How to Improve Your Proxy Business Metrics with GiniMachine
Working in banking and lending businesses involves managing vast amounts of data that guide your institution towards achieving its KPIs. However, what if there’s more to focus on beyond just KPIs?
This article delves into the intricate world of proxy metrics, essential tools that indirectly measure and infer the value of more elusive, direct metrics. As we navigate through the nuances of proxy metrics and their critical role in business strategy, we will explore how GiniMachine’s AI-powered capabilities can profoundly enhance decision-making processes, transforming data into actionable intelligence. Let’s get started.
But First… What Proxy Metrics Are About?
A proxy metric is an indirect measure used to estimate or infer the value of a target metric with which it is closely correlated. It acts as a stand-in for a more direct or primary metric that might be harder to measure. The change in a proxy metric can indicate the direction of change in the target metric. For example, Gross Domestic Product (GDP) per capita might be used as a proxy metric for the quality of life in a region. Proxy metrics are often derived from business data, like the number of loan approvals or rejections, and are utilized for making informed business decisions.
In banking and alternative lending businesses, proxy metrics are used to gauge performance, risk, and operational efficiency. Some common examples include:
1. Loan Approval Rates
This measures the percentage of loans approved and can indicate the effectiveness of lending criteria and market reach.
2. Non-Performing Loan (NPL) Rates
NPL rates give insight into the credit quality of the loan portfolio and the effectiveness of credit risk management.
3. Portfolio Size
The total size of the loan portfolio can act as a proxy for market share and business growth.
4. Average Loan Size
This metric can reflect the target market segment and risk appetite of the lender.
5. Customer Acquisition Cost (CAC)
CAC can be a proxy for marketing efficiency and long-term profitability.
6. Default Rates
The rate of loan defaults can indicate the effectiveness of underwriting processes and overall risk management.
7. Customer Satisfaction Scores
These scores can serve as a proxy for service quality and customer retention potential.
8. Cost of Risk (CoR)
This measures the cost incurred by the bank due to risks taken, serving as a proxy for overall risk management effectiveness.
Each of these proxy metrics can provide valuable insights into different aspects of the banking and alternative lending business, guiding strategic decisions and operational improvements.
The Difference Between Proxy Metrics and KPIs
It looks like proxy metrics are similar to KPIs in many ways. Both are used to measure performance and guide decision-making in businesses. However, there are subtle differences:
Proxy metrics are indirect measures used when direct measurement of a desired outcome is difficult. They are based on the assumption that they correlate strongly with the target metric.
