The Importance of Predictive Model Monitoring and How to Do It
Predictive models play a crucial role in aiding businesses in making informed decisions based on data-driven insights. However, it is essential to recognize that these models can experience a decline in their predictive power over time because of data drift or concept drift.
In this article, we will explore why it is important to proactively monitor predictive models and provide guidance on how to effectively carry out this monitoring process.
Understanding Data Drift and Concept Drift
Data drift refers to the situation where the input data used for training the model undergoes meaningful changes, leading to a significant difference in the distribution of variables. For instance, if a business starts working with borrowers from a different region, new columns may be introduced to the dataset. Although the model may still perform well on data similar to the “old” dataset, its predictive accuracy may suffer when faced with new, unseen data.
On the other hand, concept drift occurs when the relationships between the model’s inputs and outputs change, rendering the patterns learned by the model no longer relevant. This can happen even if the structure of the applicant’s data remains the same. For example, during an economic crisis, the factors influencing loan approval may shift, making the previously effective model less accurate.
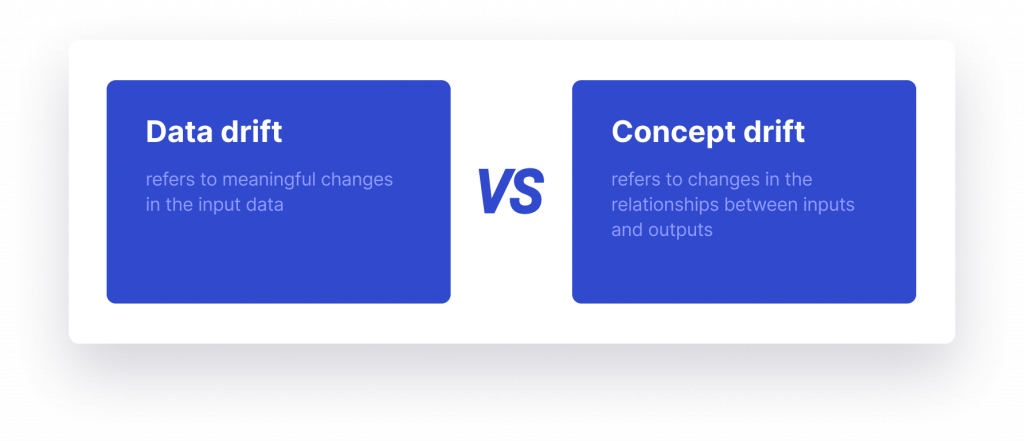
Why Model Monitoring Is So Important
1. Maintaining Accuracy
Regularly monitoring predictive models helps ensure their ongoing accuracy and reliability. By detecting and addressing data drift or concept drift early on, businesses can prevent the model’s predictions from becoming outdated or inaccurate, thus preserving the effectiveness of their decision-making process.
2. Adapting to Changing Conditions
Business environments are dynamic, and factors influencing predictions can change over time. Monitoring models allows businesses to adapt to these changes by updating and retraining models to capture new patterns or relationships, enabling them to make decisions based on up-to-date insights.
3. Mitigating Risks and Costs
An outdated or inaccurate predictive model can lead to costly errors or missed opportunities. With the regular and proactive model monitoring, businesses can mitigate the risks associated with relying on outdated predictions, avoid unnecessary costs, and maintain a competitive edge in their industry.
Principles of Model Monitoring
The power of your model hinges not just on its initial setup but on its continuous monitoring and improvement. To harness the full potential of your predictive models, we outline five essential principles for effective model monitoring. These guidelines are designed to optimize model performance and ensure that your predictive analytics software remains a reliable and powerful tool in your decision-making arsenal.
1. Track Performance Statistics and Make Improvements
Monitor the performance of the models and identify areas for improvement. This can be done by analyzing performance statistics and making adjustments to the model. Additionally, providing the model with new data can help improve its performance.
