Choosing the Cut-Off Value
The cut-off value plays a crucial role in determining the classification threshold for the predictions. By selecting an appropriate cut-off, businesses can strike a balance between precision and recall, maximizing the tool’s effectiveness. It essentially defines the point at which a prediction is classified as positive or negative, or when a specific decision should be made based on the predictive scores.
Factors to Consider when Selecting the Cut-Off
- Profit forecast: At GiniMachine, you can specify the desired value for accurate predictions and the cost associated with mistakes. The platform will then provide you with the optimal cut-off value to maximize your profit.
- NPL rate analysis: Assess the impact of different cut-off values on the NPL rate. Determine how effectively the model can identify and classify loans that are likely to result in non-payment or default. A lower NPL rate indicates better risk management and loan portfolio quality.
- Acceptance rate: Examine the acceptance rate for different cut-off values. Consider the trade-offs between approving more loans (higher acceptance rate) and potential risks associated with increased defaults or non-payments. Striking the right balance is crucial for optimizing lending decisions and maintaining a healthy loan portfolio.
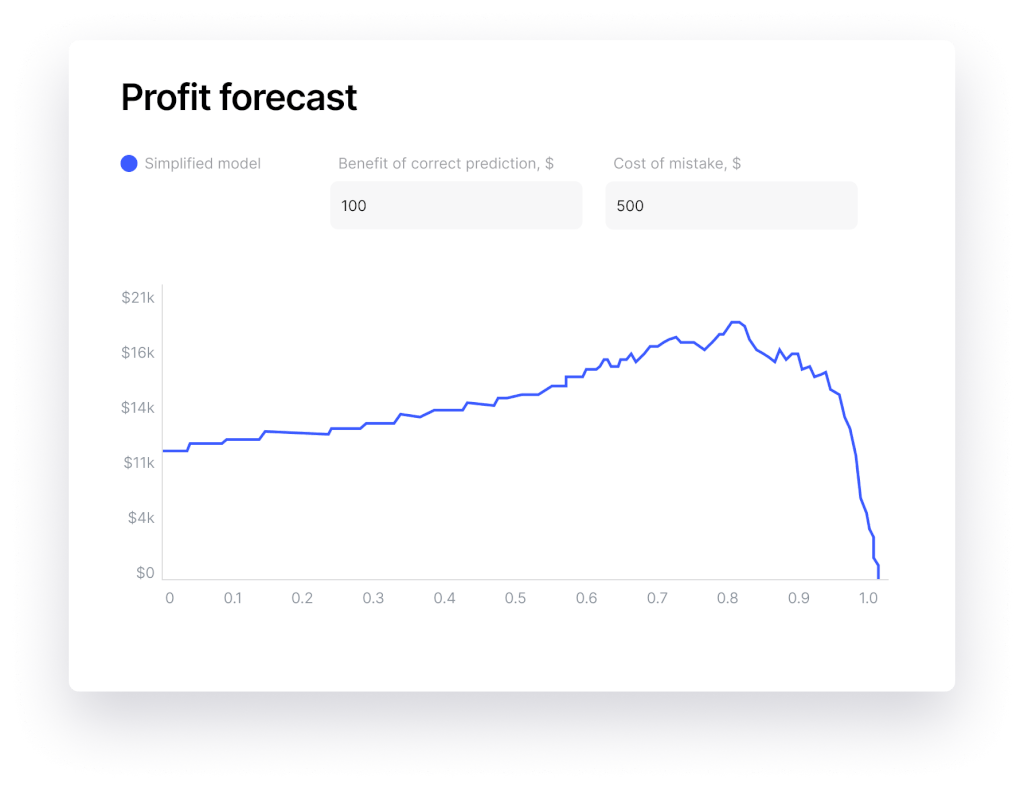
Now let’s examine how to actually set up the cut-off with GiniMachine.
3 Steps to Set Up Cut-Off with GiniMachine
- Move the Cut-off Value Slider for Balance. The first step in selecting the cut-off value is to aim for a balanced state. This means finding a threshold that aligns with your business strategy, priorities, and risk tolerance. By moving the cut-off value slider, users can visually observe how the distribution of predictions and economic effects change in real-time.
- Monitor Predictions and Economic Effects. As you adjust the cut-off value, it is essential to closely monitor the predictions and economic effects in real-time. GiniMachine provides a dynamic environment where you can observe the impact of changing the cut-off value on various metrics, such as precision, recall, accuracy, and revenue. By examining these metrics and their fluctuations, you can gain insights into the trade-offs between different cut-off values and their corresponding outcomes.
