Loan Prediction Using Machine Learning: How Much Does It Really Cost?
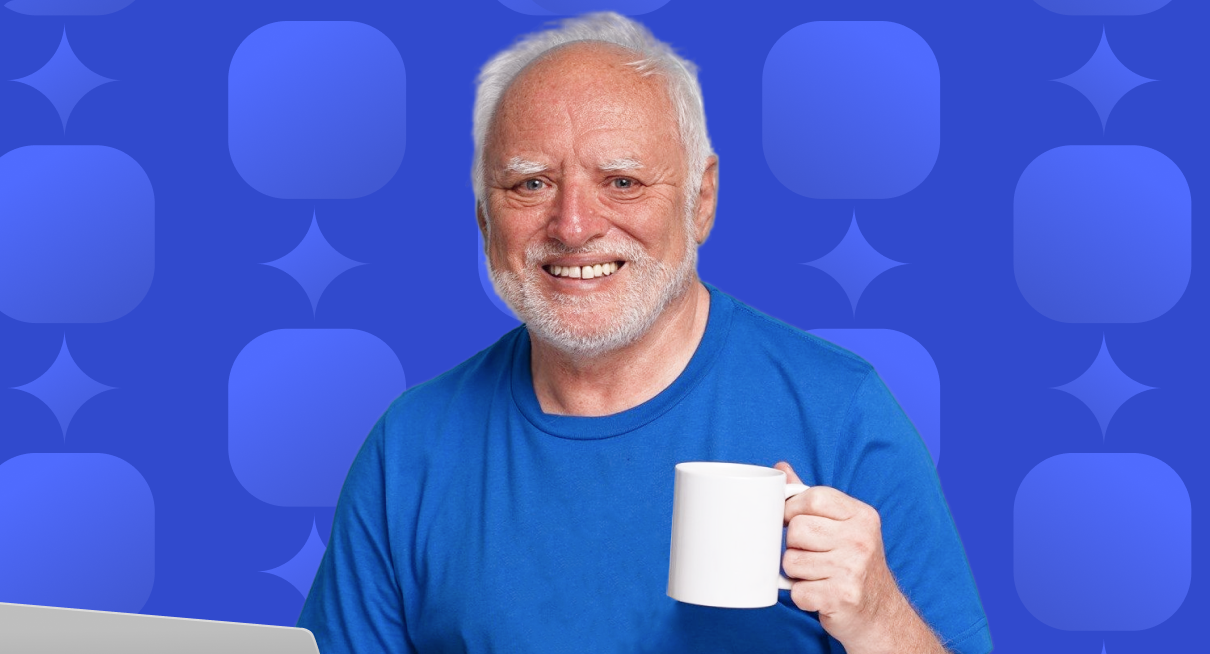
Loan prediction using machine learning offers businesses the power to tailor their offerings and lower their risk at the same time. So, then, why are many choosing to stick to older assessment credit models instead of a smarter future? The answer is cost, or at least, the perception of the expense. For businesses, it’s a struggle to qualify and quantify the level of improvement and its price when adopting a new technology, such as intelligent bank underwriting software. And this is even despite the evidence that proves it as a solid solution.
The Historic Underwriting Model
In the past, the credit score, issued by a set list of providers, was the gold standard for assessing a person or company’s creditworthiness. This credit scoring algorithm was somewhat efficient in assessing and identifying risks by examining key identification factors in a person/business’ financial history. However, it failed to take into account individual circumstances, such as a person’s lack of credit history or extremely old transactions, leading to a poor score. While credit scores have been a decent indicator of an individual or company’s credit health in the past, a lot has changed since then, and new, viable solutions are taking over.
New technologies, such as GiniMachine, are specifically developed to provide machine learning underwriting tools and credit checks using intelligent technology. These solutions are often automated, leading to efficiency increases and smarter, allowing for personalized lending risk assessments and more intelligent loan decisions. It seems like a no-brainer that almost every lending provider would want to onboard them immediately. However, this is not the case.
AI applications in finance are often costly investments. This leads financial providers away from onboarding them and favoring cheaper, less effective solutions due to a lack of clarity of the long-term benefit for their business.
How Much Does Loan Prediction Using Machine Learning Actually Cost?
Cost is never just a number on a page. Instead, the cost is all value something brings to a business or individual, and whether or not this value exceeds the numerical figure given to acquire that. That’s why, when examining how much ML credit underwriting models cost, we break it down into the quantitative (numbers) and the qualitative (business value).
Quantitative
First, let’s take a look at those all-important numbers and how to establish the cost of adopting AI-based credit underwriting models in your business. Here are the elements you should take into account before you decide to make a purchase.
Calculating the TCO (total cost of ownership)—this metric measures the total amount, in terms of finances, that it will cost to own your loan prediction technology. Instead of focusing solely on the acquisition costs, TCO looks at how much this particular investment will cost your business over time to allow you to better estimate the costs. It is calculated via a formula:
- Initial cost + maintenance costs – remaining costs = total cost of ownership
In this case, initial costs include the price/subscription you will pay for the technology. Maintenance costs refer to upkeep and updates to ensure the loan prediction technology runs smoothly. Meanwhile, remaining costs look at the tech’s long-term value and how much it will be worth to your business over time.