2. Increase the Quality and Quantity of Information
To enhance the model’s accuracy and effectiveness, increase the quality and quantity of information available for training and evaluation. How? Try to involve collecting more relevant data or improving data collection methods.
3. Remember Dates of Changes and Conduct Periodic Evaluations
Keep track of the dates when changes are made to the model and revisit its performance after a certain period. This approach is similar to A/B testing, allowing for comparison and assessment of the model’s performance over time.
4. Continuously Assess Effectiveness and Audit External Factors
Regularly evaluate the model’s effectiveness to identify any significant changes. If there are substantial deviations in the model’s performance, initiate an audit of external factors, including macro and microeconomic factors. This examination helps identify potential influences that may impact the model’s accuracy.
5. Review the Cutoff
Periodically review and reassess the knowledge cutoff point, which is the date until which the model has been trained. This ensures that the model incorporates the most up-to-date information and accounts for any changes that may have occurred since the cutoff.
How to Monitor Predictive Models with GiniMachine
Go to the Monitor section. Here, you’ll see the dashboard with graphics that provide insights into the model’s performance. GiniMachine offers a comprehensive monitoring solution that facilitates effective model monitoring and helps businesses stay on top of their predictive analytics.
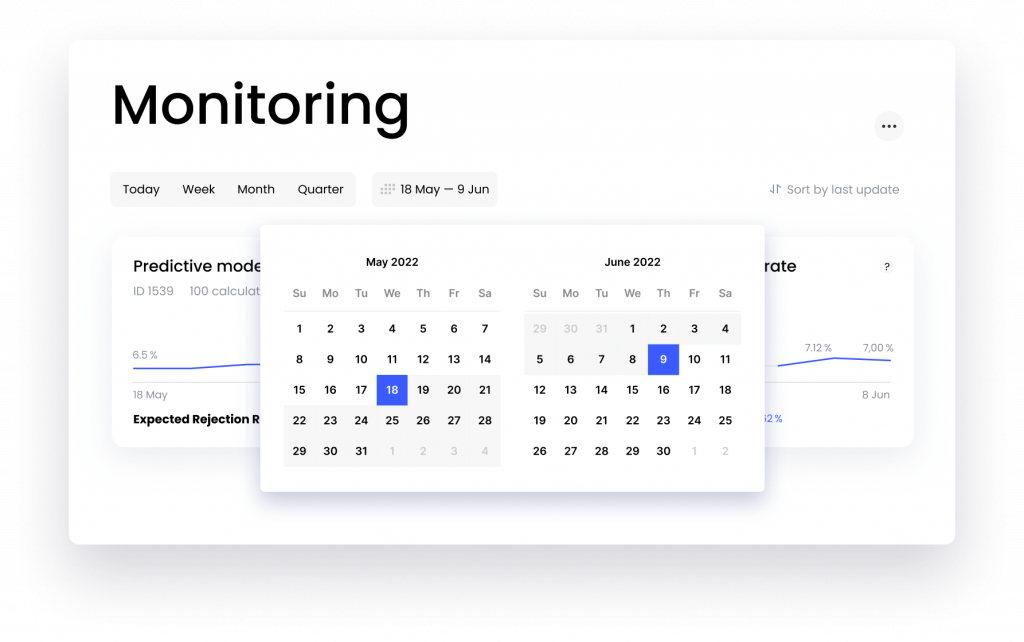
Choose the model for monitoring, and observe the evolving graphic on a daily basis.