- Build Powerful Scoring Models. To effectively explore the best cut-off value, it is recommended to build multiple powerful scoring models (see how to start scoring with GiniMachine). GiniMachine allows users to create and evaluate different models using various algorithms and configurations. By generating multiple models, you can compare their performance at different cut–off values. This approach helps in identifying the optimal cut-off value that maximizes the desired outcomes while considering the associated risks.
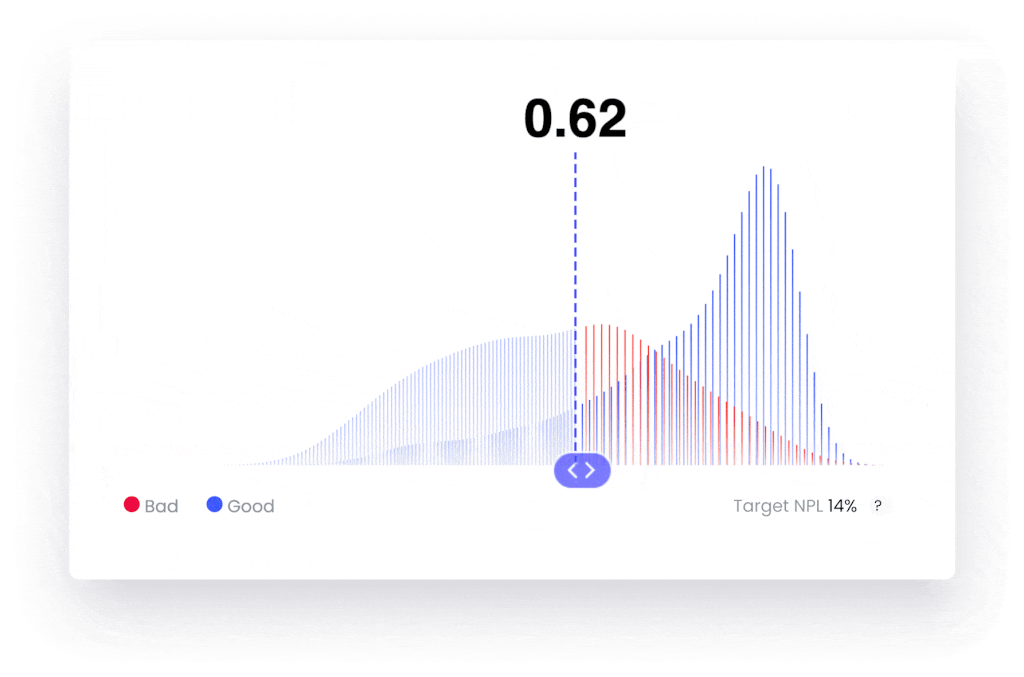
How to Interpret the Cut-off Selection
The choice of the cut-off value directly affects the number of applications that are classified as positive or negative. A higher cut-off value means that more applications will be rejected, leading to a safer strategy. This approach minimizes the risk of false positives, ensuring that