KPIs are direct and specific measures used to evaluate the success of an organization or of a particular activity in which it engages. KPIs are often more closely aligned with strategic goals and objectives.
While proxy metrics can sometimes be KPIs, not all KPIs are proxy metrics. Proxy metrics serve more as a stand-in for another measurement, while KPIs directly assess performance against key business objectives.
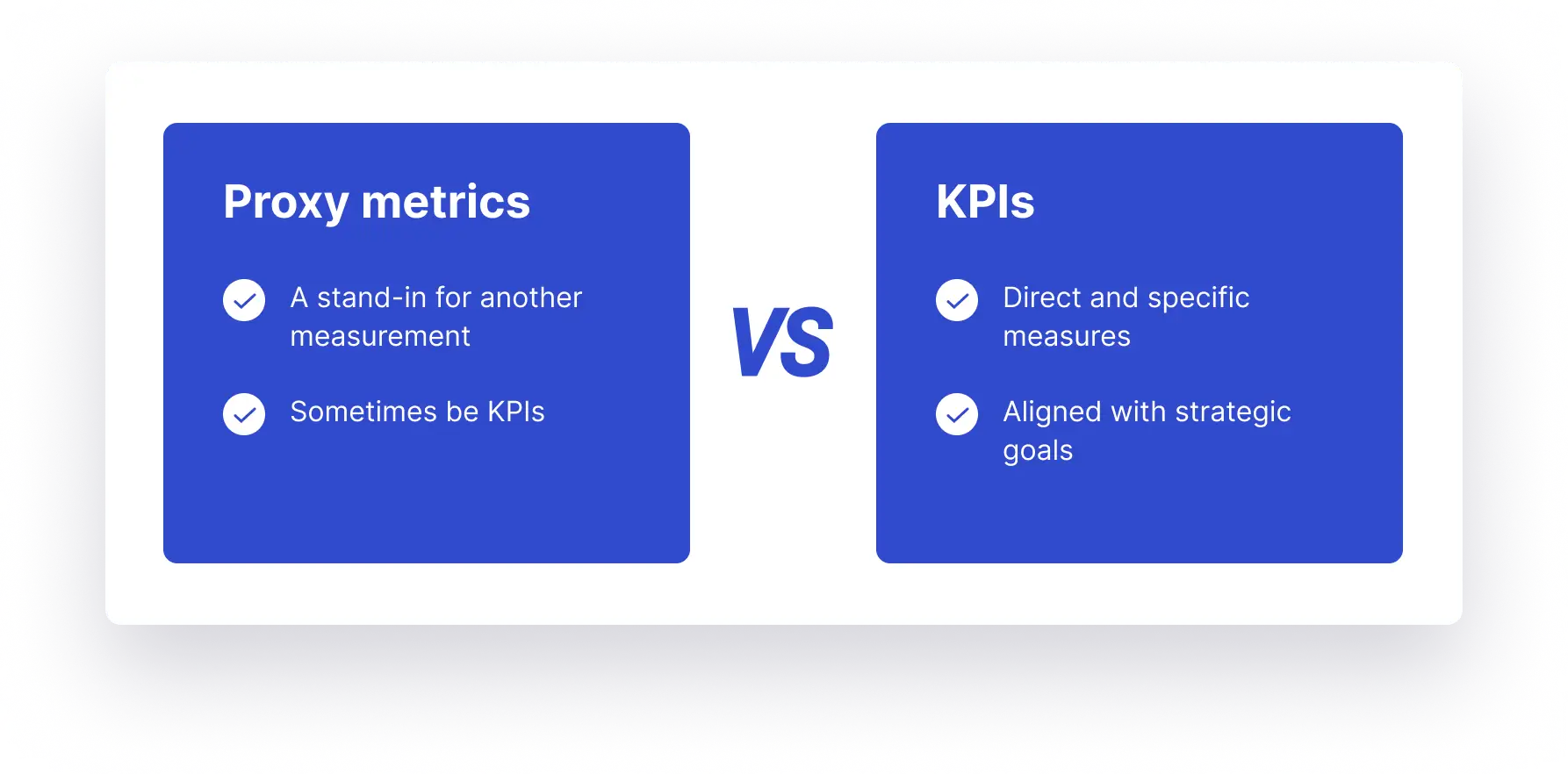
About GiniMachine
Our automated decision-making platform replaces outdated spreadsheets and static data visualizations with advanced AI algorithms. These algorithms can process terabytes of historical data, building, validating, and deploying predictive models in mere minutes. This speed and efficiency can significantly enhance your decision-making processes.
Transform Credit Scoring
For businesses focusing on credit scoring, GiniMachine offers a fresh perspective. It overcomes the limitations of traditional credit scoring methods by employing AI underwriting decision software. This approach not only maximizes profits but also effectively manages risks, even extending loans to thin-file borrowers.
Optimize Collection Scoring
GiniMachine excels in collection scoring by prioritizing debtors who are primed for quick payback. It recommends the most effective collection tools, be it calls, messages, or other methods, based on sophisticated scoring parameters. This results in a significant boost in productivity for collection businesses and an effective management of non-performing debts.
No-Code AI Predictive Modeling
One of the standout features of GiniMachine is its no-code AI predictive modeling. This allows a wide range of business types to utilize AI for balanced decision-making. The platform’s user-friendly interface and industry-specific features make it accessible to non-technical users, broadening its applicability across various sectors.
Building and Validating Models
GiniMachine can unlock precision in your predictive models using as few as 1000 raw records of past business decisions and their outcomes. It automatically identifies the most impactful factors leading to positive or negative results. Furthermore, it self-assesses its own accuracy and suitability for business forecasting, ensuring the reliability of the predictions.
The Role of Cut-Off Selection to Improve Proxy Metrics
A cut-off refers to a threshold value used to categorize predictions. It determines the point at which a prediction is classified as positive or negative. For instance, in loan approval processes, the cut-off would decide what score range is considered acceptable for approving loans.
The importance of the cut-off lies in its role in balancing the trade-offs between various outcomes like acceptance rates, default risks, and profitability. An appropriately set cut-off ensures that the predictive model aligns with the business’s strategic objectives, such as minimizing risk while maximizing loan approvals. It’s a key factor in fine-tuning decision-making processes to achieve optimal outcomes.