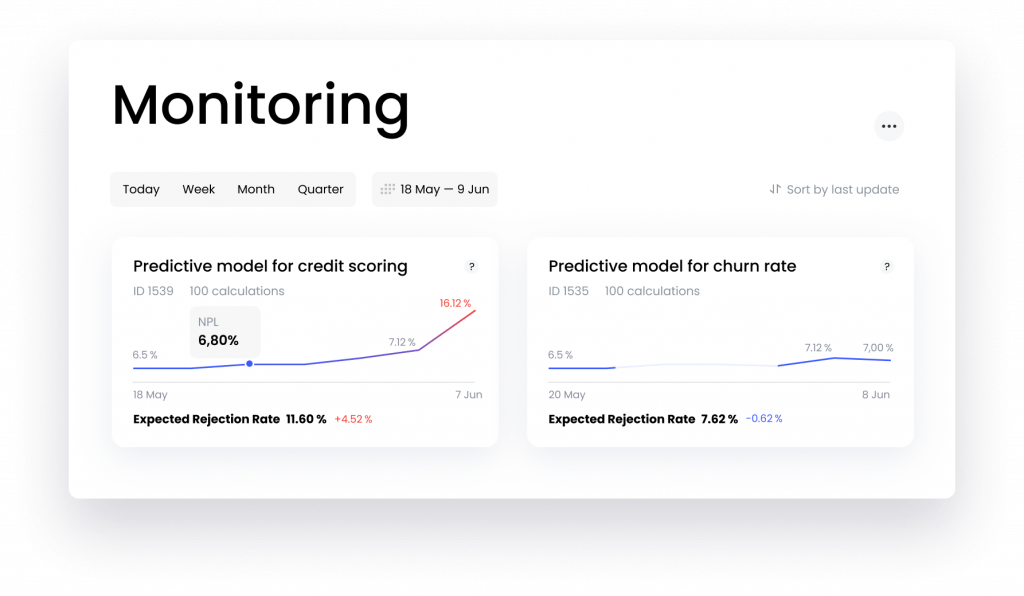
For each model, you’ll find a tab that provides a hint about the model’s performance, allowing you to make more informed decisions based on that data.
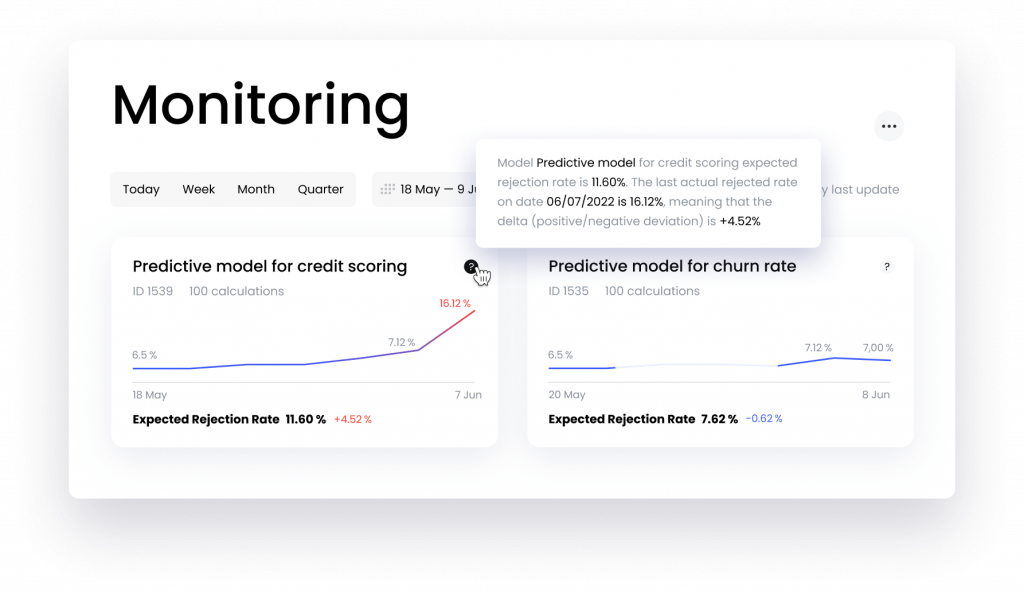
Wrapping Up
By implementing these principles and utilizing the right tools, businesses can ensure the ongoing accuracy and reliability of their predictive models. Proactive model monitoring enables them to adapt to changing conditions, mitigate risks, and make data-driven decisions with confidence.