Credit Card Fraud Prediction: How to Beat the Odds & Get Results
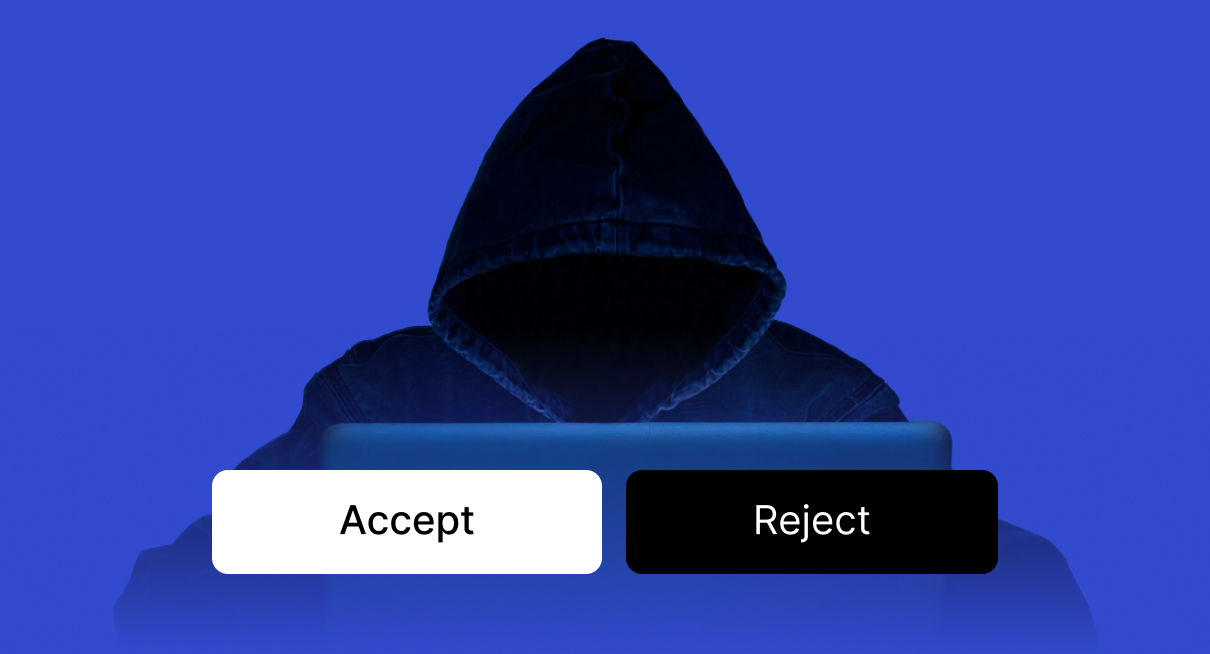
Credit card fraud statistics are falling, but what should be a win for the industry may be the calm before the storm. A recent report suggests that another rise in fraud is on the horizon. Estimates in credit card fraud prediction show an expected ~$34 billion fraud level in 2022, rising to ~$49 billion by 2030, with the majority occurring in the US market.
The sophistication level of attacks, coupled with increased usage of online technology makes it harder for card providers to get on top of fraud. This has left many seeking smarter tools, such as fraud app detection software equipped with artificial intelligence (AI) and machine learning (ML) to help tackle credit card fraud.
What is Credit Card Fraud?
Credit card fraud covers a number of activities that are intended to acquire money or other using a credit or debit card in a fraudulent way. Although the rise of internet technologies has made credit card fraud more widespread, for a long time, credit card fraud statistics have shown that fraudsters are swift to adapt to industry changes and take on new, innovative methods to achieve their objectives.
For both companies and businesses, credit card fraud is a massive problem causing undue stress, loss of funds and even long-term life disruption, for example in the case of identity fraud.
Although the methods are constantly changing, the types of credit card fraud generally fit into five categories:
Lost or stolen card fraud—a common type of credit card fraud. This is where the fraudster steals a card or uses a lost card. Sometimes they are able to do so by stealing cards from someone’s post or intercepting delivery.
Card-not-present fraud (CNP)—one of the more difficult types of fraud to prevent. Card-not-present fraud is what happens when a fraudster uses a card’s details to make payments by phone or online. As no physical card is needed, this makes it much easier for the fraudster to deceive the merchant.
Card application fraud—by using real details of a person, a fraudster is able to apply for and acquire a credit card in another person’s name. This type of fraud can have long-term consequences for the individual whose identity was stolen as it can affect their credit score.
Card-never-arrived fraud—this is what happens when a genuine client orders a card, however, the physical card never arrives at their address as it is intercepted or stolen along the way.
