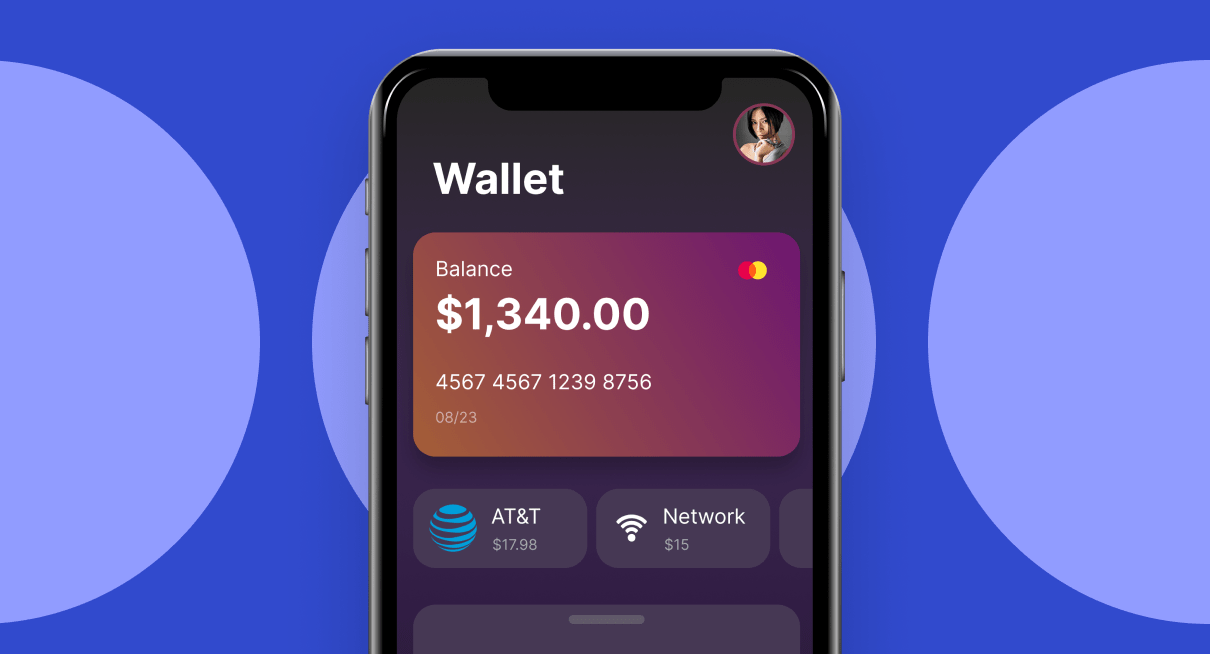
According to McKinsey, risk management standards are rising, making predictive analytics in banking a must-have.
With the rise of Big Data, financial risk modelling requires much expertise and thorough management. Errors in datasets can lead to near-fatal losses. Asia-Pacific bank, for example, lost $4 billion when applied an interest-rate model that contained incorrect assumptions and data errors. Needless to say, the stakes are high.
Flaws in risk management can impact not only financial institutions but borrowers as well.
“If banks are less able to differentiate a risky borrower from a non-risky borrower, lenders and servicers have to hedge for that risk. As a result, lenders will have to charge higher interest rates across the board. Overall, consumers actually get hurt”.
Nick Larson, business development manager at LexisNexis Risk Solutions.
As risk management issues mount, current approaches can no longer focus resources efficiently to make better data-driven decisions. The only solution is a system widen root-and-branch overhaul with AI.
AI in Banking: Beyond the Hype
Analysts estimate that artificial intelligence will save the banking industry more than $1 trillion by 2030. In particular, applying AI to risk management will help banks save $31 billion (according to Autonomous).
Financial institutions already implement advanced machine learning techniques across various channels:
- chatbots and virtual assistants;
- customer segmentation;
- portfolio management;
- risk modelling and underwriting;
- fraud and churn predictions;
- data analysis and more.
