000, not to mention the uninsured. And their number is declining from year to year.
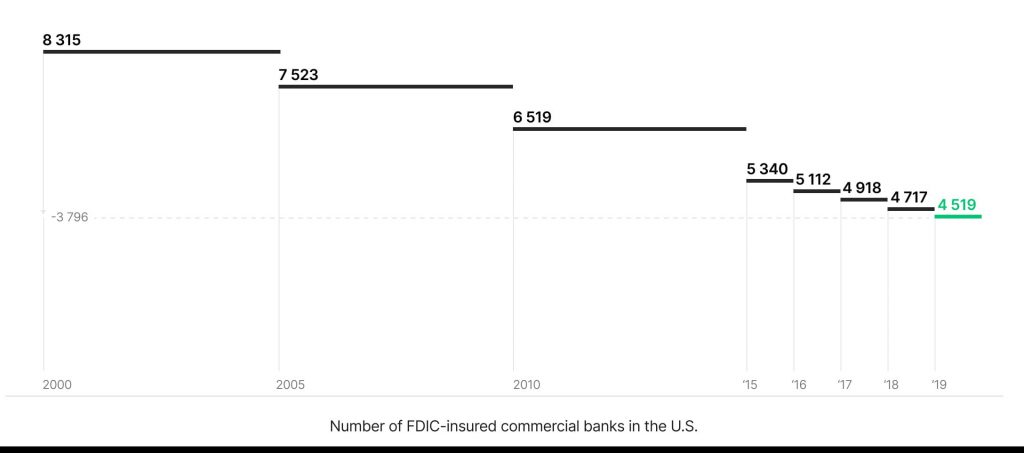
One of the main aspects contributing to the competitiveness and existence of banks and alternative lenders is credit risk management. Banks, traditionally, set credit risk parameters, according to the financial opportunities, and provide loans only to clients within this number. It’s a practice of loss reduction when a credit risk manager identifies risk factors and selects measures to manage loan activities in the future.
Advantages of Credit Risk Management:
- Ability to measure and predict the risks of any single application.
- Allows banks planning strategies ahead to avoid a negative outcome.
- Using various credit scoring models, it’s possible to figure out the best ones for the business and determine the level of risk while lending.
Thus, risk management banking allows them to withstand the competition on the market and not to drown in unrepaid loans.
Disadvantages of Traditional Credit Risk Management:
- Risk predictions do not guarantee low percentages of bad loans; the approach isn’t scientific, so the results might be judged in several ways.
- Outdated systems can overlook various factors and thus make incorrect predictions about certain borrowers.
- Financial losses due to the failure of a credit risk model.
- A long period of time between a loan application, its approval, and issuance.
- Credit scoring models may provide completely different scoring results, complicating the lending process.
- The cost and work of the majority of credit scoring models are questionable.
Lack of modern risk assessment tools and limited view of risk measures lead to incorrect analysis of a borrower’s abilities to pay back. It’s crucial for either banks or small lending organizations to completely understand a loan loss reserve to measure credit risks. As a result, traditional scorecards by credit risk managers are no longer enough to recognize bad borrowers among the others.
How does AI Assist Credit Risk in Banks?
With the rising popularity of virtual assistants, self-driving vehicles, and automated management systems, AI in combination with machine learning (ML) takes over more and more business domains. It remains a high technology for digital credit scoring. Combining artificial intelligence with traditional credit scoring helps to run and analyze various data points on borrowers, including historical and transactional. Covering more data, you can predict a borrower’s behavior, improve the accuracy of banking processes, and shorten the time of loan decision-making.
According to Finance Monthly, 31% of respondents think that non-traditional data lead to better credit scoring. While 14% of the market professionals prove that AI scoring systems already outperform human-based ones. There are also respondents who guess that a broader range of data reduces subjectivity. However, users can easily exclude unnecessary parameters from the analysis or create a custom business solution.
Let’s consider the benefits of using AI for credit and risk management.
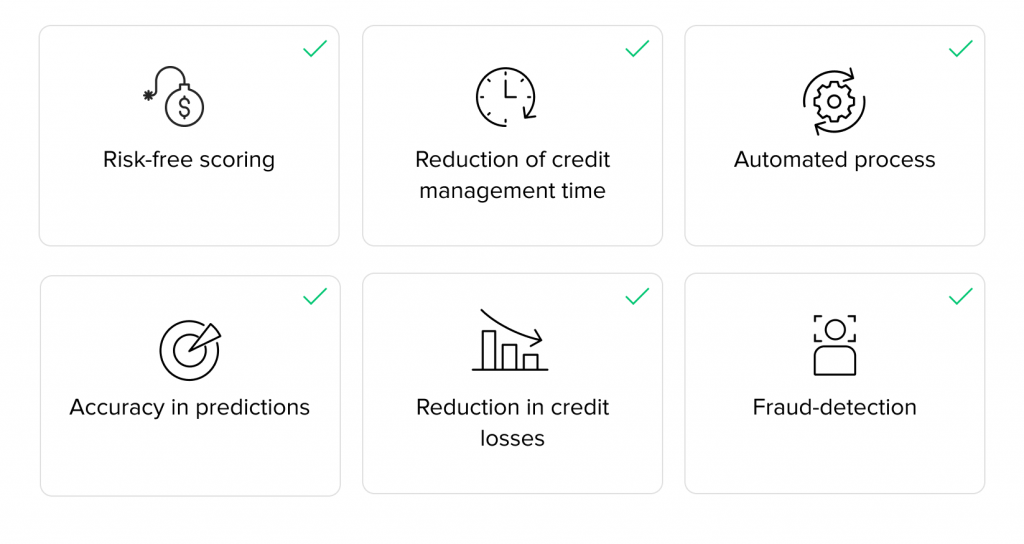
- Error-free. The traditional method applied by banks and financial institutions is not error-free. Mistakes might lead to failure to recognize potential defaulters, as well as you can reject an applicant who might be valuable for the organization. AI credit risk tools can better process a huge amount of data and identify patterns and creates as much error-free credit risk management as possible.
- Reduction of credit management time. Financial institutions and banks spend a significant amount of time to physically verify applications. Artificial intelligence significantly reduces time to market. For example, the GiniMachine solution can verify 1,000 applicants in 10 seconds. This significantly changes credit management time.
- Automated process. Organizations and banks, working in a traditional way, usually have to hire a quality team able to deal with big data and generate reports. But AI can automate the whole process practically eliminating human participation in credit risk scoring. This allows banks to set free people with other vital tasks, or cut staff.
- Reduction in credit losses. The main goal of credit risk management tools is to predict delinquencies before they occur, take timely actions to reduce credit losses. While AI can predict delinquencies for a year in advance. The only thing is that you have the right data necessary to build a model.
- Accuracy in predictions. Traditional scoring models might be tight since they function according to clearly defined parameters. AI solutions are intuitive; the more new datasets they analyze, the better they learn, coming up with more accurate predictions.
Artificial intelligence brings great efficiency to credit risk management reducing time to market. In addition, it strengthens fraud detection mechanisms making your bank more appealing for liable borrowers.
Wrapping it Up
There is no doubt that credit risk management with AI becomes a key area for banks and financial organizations. It solves practically all issues caused by traditional scorecards improving banking workflow. Risks will continue to grow, and AI can almost completely reduce them. So, it’s time for your business to embrace the potential of artificial intelligence in credit scoring.
Need a high-end credit scoring solution? Contact us to try Ginimachine.