Establishing the number of good loans missed—in addition to calculating how much you’ll spend on machine learning in loans, you’ll need to take account of the amount you could potentially generate for your business.
When taking account of these metrics, it’s vital to examine the loans you could potentially issue if your loan model allowed for it. For example, could you issue to those without a credit history? Or look at servicing clients who defaulted 20 years ago but have made every payment since? Or perhaps look at young lenders who would otherwise be deemed risky?
In establishing the profit of being able to work with these groups, you could potentially access a new line of revenue at a relatively low risk.
Evaluating the amount saved—At the same time, it makes sense to seek out the borrowers that are costing you more. Within your current creditworthiness system, you may have noticed some borrowers tend to default more than others despite overall ‘good’ credit scores. And if you’ve ever wondered why you already see the benefit of establishing smart credit checks.
One you calculate these values; you’ll have a tangible figure of how much onboarding a new loan prediction technology could save you and its potential impact on your current credit scoring model.
Qualitative
However, as you’ll know, it’s not just the monetary values that play a role in deciding to onboard a new system. It’s also the qualitative value that it’s set to deliver. That’s why before deciding to invest, it’s essential to take a look at these factors:
Time saved—how much time could you potentially save your team when dealing with loan applications. By reducing the time spent evaluating credit scores using machine learning technology, you build your efficiency and can dedicate that time to increasing the number of loan applications processed and clients serviced.
Accuracy—one of the primary benefits of using machine learning for credit scoring is its accuracy. Unlike human manual processing, ML-based models are automated and less likely to make mistakes. This means that loan processing becomes not only faster but more accurate, too, cutting costs on the whole.
Ease of service—in the last decade, the lending industry has boomed with new competitors entering the market alongside traditional providers. Given a wider range of financial providers, borrowers are now choosing where to lend from. By creating ease-of-service and seamless onboarding, you increase your market competitiveness and make it more likely that clients will choose your service provider.
Personalization—this has long been growing as a trend, and no more so in the advancements of digital service use in the wake of the COVID-19 pandemic. Clients expect personalization and ML-based models are able to deliver. Not only can you deliver more tailored loans, but you can also establish an individual’s risk more closely than in previous years.
Growth potential—how much could your business potentially grow if more efficient methods of credit scoring were onboarded? The growth potential in terms of clients serviced or the variety of services offered helps to establish the value of intelligent bank underwriting software for your business.
Credit Underwriting Models Compared
Onboarding any new technology is going to come at a cost to your enterprise. But before you consider adding new machine learning underwriting tools, its vital to establish how they perform in comparison to traditional methods.
Traditional credit scoring for underwriting | Machine learning (ML) for credit underwriting |
---|---|
Limited to established models (FICO, etc.) and metrics Often fail to take account of the credit invisible (those who have never had credit before). Good for general checks and includes: сredit history, credit utilization, length of credit history, types of credit, when someone took credit. | Takes account of the client as a whole Looks at defined factors, such as but not limited to spending and subscriptions, lending history, risky behavior, etc. Can also take account of traditional credit scoring. |
Manual checks Client data is manually sent off a review by an established provider. | Automated processes Once your client data is entered into the system it is automatically processes and returned with a risk assessment. |
Often safer (not always better) Traditional credit checks are designed to protect against risk for the lending provider. However, many use stringent, established processes that don’t allow for actual risk, vs. perceived risk. | Potential to offer risk-based pricing Machine learning models for credit scoring take into account the actual risk an individual poses when taking credit and allows lending providers to offer risk-based rates. I.e., High rates for high-risk borrowers, lower rates for low-risk borrowers. |
Checks don’t change much over time Establishing credit scoring methods haven’t changed much since they were put into practice. This means that some of the metrics they use don’t necessarily correlate with modern market demand. | Take account of the latest data ML-based models work based on the metrics your business decides are important for lending. This can take account of specific risk areas deemed essential and the latest lending data. Such practices can be adjusted to suit changing needs, such as those posed by the economic effects of coronavirus lockdowns. |
Long-practiced Credit scoring via traditional models has been long-practiced and proven to be effective. It does genuinely lower the risk for a provider. | Faster New technologies blend speed and efficiency to deliver decisions on lending quicker than ever before. That said, due to their novel character, the technology many need tuned to your particular business before you see the full effects. |
Ready to Add Machine Learning in Finance to Your Business?
The lending market is becoming increasingly competitive in terms of the variety of offerings and providers. For lending providers, this means it’s essential to review the current services provided and establish if new processes are required to keep up with market demand. Lenders considering purchasing intelligent bank underwriting software such as machine learning tools should take account of the various value factors that go into such technology and establish if these are a good fit for their business. By utilizing the latest AI technology, they could be set to gain via the creation of custom lending models that take account of the client as a whole, not just their presumed risk, leading to increased revenue potential over time.
Interested in machine learning software for scoring? Contact us for a free live demo tour.