only highly confident predictions are accepted. On the other hand, a lower cut-off value is a good choice when you want to play it safe. It increases the acceptance rate, reducing the chances of rejecting potentially positive cases. However, this approach may come with a higher risk of false positives.
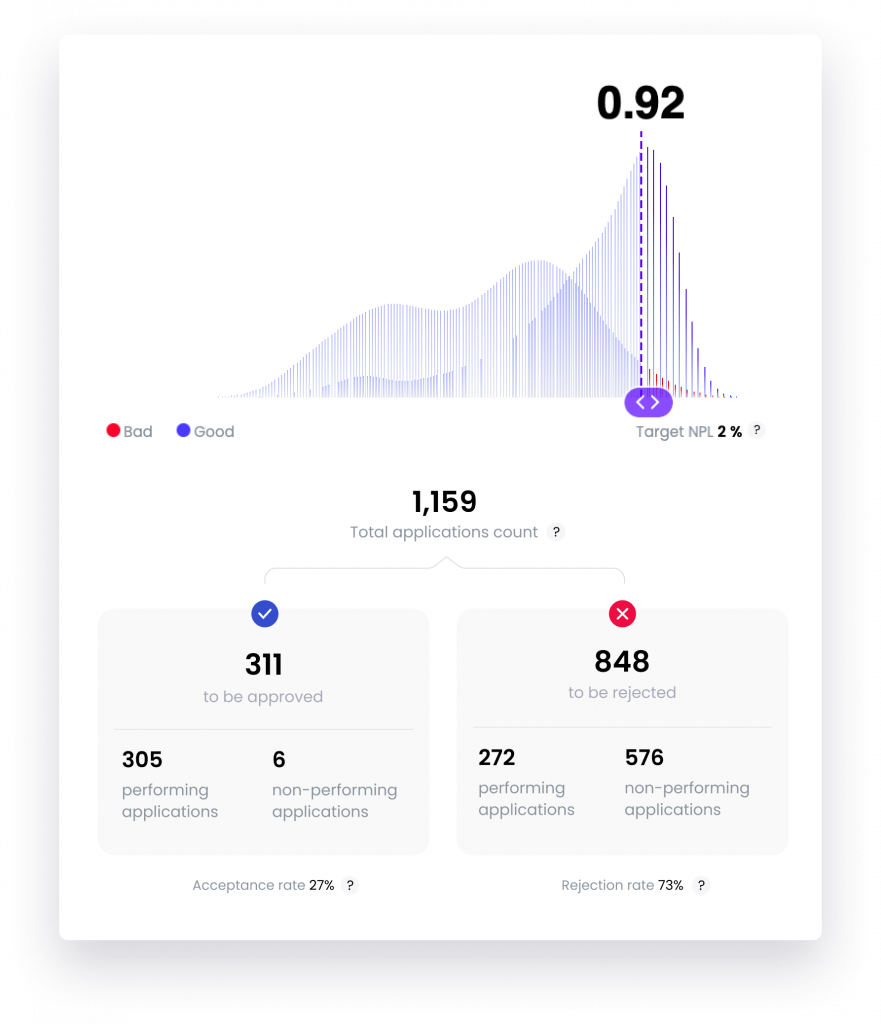
When selecting the cut-off value, it’s crucial to consider the trade-off between the benefits of accepting more positive cases and the associated risks of false positives. A higher cut-off value may result in missed revenue opportunities from potential positive cases, while a lower cut-off value may increase the risk of accepting unreliable or fraudulent cases. Finding the right balance is essential to strike a compromise that aligns with your business goals, risk appetite, and regulatory requirements.
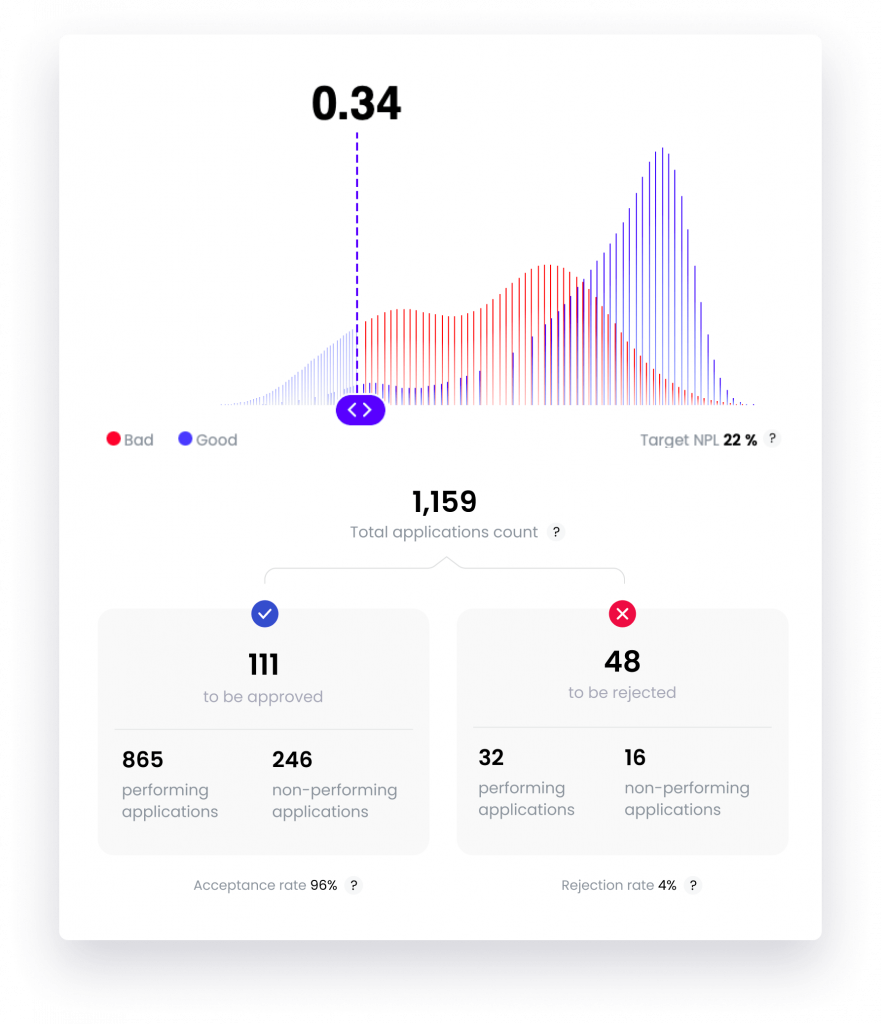