Card skimming and counterfeit cards—skimming is a practice used by fraudsters to copy the details stored on a card’s magnetic stripe when it is used at an ATM or merchant’s terminal. This allows them to acquire the information they need to undertake other types of fraud.
Credit Card Fraud Statistics to Take Note of Today
- The US market accounts for 35.8% of worldwide credit card fraud. Meanwhile, the remaining ~194 countries account for 64.2%.
- Despite this, the US accounts for only 22.4% of overall card volume, indicating a reasonably high fraud incidence rate.
- By 2030, credit card fraud statistics are expected to show a collective loss of $408.5 billion globally.
- In 2020, the Federal Trade Commission, credit card fraud is the 2nd most common type of identity fraud, according to reports.
- Nevada, Florida, Georgia, Maryland, and Delaware where the states that reported the highest levels of fraud per capita in the US.
- Fraud adds up. Despite estimated overall losses to fraud of $149 million (Federal Trade Commission), the median sum in these amounts was $311 and there were 526,007 reports of fraud between $1 and $100.
- Credit card fraud during COVID soared. Why? Due to an increase in online shopping activity, fraudsters had more opportunities to commit fraud than ever before.
How Can the Finance Industry Combat Credit Card Fraud?
Traditionally, combatting identity fraud relies on detecting fraudulent transactions and halting them in their tracks using a pre-determined set of ‘rules’ programmed into a system to highlight worrying transactions.
This is known as rules-based fraud detection.
For example, you may have run into this with a transaction being declined when you’re on holiday if you didn’t inform your bank you’d be traveling. Although rules-based fraud detection has been effective, advances on the side of fraudsters have meant that this type of fraud detection is no longer able to keep up. Instead, companies are increasingly turning to credit card fraud detection using machine learning techniques and artificial intelligence technology.
Benefits of ML and AI for Fraud Detection
Machine learning and artificial intelligence are technologies that allow a system to ‘learn’ as it works. If we take the original ‘rule-based’ approach, it’s limited in the fact that it doesn’t allow any flexibility.
Meanwhile, credit card fraud detection using machine learning techniques builds on this concept to analyze data and learn from it to develop more accuracy in fraud detection, fewer issues with genuine transactions (the holiday scenario) and requires less manual work for fraud detection agents, allowing them to focus on more serious cases. At the same time, AI for fraud detection allows the technology to evolve and develop to meet emerging fraud trends. Let’s take a look at the benefits.
- Speed of detection. Fraud is never ‘expected’ that’s why it’s essential that the detection system is swift at finding it. Machine learning in credit card industry for fraud detection updates in real-time to adapt to real market threats.
- Manages mass amounts of data. With traditional systems, fraud detection can be a laborious task as agents may have to wade through data manually with discover discrepancies. Credit card fraud detection using machine learning and AI is able to analyze mass amounts of data quickly so that inconsistencies can be found quicker or connections between various transactions. For example, this may be helpful in detecting those small ticket fraud items.
- Adapts to market changes. Unlike traditional systems that require rewriting the rules to detect fraud. Credit card fraud detection using artificial intelligence and machine learning monitors current results and trends, learning from them to become better at catching fraudulent activity.
What is GiniMachine and How Can it Help Credit Card Fraud Prediction?
GiniMachine is an ML-as-a-service platform that helps companies make smart predictions. The technology can analyze risk and data in order to detect fraud or other unusual activity. When it comes to credit card fraud prediction, adding GiniMachine to your fraud app detection software or web tool gives companies the power to build high-quality data-based models that can detect fraud, while at the same time analyzing reams of data to detect issues almost before they happen.
As a web-based application, GiniMachine utilizes Java and Python technology to work which data—ranging from socio-demographics, information about transactions, social networks and more. It works by:
- Managing data utilizing data pre-processing and preliminary analysis.
- Building and training models. GiniMachine does this by dividing training and test records to build a workable model.
- Validating models using detailed reports, insights, and diagrams. It is able to calculate an Index, provide recommendations, give important attributes and more.
- Deploying the technology allows GiniMachine to take action. The fully autonomous model is equipped to make real-life predictions for fraud prediction.
Next Steps for Credit Card Fraud Detection
Although onboarding smart AI and ML is often the most logical step, it’s not one to be taken lightly. Instead, such technology upgrades often require a strategic approach that will allow your company to maximize the benefits while minimizing the costs. Before setting out on the journey to better fraud detection, get in touch to find out how GiniMachine can help.
Interested in GiniMachine? Get in touch with the team to know more.