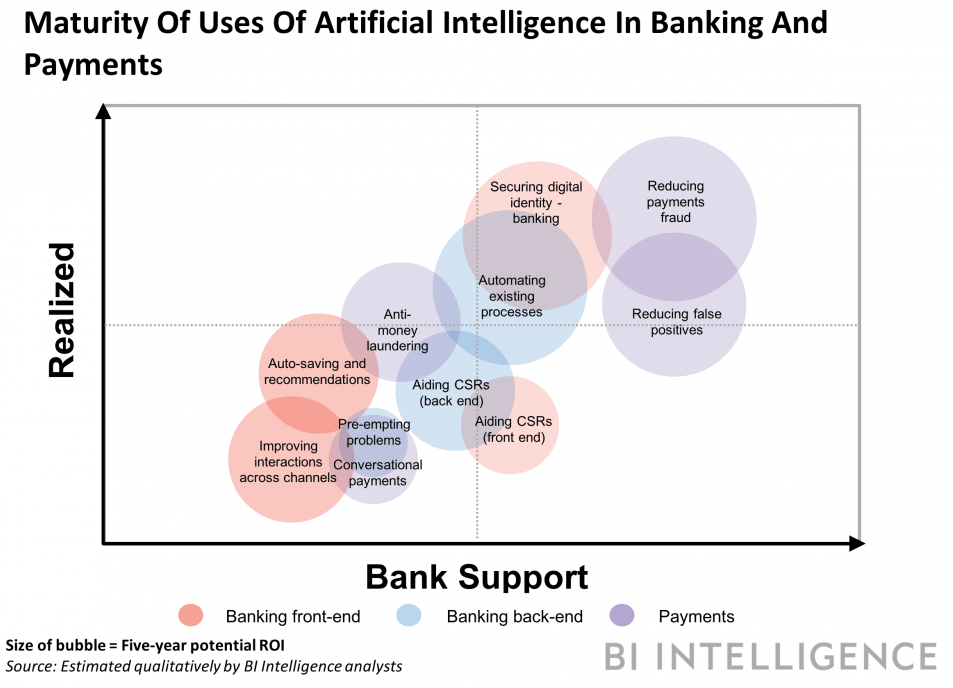
A number of industry giants such as JPMorgan Chase, BBVA, HSBC and OCBC have already started to use back-end risk management with AI to automate processes, aid employees, and solve critical issues in the underwriting process.
Areas of struggle for legacy underwriting
According to the World Fintech Report, the relationship between FinTechs and traditional financial institutions has morphed from competition to collaboration. This happens because FinTechs are focused on narrow functions and segments underserved by legacy financial institutions.
Legacy credit underwriting issues:
- Inefficient data and portfolio management. Inability to gain, process, and manage the right data leads to a higher degree of risk than necessary to achieve a target return. In order to achieve competitive industry insights, having high-quality data is a must when it comes to risk-return models.
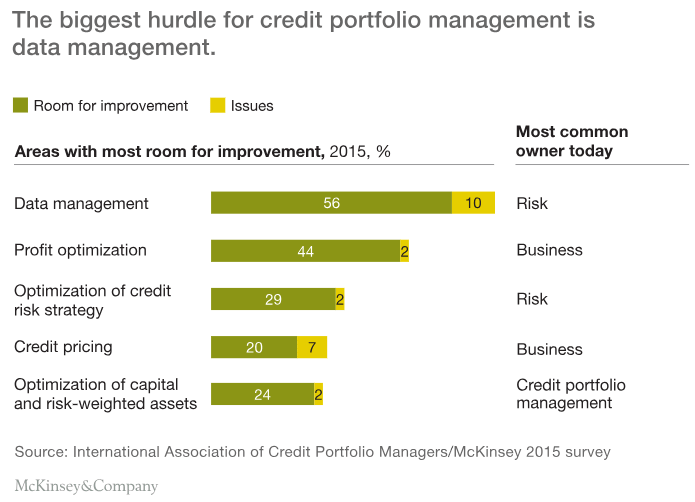
- Poor consumer segmentation. Operating across different markets, each with their own regulations, consumer preferences, and risk profiles, financial institutions require flexible solutions that can easily adapt to a range of scenarios.
- Cumbersome model updates. Large global banks have a wide range of model types that make it highly complicated and time-consuming to modify model parameters, revoke outdated models and implement new models.
- Lack of advanced analytics. In-depth data analysis allows institutions to extract maximum value from the information available in each market and design multiple strategies.
AI in credit underwriting, under the hood
AI techniques can help banks to overcome risk management challenges. That’s exactly why we developed GiniMachine – risk management with AI software that helps enterprises streamline middle office operations and back-end processes.

- Advanced analytics. Software based on advanced machine learning techniques which provides valuable insights into data sets and is perfect for exploratory analysis. GiniMachine is capable of pinpointing hidden dependencies between variables that may otherwise be impossible to discover. This solution can use unstructured and Big Data while handling imperfect and missing values.
- Credit risk modeling. Based on “supervised learning” algorithms, GiniMachine can make predictions on raw data without any preliminary analysis and data-preprocessing. With a prepared dataset, it only takes 2-10 minutes to build and validate a risk model. Thus, GiniMachine saves hundreds of hours of manual work for risk officers and data analysts.
- Consumer segmentation. GiniMachine can adapt to a variety of scenarios giving access to new potential consumer segments such as millennials, self-employed entrepreneurs, small business owners.
- Fraud & churn prediction. The software can detect suspicious transactions, loan applications and behavioural patterns. GiniMachine can find dependencies that are not obvious and assess fraud risk during the loan origination process. It can also identify the top drivers of churn, enabling in-session personalization.
- Real-time predictions. GiniMachine can generate predictions in milliseconds.
Bottom Line:
According to PwC, 82% of banks and investment managers plan to increase partnership with FinTechs over the next 3 to 5 years and expect an average ROI of 20% on their innovation projects.
Risk management demands more attention and stricter practices. If companies wish to achieve higher organizational agility, they need to embrace innovation and view FinTechs as key contributors to the bottom line.
Interested in AI scoring? Get in touch with the GiniMachine team.