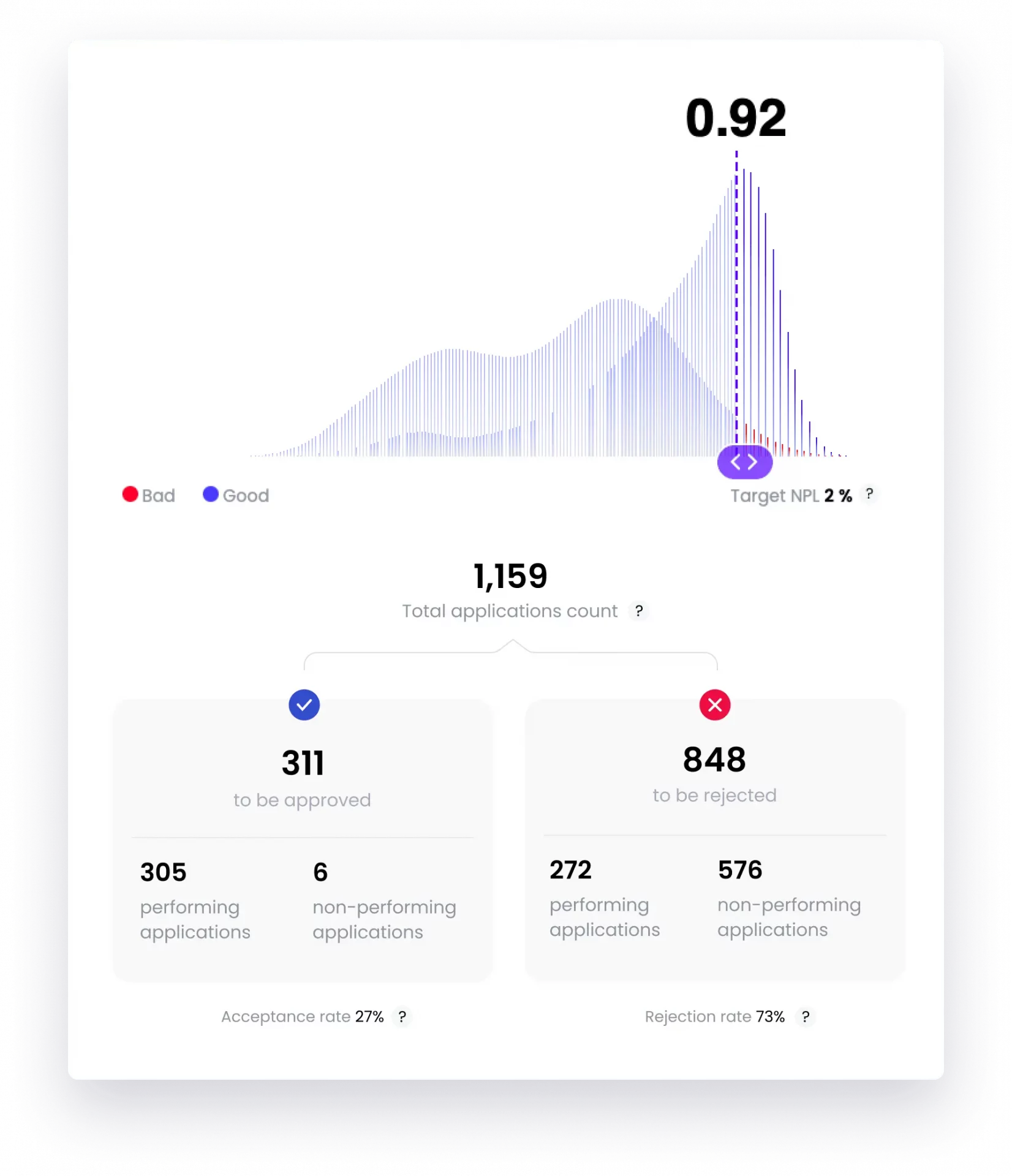
In the context of improving proxy metrics, managers can effectively select the cut-off value to enhance decision-making in banking and alternative lending. The cut-off value, crucial in determining the classification threshold for predictions, balances precision and recall.
By adjusting the cut-off value, they can monitor real-time impacts on metrics such as precision, recall, and revenue, thereby optimizing lending decisions, risk management, and profitability. This process enables managers to align their decisions with business goals, risk appetite, and regulatory requirements, directly impacting their proxy metrics such as loan approval rates, NPL rates, and overall portfolio quality.
Wrapping Up
Proxy metrics, acting as indirect measures, provide valuable insights into performance, risk, and operational efficiency. Tools like GiniMachine enhance the capability to accurately assess and improve these metrics. By utilizing its advanced AI algorithms for predictive analytics and strategic cut-off selection, businesses can effectively align their decision-making processes with their objectives, thereby optimizing key proxy metrics such as loan approval rates, NPL rates, and customer satisfaction scores. This leads to more informed business strategies and improved overall performance.